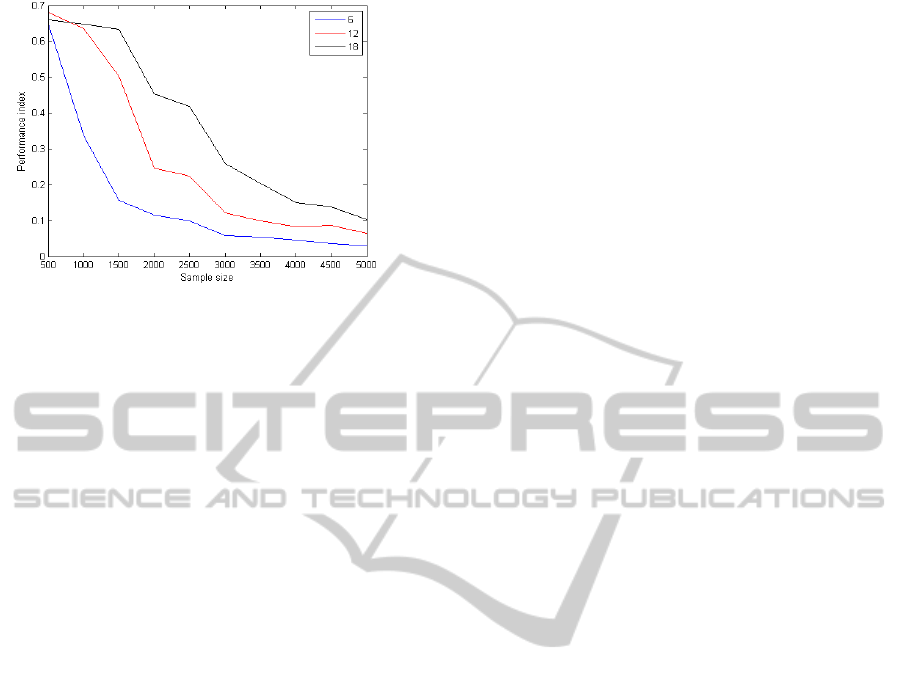
Figure 7: Extended InfoMax PI evolution for different num-
ber of channels as a function of the data length.
previous work (Palmer, 2010) stating that, for EEG
signals, using sphering instead of classical whitening
would result in more physiologically plausible sepa-
rated sources (i.e. more dipolar).
5 CONCLUSIONS AND FUTURE
WORK
Three popular ICA algorithms, often used to anal-
yse EEG signals, have been tested for different data
lengths and number of signals. The main objective
was to define a low bound of data length for robust
separation results, in order to take into account the
short term stationarity of the EEG signals. The re-
sults on simulated mixtures of subgaussian and su-
pergaussian sources are significant enough to extract
an empirical rule for the minimum data length, de-
pending on the number of channels. This result is
based on an original distance measure inspired by the
computer vision community and leads to a reasonable
time length (approximately 10s for 18 channels, sam-
pled at 256 Hz). An auxiliary objective was to test the
convergence robustness of these algorithms for differ-
ent initializations (whitening or sphering). Separation
Performance Index turns out to be similar whether
the decorrelation step is performed using sphering or
whitening method, confirming the robustness of these
algorithms.
Still, these results are not sufficient to conclude
on the impact of different decorrelation methods on
real EEG signals. We are considering further work on
EEG-like (dipolar mixtures) simulated data corrupted
with noise, in order to evaluate the importance of the
decorrelation step from a physiological point of view
(source dipolarity). If such sensitivity is confirmed,
a longer term ambition would be to find an adequate
decorrelation scheme that guarantees the convergence
of ICA algorithms to plausible physiological sources.
Finally, an immediate perspective would be to
extend our study to more HOS algorithms and
more channels, but also to use more realistic time-
structured data allowing the evaluation of SOS BSS
algorithms (SOBI and similar).
REFERENCES
Bell, A. and Sejnowsky, T. (1995). An information-
maximization approach to blind separation and blind
deconvolution. Neural Computation, 7(6):1129–1159.
Cichocki, A. and Amari, S.-I. (2002). Adaptive Blind Signal
and Image Processing. Wiley-Interscience.
Delorme, A. and Makeig, S. (2004). EEGLab: an
open source toolbox for analysis of single-trial EEG
dynamics including independent component analy-
sis. Journal of Neuroscience Methods, 134(1):9–21.
http://sccn.ucsd.edu/wiki/EEGLAB.
Hyvärinen, A. (1999). Fast and robust fixed-point algo-
rithms for independent component analysis. IEEE
Transactions on Neural Networks, 10(3):626–634.
Ma, J., Gao, D., Ge, F., and Amari, S.-i. (2006). A one-bit-
matching learning algorithm for independent compo-
nent analysis. In Rosca, J., Erdogmus, D., Príncipe,
J., and Haykin, S., editors, Independent Component
Analysis and Blind Signal Separation, volume 3889 of
Lecture Notes in Computer Science, pages 173–180.
Springer Berlin / Heidelberg.
Onton, J. and Makeig, S. (2006). Information-based mod-
eling of event-related brain dynamics. In Neuper, C.
and Klimesch, W., editors, Event-Related Dynamics of
Brain Oscillations, volume 159 of Progress in Brain
Research, pages 99 – 120. Elsevier.
Palmer, J. e. a. (2010). Independent component analysis
of high-density scalp EEG recordings. In Indepen-
dent Component Analysis of High-density Scalp EEG
Recordings, 10th EEGLAB Workshop, Jyvaskyla, Fin-
land.
Sanei, S. and Chambers, J. (2007). EEG signal processing.
Wiley-Interscience.
Schomer, D. and Lopes da Silva, F. (2011). Niedermey-
ers’s Electroenephalography: Basic Principles, Clini-
cal Applications and Related Fields. Wolters Kluwer,
Lippincott Willimas & Wilkins, 6th edition.
Smekhov, A. and Yeredor, A. (2004). Optimization of
JADE using a novel optimally weighted joint diago-
nalization approach. In Proc. EUSIPCO, pages 221–
224.
Särelä, J. and Vigario, R. (2003). Overlearning in marginal
distribution-based ICA: Analysis and solutions. Jour-
nal of Machine Learning Research, 4:1447–1469.
Vaseghi, S. and Jetelová, H. (2006). Principal and inde-
pendent component analysis in image processing. In
Proceedings of the 14th ACM International Confer-
ence on Mobile Computing and Networking (MOBI-
COM’06), pages 1–5. Citeseer.
Wu, Y., Wu, B., Liu, J., and Lu, H. (2008). Probabilistic
tracking on Riemannian manifolds. In Pattern Recog-
nition, 2008. ICPR 2008. 19th International Confer-
ence on, pages 1–4. IEEE.
BIOSIGNALS 2012 - International Conference on Bio-inspired Systems and Signal Processing
60