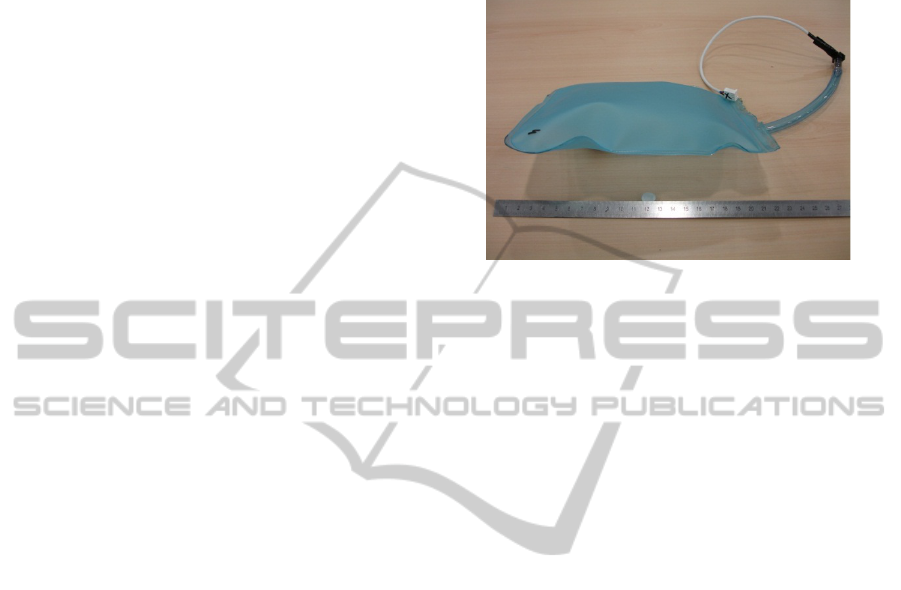
Tan et al (2001) described the use of a chair as a
haptic interface for human-computer interactions.
This work used two Tekscan® sensor sheets, with
42-by-48 sensing units to monitor the pressure
distribution in both the seat pad and the backrest.
The use of pattern recognition technics to develop a
static posture classification algorithm, such as
Principal Component Analyses (PCA), achieved an
overall classification accuracy of 96% and 79% for
familiar and unfamiliar users, respectively.
Other researchers used the same sensing system
configuration to test other classification algorithms.
Mota and Picard (2003) used Neural Networks to
classify 9 static postures in real time, achieving an
overall accuracy of 87.6%. In addition, Hidden
Markov Models were used to associate seated
postures with affective states. The algorithm
achieved an overall performance of 82.3% with
postures sequences coming from known subjects and
76.5% from unknown subjects. Zhu et al. (2003)
tested several classification algorithms to find which
one suits best static posture classification. Between
k-Nearest Neighbor, PCA, Linear Discriminant
Analysis and Sliced Inverse Regression (SIR), the
authors found PCA and SIR comparable in
performance and both outperformed the other
methods tested.
As suggested by Tan et al (1997, pp.57) “a low-
cost and low-resolution pressure sensing system will
then be developed to facilitate the widespread use of
smart chairs”. Mutlu et al (2007) adopted a near
optimal sensor placement approach to drastically
reduce the number of pressure sensors used. The
algorithm down-sampled the sensor data from the
high resolution sensor sheets used in previous
researches (Tan et al 2001; Mota and Picard 2003;
Zhu et al 2003) and determined the near optimal
placement of 19 one-and-a-half-inch-square FSR
(Force Sensitive Resistors) sensors. The system
achieved 78% accuracy with 19 sensors, and 87%
using data from 31 sensors.
More recently, Zheng and Morrell (2010)
developed a system with only 7 FSR and 6
vibrotactile actuators, specifically designed to
posture guidance through haptic feedback. With a
classification algorithm based on the mean squared
error between the pressure measurements and the
reference pressure for each static posture, an overall
accuracy of 86.4% was achieved when
distinguishing among 10 postures. This study has
also successfully shown the effectiveness of haptic
feedback for coaching motor behavior in the form of
seated posture.
3 CHAIR DEVELOPMENT
3.1 Pressure Cells
Figure 1: An inflated pressure cell with a gauge pressure
sensor attached.
The objectives of this project required an interface
that could measure the applied pressure and also
change the chair´s conformation. Taking in
consideration a low cost and commercial available
solution, we developed the pressure cells. This
pressure cells can sense pressure and serve as
actuators, by inflation and deflation, for posture
guidance and correction.
As seen in figure 1, a pressure cell is composed
of a large sealed thermoplastic polyurethane pocket
with 20x19cm of dimensions with a rubber pipe
attached. On the other end of the rubber pipe there is
a piezoelectric gauge pressure sensor to measure the
internal pressure of the cell. All cells have an equal
residual air volume for a baseline pressure
measurement. The gauge pressure sensors used were
the Honeywell 24PC series rated to 15PSI. In order
to compensate the inherent linear differences and
offsets between the sensors, calibration curves were
taken and corrected in software data processing, so
the sensors better match themselves.
3.2 Cells Placement
The use of a low resolution sensor matrix requires a
strategically sensor placement in order to achieve
good performance results. Two main strategies were
identified on previous literature. A pure
mathematical and statistical approach (Mutlu et al.,
2007) and an anatomical approach (Zheng and
Morrell, 2010) which considered the ischial
tuberosities, the thigh region behind the knee, the
lumbar region of the spine and the shoulder blades
as the most important and distinguishable areas of
the body for detecting postures.
BIODEVICES 2012 - International Conference on Biomedical Electronics and Devices
334