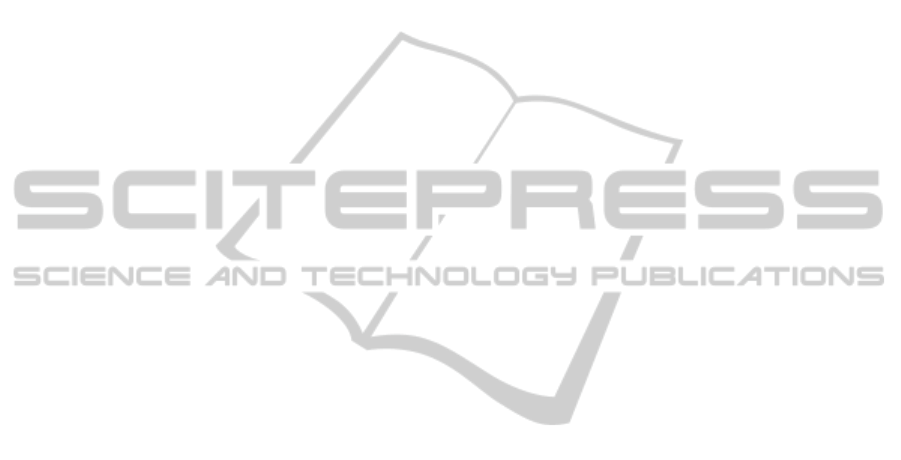
The above results show that flu-related Twitter ac-
tivity is more correlated with flu activity with certain
age-groups within the USA population and the cor-
relation may be better in certain regions compared
to others. This does indicate that training prediction
models that are targeted to specific population seg-
ments is a worthwhile endeavor in a future effort.
7 CONCLUSIONS
In this paper, we have described our approach to
achieve faster, near real time detection and prediction
of the emergence and spread of influenza epidemic,
through continuoustracking of flu related tweets orig-
inating within United States. We showed that apply-
ing text classification on the flu related tweets signif-
icantly enhances the correlation (Pearson correlation
coefficient 0.8907) between the Twitter data and the
ILI rates from CDC.
For prediction, we build an auto-regression with
exogenous input (ARX) model where ILI rate of pre-
vious weeks from CDC formed the autoregressive
portion of the model, and the Twitter data served as
an exogenous input. Our results indicated that while
previous ILI rates from CDC offered a realistic (but
delayed) measure of a flu epidemic, Twitter data pro-
vided a real-time assessment of the current epidemic
condition and can be used to compensate for the lack
of current ILI data.
We observed that the Twitter data was highly cor-
related with the ILI rates across different HHS re-
gions. Our age-based prediction analysis suggested
that for most of the regions, Twitter data best fit the
age groups of 5-24 years and 25-49 years, correlating
well with the fact that these were likely the most ac-
tive age group communities on Twitter. Therefore, flu
trends tracking using Twitter significantly enhances
public health preparedness against influenza epidemic
and other large scale pandemics.
ACKNOWLEDGEMENTS
This research is supported in parts by the National
Institutes of Health under grant 1R43LM010766-01
and National Science Foundation under grant CNS-
0953620.
REFERENCES
Achrekar, H., Gandhe, A., Lazarus, R., Yu, S.-H., and Liu,
B. (2011). Predicting flu trends using twitter data.
IEEE Infocom, 2011 workshop on on Cyber-Physical
Networking Systems (CPNS) 2011.
Centers for Disease Control and Prevention (2009). Flu-
View, a weekly influenza surveillance report.
Culotta, A. (2010). Detecting influenza outbreaks by ana-
lyzing twitter messages. Knowledge Discovery and
Data Mining Workshop on Social Media Analytics,
2010.
Espino, J., Hogan, W., and Wagner, M. (2003). Tele-
phone triage: A timely data source for surveillance of
influenza-like diseases. In AMIA: Annual Symposium
Proceedings.
Ferguson, N. M., Cummings, D. A., Cauchemez, S., Fraser,
C., Riley, S., Meeyai, A., Iamsirithaworn, S., and
Burke, D. S. (2005). Strategies for containing an
emerging influenza pandemic in southeast asia. Na-
ture, 437:209–214.
Ginsberg, J., Mohebbi, M. H., Patel, R. S., Brammer, L.,
Smolinski, M. S., and Brilliant, L. (2009). Detecting
influenza epidemics using search engine query data.
Nature, 457:1012–1014.
Jansen, B., Zhang, M., Sobel, K., and Chowdury, A. (2009).
Twitter power:tweets as electronic word of mouth.
Journal of the American Society for Information Sci-
ence and Technology, 60(1532):2169–2188.
Jordans, F. (2009). WHO working on formulas to model
swine flu spread.
Lazarus, R., Kleinman, K., Dashevsky, I., Adams, C.,
Kludt, P., DeMaria, A., Jr., R., and Platt (2002). Use
of automated ambulatory-care encounter records for
detection of acute illness clusters, including potential
bioterrorism events.
Leskovec, J., Backstrom, L., and Kleinberg, J. (2009).
Meme-tracking and the dynamics of the news cy-
cle. International Conference on Knowledge Discov-
ery and Data Mining, Paris, France, 495(978).
Longini, I., Nizam, A., Xu, S., Ungchusak, K., Han-
shaoworakul, W., Cummings, D., and Halloran, M.
(2005). Containing pandemic influenza at the source.
Science, 309(5737):1083–1087.
Magruder, S. (2003). Evaluation of over-the-counter phar-
maceutical sales as a possible early warning indicator
of human disease. In Johns Hopkins University APL
Technical Digest.
Motoyama, M., Meeder, B., Levchenko, K., Voelker, G. M.,
and Savage, S. (2010). Measuring online service avail-
ability using twitter. Workshop on online social net-
works, Boston, Massachusetts, USA.
Nardelli, A. (2010). Tweetminister.
Sakaki, T., Okazaki, M., and Matsuo, Y. (2010). Earth-
quake shakes twitter users: real-time event detection
by social sensors. In 19th international conference on
World wide web, Raleigh, North Carolina, USA.
Signorini, A., Segre, A. M., and Polgreen, P. M. (2011).
The use of twitter to track levels of disease activity
and public concern in the u.s. during the influenza a
h1n1 pandemic. PLoS ONE, Volume 6 — Issue 5.
Sitaram, A. and Huberman, B. A. (2010). Predicting the
future with social media. In Social Computing Lab,
HP Labs, Palo Alto, California, USA.
Twitter (2011). Information on twitter users age-wise.
HEALTHINF 2012 - International Conference on Health Informatics
70