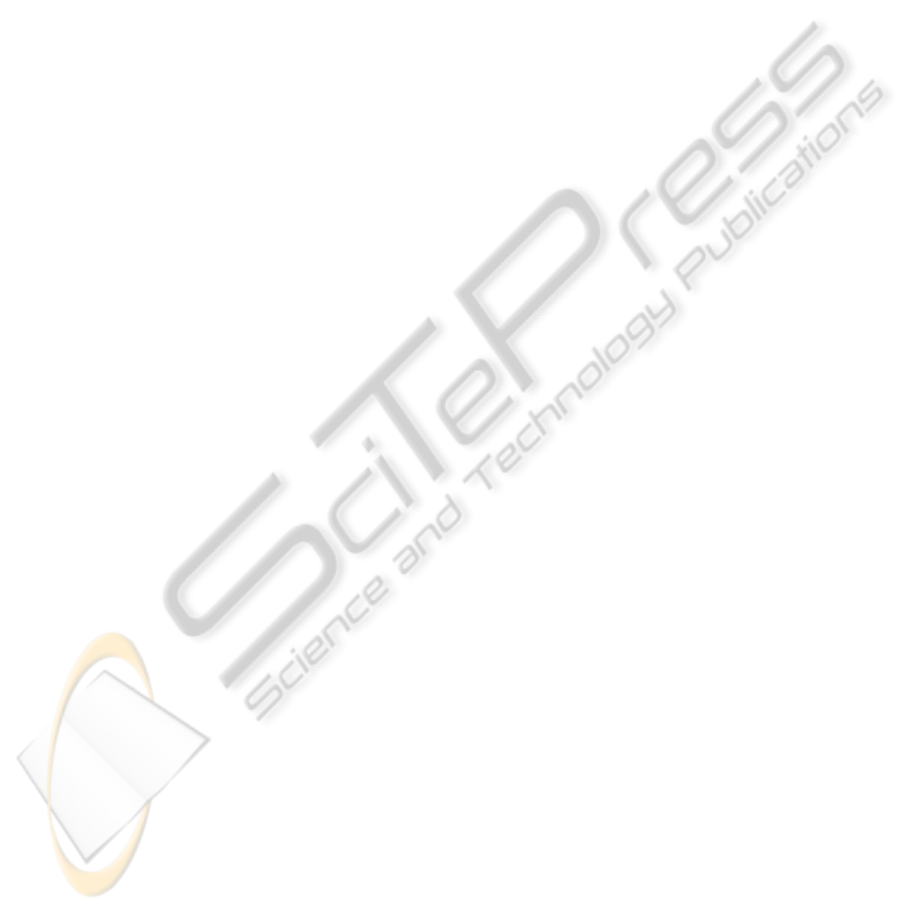
dence measure (e.g. the trace of the covariance ma-
trix). Experiments on synthetic sequences showed the
good performance of this approach.
We used the estimated motion and confidence mea-
sures to improve a super-resolution method. How-
ever, motion estimates are still not as accurate as best
estimators in the Middlebury benchmark. In future we
plan for a improvements for large motions, more com-
plex motion models, e.g. affine motion models, and
motions under changing illumination, e.g. by consid-
ering gradient information or estimation of illumina-
tion changes.
REFERENCES
Bab-Hadiashar, A. and Suter, D. (1998). Robust optic flow
computation. International Journal of Computer Vi-
sion, 29(1):59–77.
Baker, S., Scharstein, D., Lewis, J., Roth, S., Black, M.,
and Szeliski, R. (2011). A database and evaluation
methodology for optical flow. International Journal of
Computer Vision, pages 1–31. 10.1007/s11263-010-
0390-2.
Barron, J. L., Fleet, D. J., and Beauchemin, S. S. (1994).
Performance of optical flow techniques. Int. Journal
of Computer Vision, 12:43–77.
Black, M. and Anandan, P. (1996). The robust estimation of
multiple motions: parametric and piecewise-smooth
flow fields. In Computer Vision and Image Under-
standing, volume 63(1), pages 75–104.
Boley, D., Steinmetz, E., and Sutherland, K. (1996). Recur-
sive total least squares: An alternative to using the dis-
crete kalman filter in robot navigation. In Reasoning
with Uncertainty in Robotics, volume 1093 of Lecture
Notes in Computer Science, pages 221–234. Springer
Berlin / Heidelberg. 10.1007/BFb0013963.
Bruhn, A. and Weickert, J. (2006). A confidence measure
for variational optic flow methods. In Properties for
Incomplete data, pages 283–298. Springer.
Chojnacki, W., Brooks, M. J., and Hengel, A. V. D. (2001).
Rationalising the renormalisation method of kanatani.
Journal of Mathematical Imaging and Vision, 14:21–
38.
Farsiu, S., Robinson, D., Elad, M., and Milanfar, P. (2003).
Fast and robust multi-frame super-resolution. IEEE
Transactions on Image Processing, 13:1327–1344.
Foerstner, W. and Guelch, E. (1987). A fast operator for de-
tection and precise location of distinct points, corners
and centers of circular features. In Proceedings of the
ISPRS Intercommission Workshop on Fast Processing
of Photogrammetric Data, pages 281–305.
Haussecker, H. and Spies, H. (1999). Motion. In Handbook
of Computer Vision and Applications, volume 2, chap-
ter 13, pages 309–396. Academic Press, 1 edition.
Horn, B. and Schunck, B. (1981). Determining optical flow.
Artificial Intelligence, 17:185–204.
Kanatani, K. (1996). Statistical Optimization for Geometric
Computation: Theory and Practice. Elsevier.
Kondermann, C. (2009). Postprocessing and Restoration of
Optical Flows. PhD thesis, Heidelberg University.
Lucas, B. D. and Kanade, T. (1981). An iterative image
registration technique with an application to stereo vi-
sion. In Proceedings of the 7th international joint
conference on Artificial intelligence - Volume 2, pages
674–679, San Francisco, CA, USA.
Nestares, O., Fleet, D. J., and Heeger, D. J. (2000). Likeli-
hood functions and confidence bounds for Total Least
Squares estimation. In Proc. IEEE Conf. on Computer
Vision and Pattern Recognition (CVPR’2000).
Scharr, H. (2005). Optimal filters for extended optical flow.
In Complex Motion, 1. Int. Workshop, G
¨
unzburg, Oct.
2004, volume 3417 of Lecture Notes in Computer Sci-
ence, Berlin. Springer Verlag.
Schuchert, T. (2010). Plant leaf motion estimation using
a 5D affine optical flow model. PhD thesis, RWTH
Aachen University.
Schuchert, T., Aach, T., and Scharr, H. (2010). Range flow
in varying illumination: Algorithms and comparisons.
IEEE Transactions on Pattern Analysis and Machine
Intelligence, 32:1646–1658.
Senst, T., Eiselein, V., Evangelio, R. H., and Sikora, T.
(2011). Robust modified l2 local optical flow esti-
mation and feature tracking. In IEEE Workshop on
Motion and Video Computing, pages 685–690, Kona,
USA.
Simoncelli, E. P. (1999). Bayesian multi-scale differential
optical flow. In Handbook of Computer Vision and
Applications, volume 2, chapter 14, pages 397–422.
Academic Press, San Diego.
Wang, Z. and Qi, F. (2004). On ambiguities in super-
resolution modeling. Signal Processing Letters, IEEE,
11(8):678–681.
Weickert, J., Bruhn, A., Brox, T., and Papenberg, N. (2006).
A survey on variational optic flow methods for small
displacements. In Mathematical Models for Registra-
tion and Applications to Medical Imaging, volume 10
of Mathematics in Industry, pages 103–136. Springer
Berlin Heidelberg.
Werlberger, M., Trobin, W., Pock, T., Wedel, A., Cremers,
D., and Bischof, H. (2009). Anisotropic huber-l1 opti-
cal flow. In Proceedings of the British Machine Vision
Conference (BMVC), London, UK.
OPTICAL FLOW ESTIMATION WITH CONFIDENCE MEASURES FOR SUPER-RESOLUTION BASED ON
RECURSIVE ROBUST TOTAL LEAST SQUARES
469