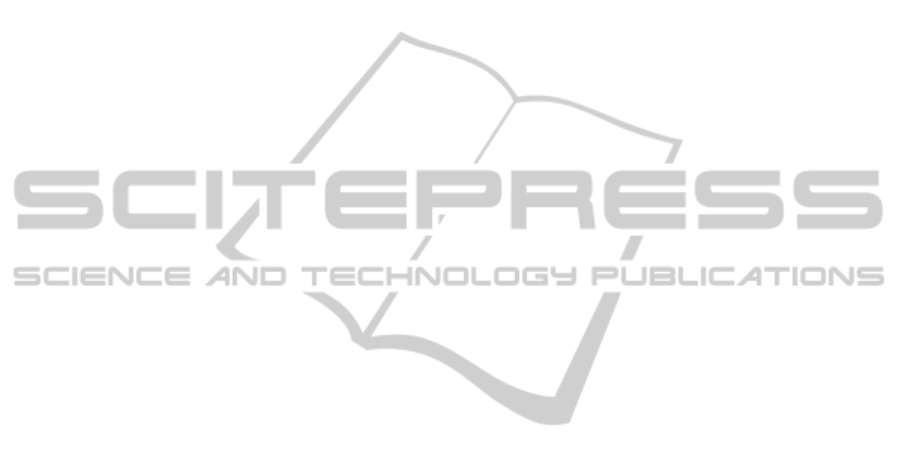
Finally, a kind of analysis about the “additive”
effect of conditions in patient’s death has been done.
For that, the first of the rules shown in Table 3 has
been chosen from the rules generated previously. It
associates alcohol consumption, tobacco
consumption and systolic blood pressure with
patient’s death. This rule expresses that “65% of the
patients with an alcohol consumption in [1.12,
1.69], smoking more than 20 cigarettes/day and with
a systolic blood pressure in [140, 220], were dead”.
To compare the effect of those conditions, alone
and in pairs, rules having the desired conditions have
been selected, and their quality measures are shown
in Table 3.
An analysis of the rules indicates that although
the condition associated to alcohol consumption is
less correlated to death (with a lift value of 1) than
the other two conditions evaluated, when added to
the combination of tobacco consumption and blood
pressure, it increases the confidence from 0.56 to
0.65.
5 CONCLUSIONS
In this work, medical data from an atherosclerosis
study has been used to extract association rules from
it.
Association rules can express unknown
knowledge present in data, in the form of
relationships between the values of the variables.
The method employed is based on a
deterministic approach that generates association
rules without a previous discretization of the
numerical attributes. Discretization can notably
affect the quality of the rules generated, and it is
usually difficult to know the best discretization
technique to apply it to a deterministic algorithm for
a particular dataset.
A variety of rules has been obtained, with good
values of their quality measures, what seems to
support the method employed as a valid way to
generate association rules without a previous
discretization of the numerical attributes.
Also, a particular analysis of a selected rule has
been performed. The rule associates some conditions
with the death of patients object of the study.
ACKNOWLEDGEMENTS
This work was partially funded by the Spanish
Ministry of Science and Innovation, the Spanish
Government Plan E and the European Union through
ERDF (TIN2009-14057-C03-03).
REFERENCES
Agrawal, R., Imielinski, T., Swami, A., 1993. Mining
Association Rules between Sets of Items in Large
Databases. In ACM SIGMOD ICMD, pp. 207-216.
ACM Press.
Bodon, F., 2005. A Trie-based APRIORI Implementation
for Mining Frequent Item Sequences. In 1st
International Workshop on Open Source Data Mining:
Frequent Pattern Mining Implementations, Chicago,
Illinois, pp. 56–65. ACM Press.
Borgelt, C., 2003. Efficient Implementations of Apriori
and Eclat. In Workshop on Frequent Itemset Mining
Implementations. CEUR Workshop Proc. 90, Florida.
Boudík, F., Tomečková, M., Bultas, J., 2004. STULONG
medical project. http://euromise.vse.cz/challenge2004.
Prague.
Brin, S., Motwani, R., Ullman, J.D., Tsur, S., 1997.
Dynamic Itemset Counting and Implication Rules for
Market Basket Data. In Proc. of the ACM SIGMOD
1997, pp. 265-276.
Fayyad, U., Piatetsky-Shapiro, G., Smyth, P., 1996. From
Data Mining to Knowledge Discovery in Databases.
AI Magazine, Vol. 17, pp. 37-54.
Han, J., Kamber, M., 2006. Data Mining: Concepts and
Techniques. Morgan Kaufmann, San Francisco.
Lee, C.-H., 2007. A Hellinger-based Discretization
Method for Numeric Attributes in Classification
Learning. Knowledge-Based Systems, 20(4), 419-425.
Liu, H., Hussain, F., Tan, C., Dash, M., 2002.
Discretization: An Enabling Technique. Data Mining
and Knowledge Discovery, 6(4), 393-423.
Salleb, A., Turmeaux, T., Vrain, C., Nortet, C., 2004.
Mining Quantitative Association Rules in a
Atherosclerosis Dataset. Contribution to the PKDD
Discovery Challenge 2004, http://www.univ-
orleans.fr/lifo/Members/salleb/Challenge2004.
Srikant, R., Agrawal, R., 1996. Mining Quantitative
Association Rules in Large Relational Tables. In Proc.
of the ACM SIGMOD 1996, pp. 1-12.
Tsai, C.-J., Lee, C.-I., Yang, W.-P., 2008. A Discretization
Algorithm Based on Class-Attribute Contingency
Coefficient. Information Science, 178(3), 714-731.
HEALTHINF 2012 - International Conference on Health Informatics
400