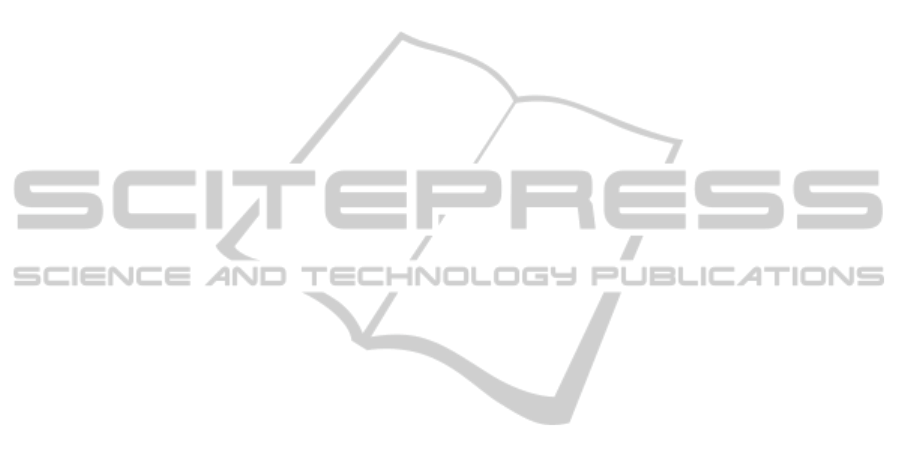
togram approach, while for joint probability distribu-
tions bidimensional histograms were used. In both
cases the number of bins was fixed at 128. The de-
layed mutual information and conditional mutual in-
formation were implemented as a function of a lag.
Each lag was executed in a different core of the com-
puters.
4 RESULTS
For evaluation of the algorithms we used simulated
signals (with a linear and non-linear system with
Gaussian random input signals) and real experimen-
tal signals. In many signal records there were both
200 Hz and 27 Hz data. The time delays from 200 Hz
→ 27 Hz, Fig.2(c) first plot, agreed with time-delay
specifications of the low-pass filter used and when re-
versing input and output, 27 Hz → 200 Hz, the correct
negative lag were obtained.
Simultaneous recordings from interneurons and
motor neurons showed that they received either exci-
tatory or inhibitory inputs during stimulation applied
to the FeCO, Fig.2(a). This conclusion is based on
extensive previous work (Kondoh et al., 1995; New-
land and Kondoh, 1997; Vidal-Gadea et al., 2010) and
parallel work not further reported here.
We evaluated the time delay from recording sites,
1, considering the peaks of delayed mutual informa-
tion and delayed conditional mutual information. Dif-
ferent combinations of these sites were evaluated and
distinct peaks with relatively short delays were clearly
apparent (all graphics in Fig.2(b)). In Fig.2(b) (top
to down, three first graphics) the analyses was per-
formed with delayed mutual information and last plot
from delayed conditional mutual information where
the conditional was 27 Hz signal. Using this approach
we analysed the delayed (conditional) mutual infor-
mation from responses of sensory neurons, spiking
and nonspiking local interneurons and motor neurons
resulting from stimulation of the FeCO.
The results of our analysis are summarised in
Fig.3 in which the presumed interconnections based
on delayed (conditional) mutual information are
shown. The numbers on the arrows give the mean
time-delay (ms).
Some of recorded signal were collected at the
same place in neuronal ganglia from different ani-
mals. In this case, data were average and in few cases
outliers were removed from mean evaluation. In one
case (Nonspiker1 and Nonspiker2 in Fig.3) there were
enough data to cluster into two different sets, with dif-
ferent mean delay value and the graph was rerouted to
accommodate this split state. Also, in part of the data
there were signals with two peaks with similar am-
plitude time delay mutual information that were sta-
tistically significant. In these cases the peaks were
considered as representing different pathways.
The results reveal a number of important points.
First, that neurons sharing similar functions and that
are closely located in the network give similar time-
delays. For example, all identified flexor motor neu-
rons recorded from anterior and posterior groups, that
generate flexion movements of the tibia, have only
short delays of only a few milliseconds relative to
each other. Similarly, the two extensor motor neurons
(the slow, SETi, and fast, FETi) that produce exten-
sion movements of the tibia share information with
similar short delays.
Secondly, nonspiking interneurons can be parti-
tioned into two distinct groups (marked as nonspiker
1 and 2) with short and long time delays with respect
to the stimulus input, reflecting their potential roles
in the neural pathways controlling limb movements.
Third, while the dataset on which this analysis was
based was very large, it should be considered as a
small sample probing network characteristics. It is
impossible to record from all combinations of neu-
rons and so many potential interconnections are miss-
ing or have few repetitions. Additional recordings are
clearly needed for a more complete analysis of the
network.
Clearly it would be desirable to confirm the anal-
ysis with detailed anatomical studies of the neuronal
connections of the actual cells from which the data
was collected. However, this would be challenging
and was not carried out in this data set. In addition,
the connections with longer time-lags are unlikely to
be mono-synaptic, and such connectivities would be
difficult to disentangle from anatomical studies. Thus
while agreement between anatomy and the current
study would provide supportive evidence for the ap-
proach taken, disagreement would not necessarily im-
ply that the proposed signal processing method failed.
It is a strength of the current method that connec-
tions are identified based on information transmis-
sion, rather than direct neuronal connections.
It should also be pointed out that the current
method based on the simultaneous recordings from
only one or two neurons cannot clearly indicate if
connections are direct or indirect.
5 CONCLUSIONS
Previous work (Kondoh et al., 1995; Newland and
Kondoh, 1997) has analysed the anatomical connec-
tions between neurones, but such methods are not able
INFERENCE ABOUT MULTIPLE PATHWAYS IN MOTOR CONTROL LIMB IN LOCUST
73