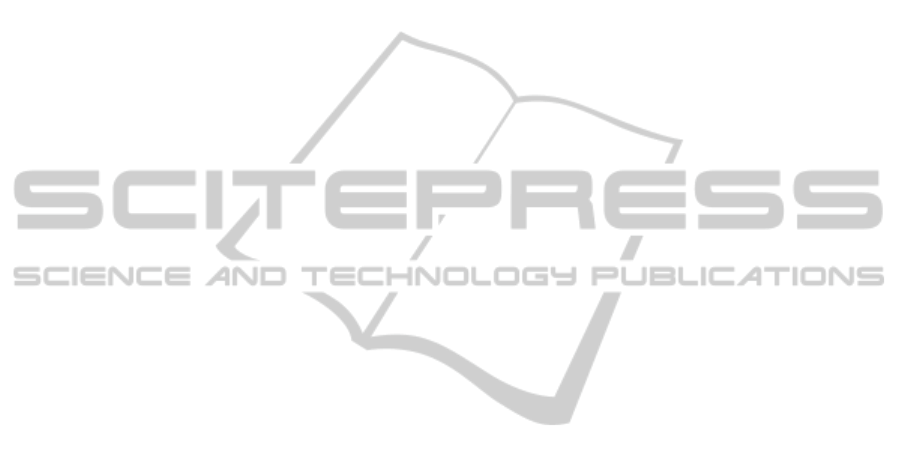
turbulent frames). Since the algorithm for choosing
frames is based on a TNI implementation and not on
pitch, the frames selected as turbulent contain
important information about the “normality” or the
“pathology” of the speaker. While in the “normal”
cases these frames correspond mostly to fricative
sounds and other unvoiced consonants, in the case of
the pathological speakers the frames also include
vowels with low quality or even whispered (which
does not happen in the case of the classifier with
non-turbulent frames). The classifier with turbulent
frames thus has more relevant data to characterize
and distinguish between both classes and for this
reason it can perform better than the others.
Some tests were also performed in order to
evaluate the influence of the number of context
frames and the number of hidden neurons on the
classifiers’ performance. As expected, the results
proved that the classifiers’ performance decreased as
the number of context frames decreased and/or the
number of hidden neurons also decreased. In
addition to the tests described, some others using
similar MLPs neural networks but with two outputs
instead of one were also performed. The results
obtained were the same as the ones stated before,
which confirms the choice to train classifiers with
only one output.
The results presented this section demonstrate
that the combination of continuous speech along
with turbulent information produces excellent
normal/pathological discrimination results when
using the KayPentax database. However, it does not
imply that the three classifiers succeeded in
obtaining the fundamental cues for “normality” and
“pathology”, independently of the database or the
text. In the literature so far produced it is not
common to see this sort of analysis, which can show
the true meaning of the results obtained.
5 CONCLUSIONS
In this work an algorithm to discriminate normal
from pathological speakers based on the analysis of
turbulent information of continuous speech is
presented. All previous works on this subject assume
that the unvoiced parts of the acoustic signals have
no useful information, which justifies the selection
of only voiced speech segments for their
classification systems. In our opinion, these studies
are in fact disregarding important pathological
information that may appear in unvoiced or almost
unvoiced segments, due to a lower quality of the
vowels produced by speakers with pathologies. To
select the less voiced and unvoiced regions of the
signal we propose a segmentation algorithm based
on an acoustic measure called turbulent noise index,
TNI. By properly adjusting a threshold it is possible
to use the TNI measure to select, among others,
meaningful frames containing vowels with low
quality or even whispered speech. Thus, relevant
pathological information is given to the classifier.
The tests performed in a well-known database
resulted in very good discrimination of the
pathological voices. This result must be emphasized
as it shows that it is possible to correctly classify
normal and pathological speakers according to
turbulent information only.
REFERENCES
Deliyski, D., 1993. Acoustic model and evaluation of
pathological voice production, in: 3rd Conference on
Speech Communication and Technology.
Krom, G., 1993. A cepstrum-based technique for
determining a harmonics-to-noise ratio in speech
signals, J. Speech Hear. Res. 36 (1993) 254-266.
Hillenbrand, J., Houde, R-, 1996. Acoustic correlates of
breathy vocal quality: dysphonic voices and
continuous speech, J. Speech Hear. Res. 39.
Michaelis, D., Gramss, T., 1997. H.W. Strube, Glottal-to-
noise excitation ratio – a new measure for describing
pathological voices, Acta Acustica 83 (1997) 700-706.
Kasuya, H., Ogawa, S., Mashima, K., Ebihara, S, 1986..
Normalized noise energy as an acoustic measure to
evaluate pathologic voice, J. Acoust. Soc. Am. 80 (5)
(1986) 1329-1334.
Klingholtz, F., 1990. Acoustic recognition of voice
disorders: a comparative study of running speech
versus sustained vowels, J. Acoust. Soc. Am. 87.
de Krom, G., 1995. Some spectral correlates of pathological
breathy and rough voice quality for different types of
vowel fragments. J. Speech Hear. Res. 38.
Godino-Llorente, J., Fraile, R., Sáenz-Lechón, N., Osma-
Ruiz, V., Gómez-Vilda, P., 2009. Automatic detection
of voice impairments from text-dependent running
speech, J. Biomed. Signal Process. Control 4.
Mitev, P. ,Hadjitodorov, S., 2000. A method for turbulent
noise estimation in voiced signals, J. Med. Biol. Eng.
Comput., 38, 625-631.
DVD 1994. Massachusetts Eye and Ear Infirmary Voice
and Speech Lab, Disordered Voice Database version
1.03, Kay Elemetrics Corp., Pine Brook, NJ.
Boersma, P., 1993. Accurate short-term analysis of the
fundamental frequency and the harmonics-to-noise
ratio of a sampled sound, Proceedings of the Institute
of Phonetic Sciences 17, 97-110.
Martin, A. et al., 1997. The DET curve in assessment of
detection task performance, in: 5th European
Conference on Speech Communication and
technology – EuroSpeech 1997, 1895-1898.
PATHOLOGICAL VOICE DETECTION USING TURBULENT SPEECH SEGMENTS
243