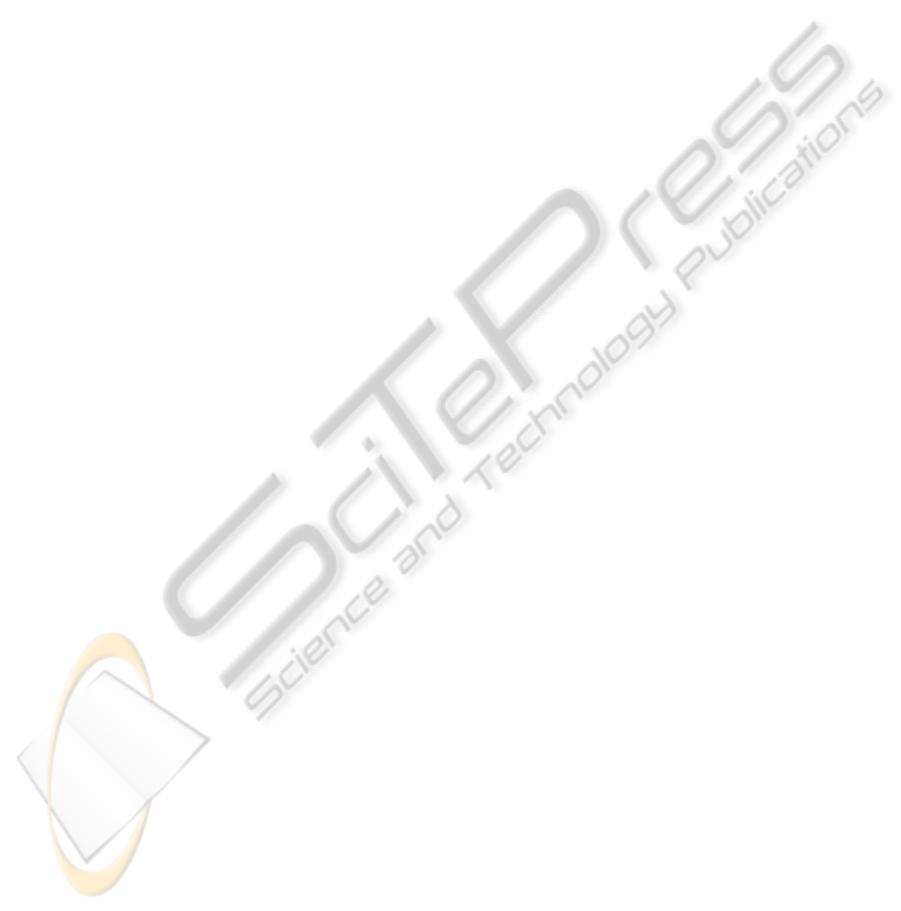
tically reduced ranging from approximately 1100
features with χ = 10% to about 100 features with
χ = 1%. It produces a strong improvement in
terms of time and storage costs.
• Gmean results are more reliable than those of Acc
because both databases have an imbalanced num-
ber of gait sequences corresponding to each gen-
der. For example, for the particular case of SO-
TON and 1NN classifier, a relatively high accu-
racy of about 80% is obtained with the original
GEI method, but it hides an inadmissible 40% of
success on the women class with an almost per-
fect classification rate for the men class (98%). In
this case, Gmean better represents this biased be-
haviour with a low value of about 60%.
• The proposed method obtains Gmean and Acc val-
ues similar to those of the original GEI, but with a
drastic dimensionality reduction. For SVM clas-
sifier, the best balanced trade-off between perfor-
mance and number of features might correspond
to the 3% Mask. However, for 1NN classifier, the
best solution is that with the highest dimensional-
ity reduction (1% Mask). This classifier shows a
tendency to improve its results as the number of
features decreases. In fact, the worst results cor-
respond to the original GEI, i.e., with all features.
A possible reason is that the number of samples
is too low in comparison with the number of fea-
tures, what produces a very spread feature space.
By considering both performance and number of
features, the best solution is probably that in which
1NN classifier is used with the 1% Mask, since differ-
ences with the best SVM results are not significant.
5 CONCLUSIONS
In this paper, an ANOVA-based algorithm has been
used to select the most relevant GEI features for gen-
der classification. The experiments carried out on two
large databases with a SVM and a 1NN classifiers
have shown that a similar performance to that of the
original GEI can be achieved by using only its most
discriminative information, what leads to an impor-
tant reduction in computing time and storage require-
ments. In particular, the 1NN approach has obtained
the highest success rates (comparable or better than
those of SVM) with the lowest number of features.
With respect to future work, a more comprehen-
sive study including other feature selection/extraction
methods should be addressed. In addition, the param-
eter χ should be automatically estimated by using a
validation set, since its value might depend on the sin-
gularities of the gallery set. In SOTON, the higher
imbalanced ratio produces worse results, thus some
techniques to deal with imbalance should be applied
in order to improve the overall Gmean result.
REFERENCES
Bashir, K., Xiang, T., and Gong, S. (2008). Feature selec-
tion on gait energy image for human identification. In
Proc. IEEE Int’l Conf. on Acoustics, Speech and Sig-
nal Processing, ICASSP 2008, pages 985–988.
Boser, B. E., Guyon, I. M., and Vapnik, V. N. (1992). A
training algorithm for optimal margin classifiers. In
Proc. 5th Annual Workshop on Computational Learn-
ing Theory, pages 144–152.
Brown, M. B. and Forsythe, A. B. (1974). The small sample
behavior of some statistics which test the equality of
several means. Technometrics, 16(1):129–132.
CASIA (2005). CASIA Gait Database. http://www.sinobio
metrics.com.
Cutting, J. and Kozlowski, L. (1977). Recognizing friends
by their walk: Gait perception without familiarity
cues. Bulletin of the Psychonomic Society, 9:353–356.
Davis, J. and Gao, H. (2004). Gender recognition from
walking movements using adaptive three-mode PCA.
In IEEE CVPR, Workshop on Articulated and Non-
rigid Motion, volume 1.
Han, J. and Bhanu, B. (2006). Individual recognition us-
ing gait energy image. IEEE Transactions on Pattern
Analysis and Machine Intelligence, 28(2):316–322.
Huang, G. and Wang, Y. (2007). Gender classification based
on fusion of multi-view gait sequences. In Proc. 8th
Asian Conf. Computer Vision, volume 1.
Johansson, G. (1975). Visual motion perception. Scientific
American, 6(232):76–80.
Kozlowski, L. and Cutting, J. (1977). Recognizing the sex
of a walker from a dynamic point-light display. Per-
ception & Psychophysics, 21:575–580.
Li, X., Maybank, S., Yan, S., Tao, D., and Xu, D.
(2008). Gait components and their application to gen-
der recognition. IEEE Trans. SMC-C, 38(2):145–155.
Makihara, Y., Mannami, H., and Yagi, Y. (2011). Gait anal-
ysis of gender and age using a large-scale multi-view
gait database. LNCS. Computer Vision ACCV 2010,
6493:440–451.
Provost, F. and Fawcett, T. (1997). Analysis and visual-
ization of classifier performance: Comparison under
imprecise class and cost distributions. In Proc. of the
3rd ACM SIGKDD, pages 43–48.
Shutler, J., Grant, M., Nixon, M. S., and Carter, J. N. (2002).
On a large sequence-based human gait database. In
Proc. 4th Int’l Conf. on RASC, pages 66–71.
Yoo, J., Hwang, D., and Nixon, M. (2005). Gender classifi-
cation in human gait using support vector machine. In
Proc. ACIVS, pages 138–145.
Yu, S., Tan, T., Huang, K., Jia, K., and Wu, X. (2009). A
study on gait-based gender classification. IEEE Trans-
actions on Image Processing, 18(8):1905–1910.
ICPRAM 2012 - International Conference on Pattern Recognition Applications and Methods
424