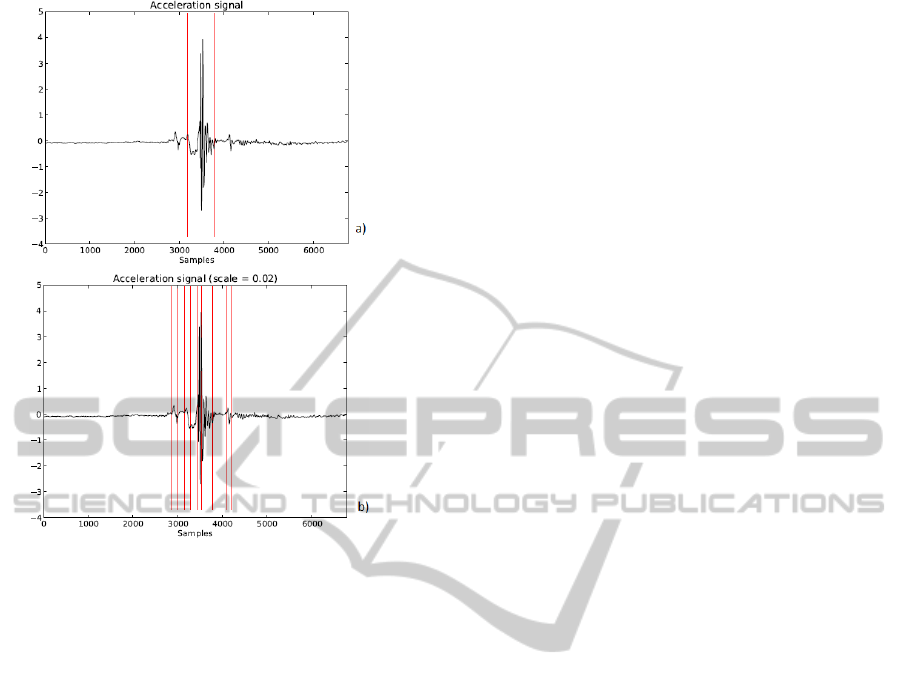
Figure 3: Acceleration signal results considering: a) default
scale factor and b) a scale factor of 0.02.
acceleration instability) and the landing phase, where
the instability increases significantly for a short time
period. The time spent recovering the knee stability
can also be estimated by accessing the posterior sig-
nal segments. When applied to EMG signals, the de-
fault mode events detection allowed a clear distinction
between activation and rest phases with an acute on-
set and offset detection. Being able to detect multiple
events and requiring no EMG specific pre-processing
steps, this algorithm presents advantages when com-
pared with the standard onset detection techniques
(Staude et al., 2001).
5 CONCLUSIONS AND FUTURE
WORK
The proposed algorithm performs an efficient events
detection within a signal. Its versatile design allows
the application in different signals, without previous
knowledge on their statistical characteristics and the
adjustment of a scale factor to achieve different detail
levels in specific applications. The added accuracy
and objectivity of this algorithm when compared with
the standard visual inspection also represents an ad-
vance in events detection from biosignals analysis.
In future work there is the intention of apply-
ing the algorithm to a wider range of biosignals and
evaluate its performance when compared with signal
specific processing techniques. Its integration into a
real-time processing tool is already under develop-
ment. Preliminary results point out the application’s
ability to detect the events in real-time, without a sig-
nificant loss of accuracy.
ACKNOWLEDGEMENTS
This work was partially supported by National
Strategic Reference Framework (NSRF-QREN) un-
der projects ”LUL” and ”Affective Mouse”, and Se-
venth Framework Programme (FP7) program under
project ICT4Depression, whose support the authors
gratefully acknowledge.
REFERENCES
Bonato, P., D’Alessio, T., and Knaflitz, M. (1998). A sta-
tistical method for the measurement of muscle activa-
tion intervals from surface myoelectric signal during
gait. Biomedical Engineering, IEEE Transactions on,
45(3):287–299.
Ciaccio, E., Dunn, S., and Akay, M. (1993). Biosignal
pattern recognition and interpretation systems. En-
gineering in Medicine and Biology Magazine, IEEE,
12(3):89–95.
Clifford, G. (2006). A novel framework for signal represen-
tation and source separation: Applications to filtering
and segmentation of biosignals. Journal of Biological
Systems, 14(2):169–184.
Hodges, P. and Bui, B. (1996). A comparison of
computer-based methods for the determination of
onset of muscle contraction using electromyogra-
phy. Electroencephalography and Clinical Neu-
rophysiology/Electromyography and Motor Control,
101(6):511–519.
Marques, C. J., Gamboa, H., Lampe, F., Barreiros, J., and
Cabri, J. (2011). Muscle activation thresholds before
and after total knee arthoplasty - protocol of a ran-
domized comparison of minimally invasive vs. stan-
dard approach. In Proceedings of the International
Conference on Bio-inspired Systems and Signal Pro-
cessing.
PLUX (2007). PLUX - Wireless Biosignals, S.A. [online]
Available at: http://plux.info/ [Accessed 5 September
2011].
Sankur, B., G
¨
uler, E. C., and Kahya, Y. (1996). Multireso-
lution biological transient extraction applied to respi-
ratory crackles. Computers in biology and medicine,
26(1):25–39.
Staude, G., Flachenecker, C., Daumer, M., and Wolf,
W. (2001). Onset detection in surface electromyo-
graphic signals: a systematic comparison of meth-
ods. EURASIP Journal on Applied Signal Processing,
2001(1):67–81.
BIOSIGNALS 2012 - International Conference on Bio-inspired Systems and Signal Processing
388