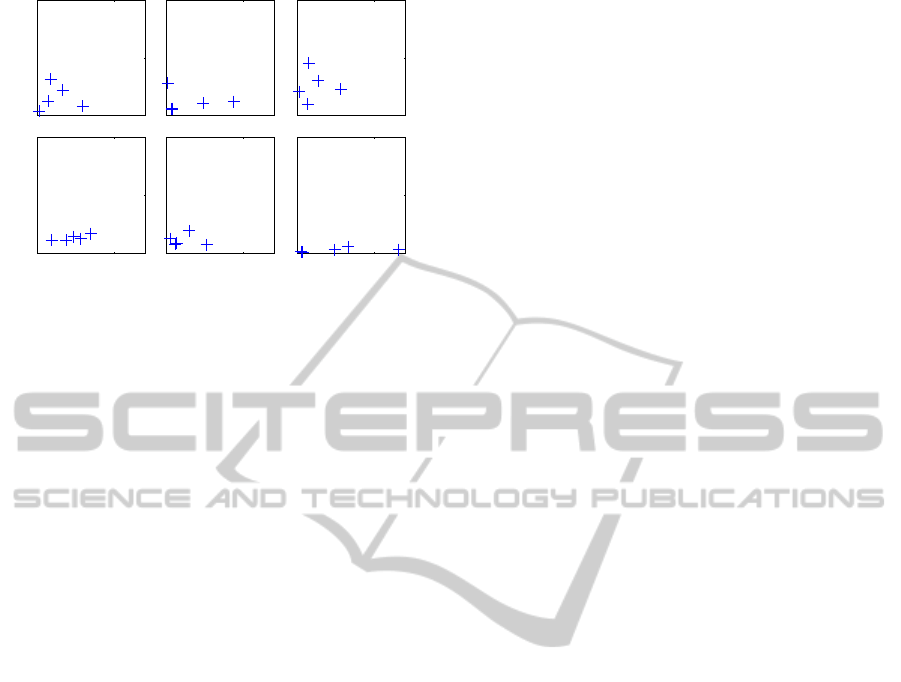
0
5
10
τ (normalisedtimeconstant)
GWN
(r=0.30,p=0.68)
S2
(r=0.00,p=1.00)
S5
(r=0.50,p=0.45)
0 50
0
5
10
S10
(r=0.90,p=0.08)
0 50
TRP/BLP
W1
(r=−0.30,p=0.68)
0 50
W2
(r=0.60,p=0.35)
Figure 3: Relationship between the rate of adaptation, mea-
sured by the time constant of an exponential function fit-
ted to the power values, and the transient response power
(TRP). Both values are normalised by the base line power
(BLP) which is calculated from a 1s window taken from the
recording before stimulation is applied. GWN, S2, S5 and
S10 represent the 50Hz bandlimited GWN, 2Hz, 5Hz and
10Hz sinusoidal stimulation signals respectively. W1 and
W2 are the walking signals. Five recordings for each stim-
ulus signal type were made from FETi in different animals.
Correlation is tested using Spearman rank correlation. We
found no correlation between TRP and the time constant.
an exponential function fitted to the response power of
the motor neurons. We haveshown that the adaptation
rate of the response of two extensor (SETi and FETi)
and one flexor (PlFITi) motor neuron to 27Hz ban-
dlimited GWN stimulation of the FeCO is the same.
Our results are contrary to those found in the study by
Field and Burrows (1982). They found that the PlFITi
motor neuron showed little difference in its response
after 10 s of stimulation, whereas we found a drop in
power of approximately 40% after 3 s. Field and Bur-
rows (1982) also found that the fast and slow flexor
motor neurons exhibited different rates of adaptation
whilst we found that the fast and slow extensor mo-
tor neurons exhibited the same rate of adaptation. It
should be noted that they stimulated the FeCO using
repetitive triangular movements with a frequency of
5Hz and femoral tibial joint angles between 40
◦
and
80
◦
. This is very different from the 27Hz bandlimited
GWN used in the current study and their excursion of
± 20
◦
does not cover the full range of movement that
occurs during walking or kicking. Our results could
also occur because the extensor and flexor neurons
may share common inputs, a property well known to
occur in vertebrate studies (Luca and Erim, 2002).
We have also shown that the adaptation rate of the
FETi motor neuron can be affected by the properties
of the stimulation signal, as has been found in other
work (Fraser et al., 2006). We have used correlation
analysis to show that FETi response power decreases
to its steady state level within the same amount of
time regardless of its transient response power (both
BLP normalised). Further experiments and analysis
are required to understand how this adaptation affects
the function of the reflex response.
ACKNOWLEDGEMENTS
This work was supported by the BBSRC, EPSRC and
the Gerald Kerkut Charitable Trust.
REFERENCES
Bar-Cohen, Y. (2006). Biomimetics-using nature to inspire
human innovation. Bioinspired Biomimetics, 1:1–12.
Bassler, U. (1993). The femur-tibia control system of stick
insects – a model system for the study of the neu-
ral basis of joint control. Brain Research Reviews,
18(2):207 – 226.
Brenner, N., Bialek, W., and de Ruyter van Steveninck,
S. (2000). Adaptive rescaling maximizes information
transmission. Neuron, 26(3):695–702.
Burrows, M. (1996). The Neurobiology of an Insect Brain.
Oxford University Press, first edition.
DiCaprio, R., Wolf, H., and Buschges, A. (2002). Activity-
dependent sensitivity of proprioceptive sensory neu-
rons in the stick insect femoral chordotonal organ.
Journal of Neurophysiology, 88(5):2387–2398.
Field, L. and Burrows, M. (1982). Reflex effects of
the femoral chordotonal organ upon leg motor neu-
rones of the locust. Journal of Experimental Biology,
101(1):265–285.
Fraser, G., Hartings, J., and Simons, D. (2006). Adapta-
tion of trigeminal ganglion cells to periodic whisker
deflections. Somatosensory and Motor Research,
23(3):111–118.
French, A. (1986). The role of calcium in the rapid adap-
tation of an insect mechanoreceptor. The Journal of
Neuroscience, 6(8):2322 –2326.
Gamble, E. and DiCaprio, R. (2003). Nonspiking and spik-
ing proprioceptors in the crab: White noise analysis
of spiking cb-chordotonal organ afferents. Journal of
Neurophysiology, 89(4):1815–1825.
Luca, C. D. and Erim, Z. (2002). Common drive in motor
units of a synergistic muscle pair. Journal of Neuro-
physiology, 87(4):2200–2204.
Nelder, J. A. and Mead, R. (1965). A simplex method
for function minimization. Computer Journal, 7:308–
313.
Newland, P. and Kondoh, Y. (1997). Dynamics of neurons
controlling movements of a locust hind leg iii: Exten-
sor tibiae motor neurons. The Journal of Neurophysi-
ology, 77(5):3297–3310.
Prescott, S. and Sejnowski, T. (2008). Spike-rate coding and
spike-time coding are affected oppositely by different
adaptation mechanisms. The Journal of Neuroscience,
28(50):13649–13661.
NEURAL ADAPTATION IN LOCAL REFLEX CONTROL OF LIMB MOVEMENTS
401