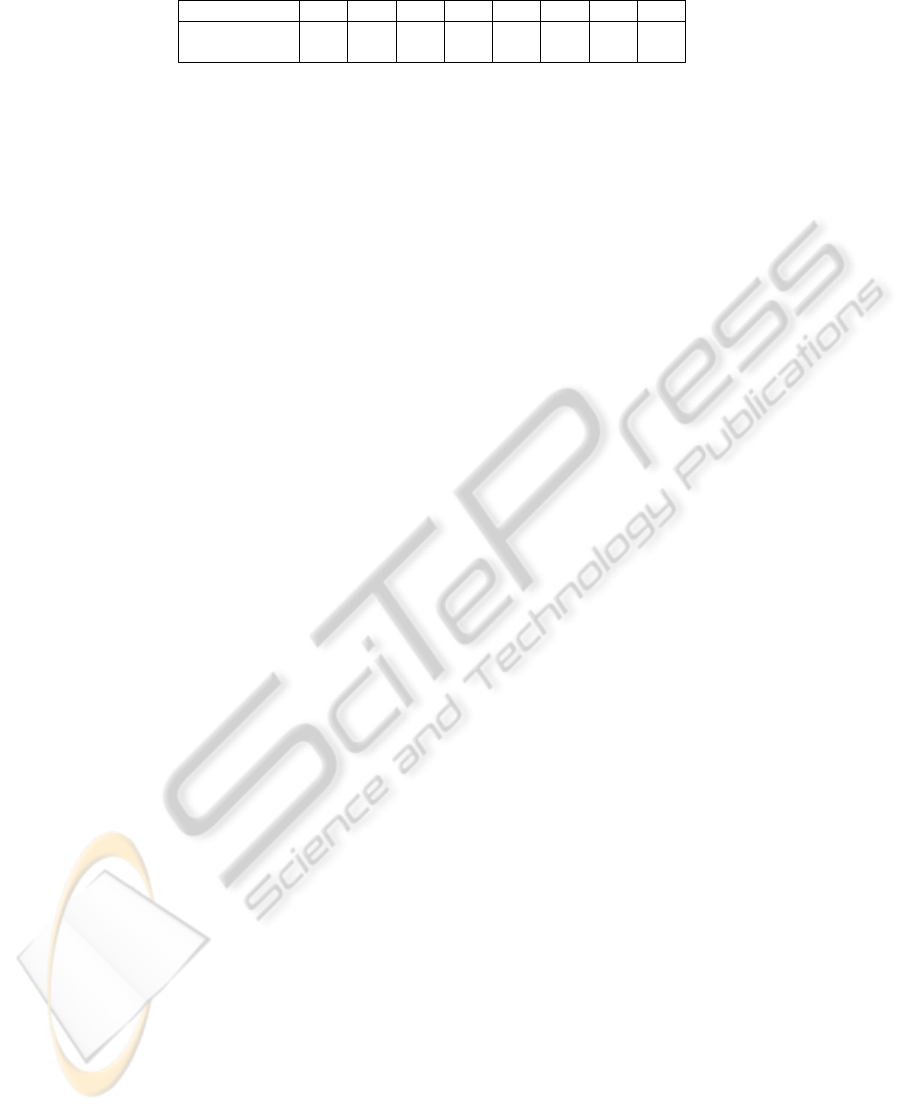
Table 2: SNR between the trajectory and predicted trajectory from the estimated motion fields.
No. of models 1 2 3 4 5 6 7 8
Ground truth 1.37 4.57 4.09 6.23 5.62 5.4 5.48 3.58
Proposed method 1.15 4.42 4.13 6.02 5.52 3.45 5.05 2.96
ground truth trajectories (corresponding to all activi-
ties) and those obtained using the proposed approach
for different numbers of fields estimated by the EM
algorithm. It can be seen that the maximum SNR ob-
tained from the extracted trajectories is close to that
obtained from the ground truth data.
6 CONCLUSIONS
We have proposed a method for automatically com-
puting the trajectories and velocity fields of multi-
ple moving objects in a video sequence, using opti-
cal flow. The trajectories obtained were found to be
close to the manually edited ground truth trajectories,
for a large set of activities occurring in the video se-
quences. The motion fields estimated from these tra-
jectories using the EM method led to an SNR close
to that obtained with the ground truth trajectories.
Hence the proposed method allows fully automatic
extraction of multiple motion fields. Current and fu-
ture work includes extending the method to denser en-
vironments such as crowds of moving people.
ACKNOWLEDGEMENTS
This work was supported by Fundac¸
˜
ao para a
Ci
ˆ
encia e Tecnologia (FCT), Portuguese Ministry
of Science and Higher Education, under projects
PTDC/EEA-CRO/098550/2008 and PEst-OE/EEI/
LA0009/ 2011.
REFERENCES
Barron, J. L., Fleet, D. J., and Beauchemin, S. S. (1994).
Performance of optical flow techniques. International
Journal of Computer Vision, 12:43–77.
Brox, T., Bruhn, A., Papenberg, N., and Weickert, J. (2004).
High accuracy optical flow estimation based on a the-
ory for warping. In ECCV (4), pages 25–36.
Collins, R., Lipton, A., and Kanade, T. (1999). A sys-
tem for video surveillance and monitoring. In Proc.
American Nuclear Society (ANS) Eighth Int. Topical
Meeting on Robotic and Remote Systems, pages 25–
29, Pittsburgh, PA.
Gonzalez, R. C. and Woods, R. E. (2002). Digital Image
Processing. Prentice Hall.
Haritaoglu, I., Harwood, D., and Davis, L. S. (2000). W
4
:
real-time surveillance of people and their activities.
22(8):809–830.
Horn, B. K. P. and Schunck, B. G. (1981). Determining
optical flow. Artificial Intelligence, 17:185–203.
Koller, D., Weber, J., Huang, T., Malik, J., Ogasawara, G.,
Rao, B., and Russel, S. (1994). Towards robust auto-
matic traffic scene analysis in real-time. In Proc. of
Int. Conf. on Pat. Rec., pages 126–131.
Kuhn, H. (1955). The Hungarian method for the assign-
ment problem. Naval research logistics quarterly,
2(1-2):83–97.
Lucas, B. D. and Kanade, T. (1981). An iterative image
registration technique with an application to stereo vi-
sion. pages 674–679.
Ma, Y.-F. and Zhang, H.-J. (2001). Detecting motion ob-
ject by spatio-temporal entropy. In IEEE Int. Conf. on
Multimedia and Expo, Tokyo, Japan.
McKenna, S. J. and Gong, S. (1999). Tracking colour ob-
jects using adaptive mixture models. Image Vision
Computing, 17:225–231.
Nascimento, J., Figueiredo, M., and Marques, J. (2009).
Trajectory analysis in natural images using mixtures
of vector fields. In IEEE Int. Conf. on Image Proc.,
pages 4353 –4356.
Nascimento, J., Figueiredo, M., and Marques, J. (2010).
Trajectory classification using switched dynamical
hidden markov models. IEEE Trans. on Image Proc.,
19(5):1338 –1348.
Ohta, N. (2001). A statistical approach to background sup-
pression for surveillance systems. In Proc. of IEEE
Int. Conf. on Computer Vision, pages 481–486.
Sand, P. and Teller, S. (2008). Particle video: Long-range
motion estimation using point trajectories. Int. J.
Comput. Vision, 80:72–91.
Simoncelli, E. P. (1993). Course-to-fine estimation of vi-
sual motion. In IEEE Eighth Workshop on Image and
Multidimensional Signal Processing.
Souvenir, R., Wright, J., and Pless, R. (2005). Spatio-
temporal detection and isolation: Results on the
PETS2005 datasets. In Proceedings of the IEEE
Workshop on Performance Evaluation in Tracking and
Surveillance.
Stauffer, C., Eric, W., and Grimson, L. (2000). Learn-
ing patterns of activity using real-time tracking.
22(8):747–757.
Turaga, P., Chellappa, R., Subrahmanian, V., and Udrea, O.
(2008). Machine recognition of human activities: A
survey. Circuits and Systems for Video Technology,
IEEE Transactions on, 18(11):1473 –1488.
Veenman, C., Reinders, M., and Backer, E. (2001). Resolv-
ing motion correspondence for densely moving points.
IEEE Trans. on Pattern Analysis and Machine Intelli-
gence, 23(1):54 –72.
Wren, C. R., Azarbayejani, A., Darrell, T., and Pentland,
A. P. (1997). Pfinder: Real-time tracking of the human
body. 19(7):780–785.
ICPRAM 2012 - International Conference on Pattern Recognition Applications and Methods
462