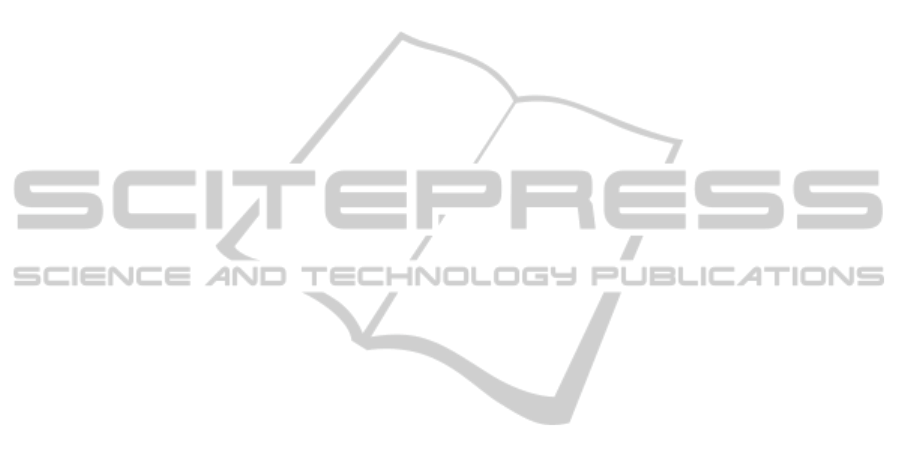
The occurrence of instabilities in the measured
biological signals of (most) non-survivors might be
explained by the fact that, in critical care patients
before dying, the complex closed-loop responses
that operate to keep the organism in equilibrium may
not be evolved to produce a healthy response to
stress, and thus respond in a maladaptive way. This
fits with the work of Lipsitz (2002) who connects
the proper working of physiological systems with
the stability of its dynamic response. However, it
can be expected at one hand that some patients
become dynamically unstable but recover from this
situation or on the other hand that some patients can
die due to very acute problems (e.g. brain
haemorrhage) which are not preceded by periods of
instability in the measured dynamics. Therefore, the
findings in our study need to be validated and
confirmed in a larger sample of patients to evaluate
the concept thoroughly.
In ICU's worldwide, attempts to improve data
processing have centered on computerized systems
and several patient data management (PDMS)
systems have been developed (Toma et al., 2007).
This is software where virtually all patient and
therapy related information is stored on a high
resolution basis. Consequently, there is a great need
for integrating the data and automating the
recognition of several diagnoses, since the quality of
health care systems depends on making the right
decisions at the right time and place (Fonseca et al.,
2009). Without automated systems, clinicians have
to manually extract the necessary information,
which is a time-consuming work that distracts them
from critical tasks and increases the risk of making
mistakes (Spencer et al., 1997). In combination with
recursive modelling techniques, such systems might
allow to model the patients’ dynamic responses in
real-time as a basis for improving personal health
status monitoring.
4 CONCLUSIONS
We found that the patients’ dynamics contains
interesting information when distinguishing between
survivors and non-survivors. A data set of 140
patients was used for the analyses. On the basis of
stability measurements calculated from the
parameters of recursive time series models on
physiological data, we were able to separate
survivors form non-survivors. The best results were
obtained when using blood urea concentration which
gave a true negative fraction of 45/72 (63%). The
true positive fraction was 43/68 (62%). The results
of this study need to be validated and afterwards
confirmed in larger trials, before the described
methodology could be considered in the future in
combination with patient data management systems
to support the physician in on-line monitoring and
decision taking for individual patients.
ACKNOWLEDGEMENTS
We wish to thank the Katholieke Universiteit
Leuven for funding the research reported in this
paper (Interdisciplinary Research project
IDO/03/006).
REFERENCES
Beier, K., Eppanapally, S., Bazick, H. S., Chang, D.,
Mahadevappa, K., Gibbons, F. K. & Christopher, K.
B. (2011). Elevation of blood urea nitrogen is
predictive of long-term mortality in critically ill
patients independent of “normal” creatinine. Crit Care
Med, 39, 305-313.
Belair, J., Glass, L., Heiden, U. A. D. & Milton, J. (1995).
Dynamical Disease - Identification, Temporal Aspects
and Treatment Strategies of Human Illness. Chaos, 5, 1-7.
Box, G. E., Jenkins, G. M. & Reinsel, G. C. (1994). Time
series analysis: forecasting and control. Prentice-Hall
International, New Jersey.
Buchman, T. G. (2004). Nonlinear dynamics, complex
systems, and the pathobiology of critical illness. Curr
Opin Crit Care, 10, 378-382.
Chang, R. W. S., Jacobs, S. & Lee, B. (1988). Predicting
Outcome Among Intensive-Care Unit Patients Using
Computerized Trend Analysis of Daily Apache-Ii
Scores Corrected for Organ System Failure. Intens
Care Med, 14, 558-566.
Chang, R. W. S., Jacobs, S., Lee, B. & Pace, N. (1988).
Predicting Deaths Among Intensive-Care Unit
Patients. Crit Care Med, 16, 34-42.
Clermont, G., Kaplan, V., Moreno, R., Vincent, J. L.,
Linde-Zwirble, W. T., Van Hout, B. & Angus, D. C.
(2004). Dynamic Microsimulation to Model Multiple
Outcomes in Cohorts of Critically Ill Patients. Intens
Care Med, 30, 2237-2244.
Faisst, M., Wellner, U. F., Utzolino, S., Hopt, U. T. &
Keck T. (2010). Elevated blood urea nitrogen is an
independent risk factor of prolonged intensive care
unit stay due to acute necrotizing pancreatitis. J Crit
Care, 25, 105-111.
Fonseca, T., Ribeiro, C. & Granja, C. (2009). Vital Signs
in Intensive Care: Automatic Acquisition and
Consolidation into Electronic Patient Records. J Med
Syst, 33, 47-57.
Glass, L. (2001). Synchronization and Rhythmic Processes
in Physiology. Nature, 410, 277-284.
DYNAMIC AUTOREGRESSIVE MODELLING OF CRITICAL CARE PATIENTS AS A BASIS FOR HEALTH
MONITORING
89