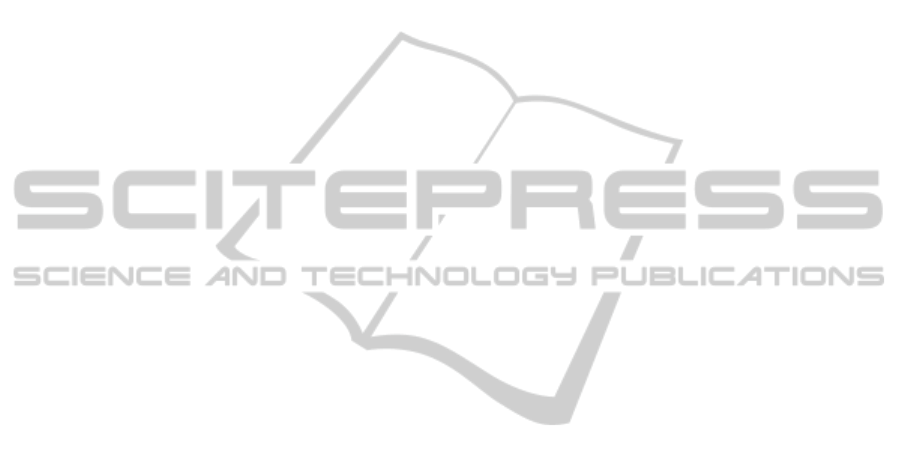
target state. This sets practical limits to the control-
lability for the SNR. If major deviations from pre-
specified scenario model occur the SNR would safely
disengage human interaction from the working enve-
lope and switches to manual override.
5 REACTIVE PLANNER
For continual planning and control the SNR actions in
nondeterminstic situations are synthesized on-the-fly.
The synthesis is based on the interaction model the
SNR has learned by observing and recording Scrub
Nurse and Surgeon’s interactive behavior. The timed
automata model learning algorithm used for that has
been introduced in (Vain et al., 2009). The synthesis
of reactive planning controller(Vain et al., 2011), that
guides the SNR action when being active is based on
the interaction model learned. The intended control
goal of the SNR operation is encoded in the scenario
automaton that specifies the sub-goals of the control,
their temporal order and timing constraints. When-
ever one of the sub-goals has been reached it triggers
resets on guard conditions of the interaction model
and activates driving conditions to reach the subse-
quent goal or one of the alternatives if multiple equal
goals are reachable. In case of violating timing con-
straints or blocking an exception handling procedure
or reset is activated and diagnostics recorded. Spe-
cial care has been taken to address the safety precau-
tions in SNR control. An independent safety monitor-
ing process is running to check if all safety invariants
are satisfied. Whenever safety violation is detected
the disengagementprocedure from continual planning
unit is activated.
6 CONCLUSIONS
The cognitive robot architecture framework described
in this paper supports several innovative aspects
needed for implementing assisting robots in different
applications. Our experience is based on the Scrub
Nurse robot control architecture and software plat-
form development exercise. We demonstrated that
DTRON model-based distributed control framework
provides flexible infrastructurefor interfacing data ac-
quisition and cognitive functions with the ones of de-
liberative control level planning and decision mak-
ing. The architecture also incorporates a module for
learning human interactions and model construction
with reactive planning controller generator and run-
time execution engine. The timed automata based in-
teraction model learning, on-the-fly reactiveplanning,
controller synthesis and online safety monitoring are
steps towards the concept of provably correct robot
design of cognitive assisting robots.
ACKNOWLEDGMENTS
This work was partially supported by the Estonian
Science Foundation under grant No. 7667 and by
Centre of Research Excellence in Dependable Em-
bedded Systems - CREDES.
We want to thank Fuji Miyawaki for the produc-
tive discussions and suggestions on this subject.
REFERENCES
Behrmann, G., David, A., and Larsen, K. G. (2004). A tuto-
rial on uppaal. In Bernardo, M. and Corradini, F., edi-
tors, Formal Methods for the Design of Real-Time Sys-
tems: 4th International School on Formal Methods for
the Design of Computer, Communication, and Soft-
ware Systems, SFM-RT 2004, LNCS, page 200–236.
Springer–Verlag.
DesJardins, M. E., Durfee, E. H., Ortiz Jr, C. L., and
Wolverton, M. J. (1999). A survey of research in dis-
tributed, continual planning. AI Magazine, 20(4):13.
Hall, M., Frank, E., Holmes, G., Pfahringer, B., Reutemann,
P., and Witten, I. H. (2009). The WEKA data min-
ing software: an update. SIGKDD Explor. Newsl.,
11(1):10–18.
Hessel, A., Larsen, K., Mikucionis, M., Nielsen, B., Petters-
son, P., and Skou, A. (2008). Testing Real-Time sys-
tems using UPPAAL. In Formal Methods and Testing,
page 77–117.
Miyawaki, F., Masamune, K., Suzuki, S., Yoshimitsu, K.,
and Vain, J. (2005). Scrub nurse robot system-
intraoperative motion analysis of a scrub nurse and
timed-automata-based model for surgery. Indus-
trial Electronics, IEEE Transactions on, 52(5):1227
– 1235.
Vain, J., Kull, A., K¨a¨aramees, M., Maili, M., and Raiend, K.
(2011). Reactive testing of nondeterministic systems
by test purpose directed tester. In Model-Based Test-
ing for Embedded Systems., Computational Analysis,
Synthesis, and Design of Dynamic Systems, pages
425–452. CRC Press - Taylor & Francis Group, Mas-
sachusetts, USA.
Vain, J., Miyawaki, F., Nomm, S., Totskaya, T., and Anier,
A. (2009). Human-robot interaction learning using
timed automata. In ICCAS-SICE, 2009, pages 2037
–2042.
MODEL BASED CONTINUAL PLANNING AND CONTROL FOR ASSISTIVE ROBOTS
385