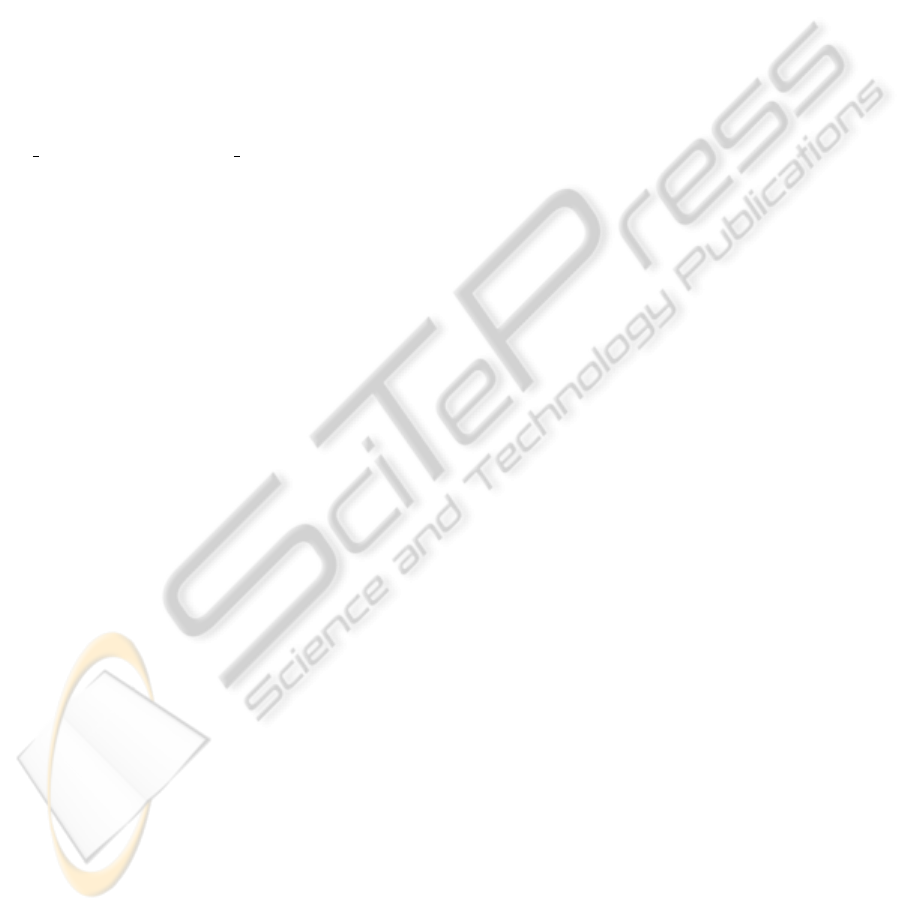
behavior. However, NB has a higher average rate with
84.5% compared to HO with 78.6% as shown in ta-
ble 5. Apparently, NB is more suited to deal with
small amounts of training data for certain user behav-
iors in our scenario: Due to the conditional indepen-
dence assumption in the NB approach, the probabil-
ities for each observation variable given the user be-
havior can be calculated independently of the other
observation variables. This leads to a more accurate
prediction of the underlying probabilities and a higher
classification rate compared to the HO method where
the observation variables are subsumed in a single ob-
servation. As shown in table 5, the NB produces ex-
cellent classification results for single user behaviors
showing a huge difference in length according to ta-
ble 4. The classification rates range from 81.7% for
brush teeth to 95.7% for rinse mouth. Only nothing
has a decreased rate of 50.4%.
Our results show that our hierarchical classifica-
tion framework based on the results of IU analysis is
well suited to approach the recognition problem in our
scenario. Our framework can deal well with the spe-
cific requirements of small amounts of training data
for certain behaviors and arbitrary behavior lengths.
7 CONCLUSIONS
In this paper, we focus on the challenging problem
of user behavior recognition in a real-world scenario.
We use a structured approach to develop a recognition
framework for an automatic prompting system assist-
ing persons with cognitive disabilities in the everyday
task of brushing teeth. We analyze the task using IU
analysis. We identify user behaviors which are im-
portant to complete the task successfully as well as
environmental configurations of objects involved in
the task. User behaviors are classified based on envi-
ronmental configurations using a Bayesian Network
(BN) in a Bayesian Filtering approach.
We present recognition results on 18 trials per-
formed by regular users. In future work, we aim to
test our recognition framework in a study with per-
sons with cognitive disabilities. An average recog-
nition rate of 84.5% using a BN with a NaiveBayes
structure shows that our framework is suitable to user
behavior recognition for an automatic prompting sys-
tem in a complex real-world scenario: The Bayesian
Filtering approach can deal with the specific require-
ments like small amount of training data for user be-
haviors and arbitrary behavior lengths. Furthermore,
the framework is applicable to other tasks and easily
extendable with different classifiers due to the hierar-
chical structure.
ACKNOWLEDGEMENTS
The authors would like to thank the inhabitants and
caregivers of Haus Bersaba for their high motiva-
tion to cooperate in the project ’Task Assistance for
Persons with Cognitive Disabilities’ (TAPeD) of the
Cognitive Interaction Technology Center of Excel-
lence (CITEC), Bielefeld University.
REFERENCES
Galata, A., Johnson, N., and Hogg, D. (2001). Learning
variable-length Markov models of behavior. Com-
puter Vision and Image Understanding, 81(3):398–
413.
Hoey, J., Ploetz, T., Jackson, D., Monk, A., Pham, C., and
Olivier, P. (2011). Rapid specification and automated
generation of prompting systems to assist people with
dementia. Pervasive and Mobile Computing, 7(3):299
– 318.
Hoey, J., Poupart, P., Bertoldi, A. v., Craig, T., Boutilier, C.,
and Mihailidis, A. (2010). Automated handwashing
assistance for persons with dementia using video and
a partially observable markov decision process. Com-
puter Vision and Image Understanding, 114:503–519.
Moore, D., Essa, I., and Hayes, M. (1999). Object Spaces:
Context Management for Human Activity Recogni-
tion. In AVBPA’99, 2nd Int. Conf. on Audio-Visual
Biometric Person Authentication, Washington, DC.
Nguyen, N., Phung, D., Venkatesh, S., and Bui, H. (2005).
Learning and detecting activities from movement tra-
jectories using the hierarchical hidden markov model.
In CVPR’05, Int. Conf. on Computer Vision and Pat-
tern Recognition, volume 2.
Oliver, N., Horvitz, E., and Garg, A. (2002). Layered repre-
sentations for human activity recognition. In ICMI’02,
Int. Conf. on Multimodal Interfaces, Pittsburgh, PA.
Patterson, D. J., Fox, D., Kautz, H., and Philipose, M.
(2005). Fine-grained activity recognition by aggregat-
ing abstract object usage. In ISWC’05, IEEE Int. Sym-
posium on Wearable Computers, Washington, DC,
USA.
Pusiol, G., Patino, L., Bremond, F., Thonnat, M., and
Suresh, S. (2008). Optimizing Trajectories Cluster-
ing for Activity Recognition. In MLVMA’08, 1st Int.
Workshop on Machine Learning for Vision-based Mo-
tion Analysis, Marseille, France.
Robertson, N. and Reid, I. (2006). A general method for
human activity recognition in video. Computer Vision
and Image Understanding, 104:232–248.
Ryu, H. and Monk, A. (2009). Interaction Unit Analy-
sis: A New Interaction Design Framework. Human-
Computer Interaction, 24(4).
Subramanya, A., Raj, A., Bilmes, J. A., and Fox, D. (2006).
Recognizing activities and spatial context using wear-
able sensors. In UAI ’06, 22nd Conference on Uncer-
tainty in Artificial Intelligence, Cambridge,MA, USA.
ICPRAM 2012 - International Conference on Pattern Recognition Applications and Methods
170