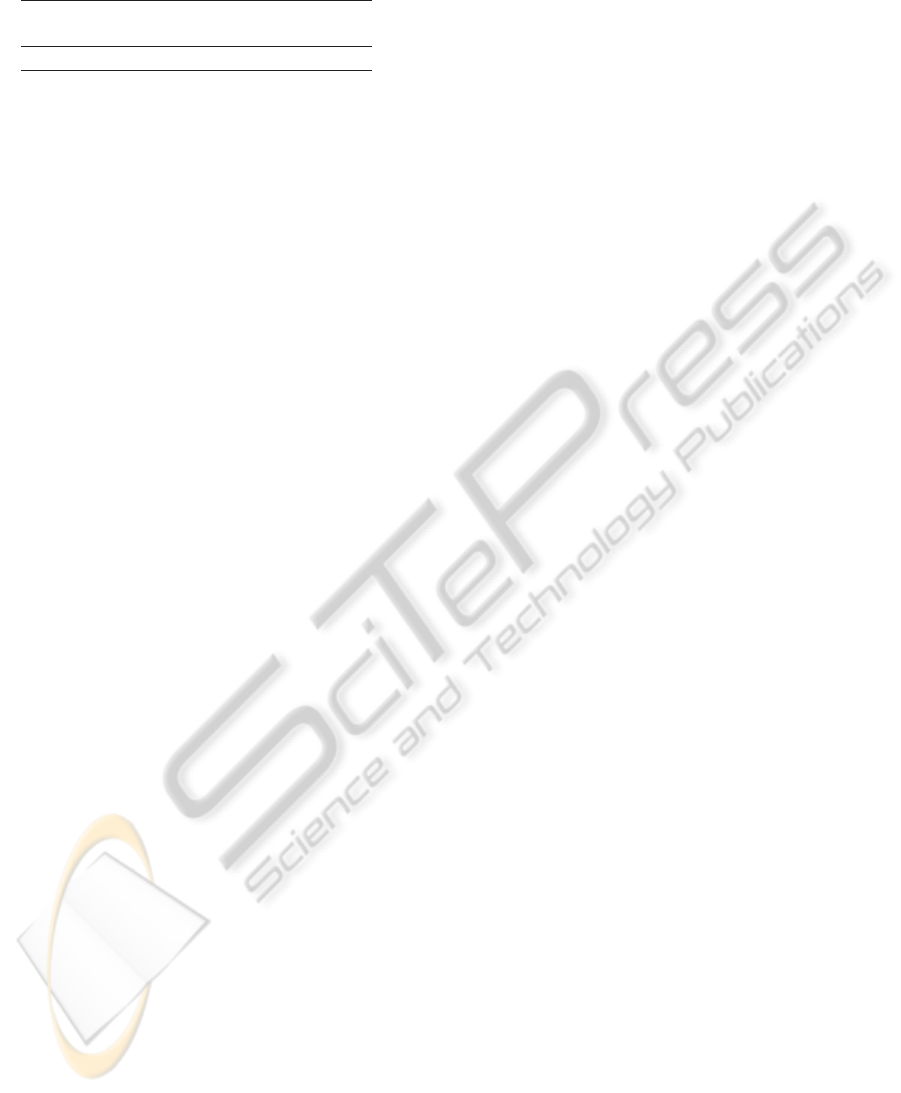
Table 2: The mean rotation error (in degrees) is shown for
our approach under different amount of outliers.
Error
Outliers
10% 20% 30%
MRE (in
◦
) 0.0570 0.0613 0.0667
of this experiment. The performancedoes not degrade
significantly even under a large number of outliers.
5 CONCLUSIONS
In this work, we have proposed a direct monocular
egomotion method based on tracking distant regions.
These regions can be assumed as located at the infi-
nite plane, inducing an infinity homography relation
between two consecutive frames. By tracking that
plane, we are able to estimate the camera rotation.
Once rotation is computed, we cancel its effect on
the images, leaving the resulting motion due to cam-
era translation. This method is simple and performs a
sufficiently stable camera parameter’s estimation.
We successfully apply our algorithm to a sequence
taken from a vehicle driving in an urban scenario. Ro-
tations are accurately estimated, since distant regions
provide strong indicators of camera rotation. In com-
parison to the state-of-the-art methods, our approach
outperforms the considered methods. Moreover, from
the experimental results, we conclude that our method
is also robust to segmentation errors. Occasional mis-
takes can occur when acquired images do not show
distant regions due to obstructions in the field of view.
As future work, we plan to test the proposal using
a monocular segmentation algorithm to distinguish
between close/far regions from single images. These
segmentations will provide enough information about
depth to be used in our egomotion algorithm.
ACKNOWLEDGEMENTS
This work is supported by Spanish MICINN
projects TRA2011-29454-C03-01, TIN2011-29494-
C03-02, Consolider Ingenio 2010: MIPRCV
(CSD200700018), and Universitat Aut`onoma de
Barcelona.
REFERENCES
Adiv, G. (1985). Determining Three-Dimensional Motion
and Structure from Optical Flow Generated by Several
Moving Objects. IEEE Trans. Pattern Anal. Mach.
Intell., 7(4):384 –401.
Baker, S. and Matthews, I. (2004). Lucas-Kanade 20 Years
On: A Unifying Framework. Int. J. Comput. Vision,
56(1):221 – 255.
Burschka, D. and Mair, E. (2008). Direct Pose Estimation
with a Monocular Camera. In Int. Workshop Robot
Vision, pages 440–453.
Cheda, D., Ponsa, D., and L´opez, A. (2010). Camera Ego-
motion Estimation in the ADAS Context. In IEEE
Conf. Intell. Transp. Syst., pages 1415 –1420.
Comport, A., Malis, E., and Rives, P. (2010). Real-
time Quadrifocal Visual Odometry. Int. J. Rob. Res.,
29:245–266.
Ferm¨uller, C. and Aloimonos, Y. (1998). Ambiguity in
structure from motion: sphere versus plane. Interna-
tional Journal of Computer Vision, 28(2):137–154.
Kitt, B., Geiger, A., and Lategahn, H. (2010). Visual
Odometry based on Stereo Image Sequences with
RANSAC-based Outlier Rejection Scheme. In IEEE
Intell. Veh. Symp., pages 486 – 492.
Liang, B. and Pears, N. (2002). Visual Navigation Using
Planar Homographies. In IEEE Int. Conf. Rob. Au-
tom., volume 1, pages 205 – 210.
Lucas, B. and Kanade, T. (1981). An Iterative Image Reg-
istration Technique with an Application to Stereo Vi-
sion. In Int. Joint Conf. Artif. Intell., pages 674–679.
Nist´er, D. (2004). An Efficient Solution to the Five-Point
Relative Pose Problem. IEEE Trans. Pattern Anal.
Mach. Intell., 26(6):756–777.
Obdrzalek, S. and Matas, J. (2010). A Voting Strategy for
Visual Ego-motion from Stereo. In IEEE Intell. Veh.
Symp., pages 382 –387.
Saxena, A., Sun, M., and Ng, A. (2009). Make3D: Learning
3D Scene Structure from a Single Still Image. IEEE
Trans. Pattern Anal. Mach. Intell., 31(5):824–840.
Scaramuzza, D., Fraundorfer, F., and Pollefeys, M. (2010).
Closing the Loop in Appearance-Guided Omnidirec-
tional Visual Odometry by Using Vocabulary Trees.
Rob. Autom. Syst., 58:820–827.
Thanh, T., Kojima, Y., Nagahara, H., Sagawa, R.,
Mukaigawa, Y., Yachida, M., and Yagi, Y. (2010).
Real-Time Estimation of Fast Egomotion with Feature
Classification Using Compound Omnidirectional Vi-
sion Sensor. IEICE Trans. Inf. Syst., 93D(1):152–166.
Tian, T., Tomasi, C., and Heeger, D. J. (1996). Compar-
ison of Approaches to Egomotion Computation. In
IEEE Conf. Comput. Vision Pattern Recogn., pages
315–320.
Wang, H., Yuan, K., Zou, W., and Zhou, Q. (2005). Visual
Odometry Based on Locally Planar Ground Assump-
tion. In IEEE Int. Conf. Inf. Acquisition, pages 59–64.
Zelnik-Manor, L. and Irani, M. (2000). Multi-Frame Esti-
mation of Planar Motion. IEEE Trans. Pattern Anal.
Mach. Intell., 22:1105–1116.
ICPRAM 2012 - International Conference on Pattern Recognition Applications and Methods
430