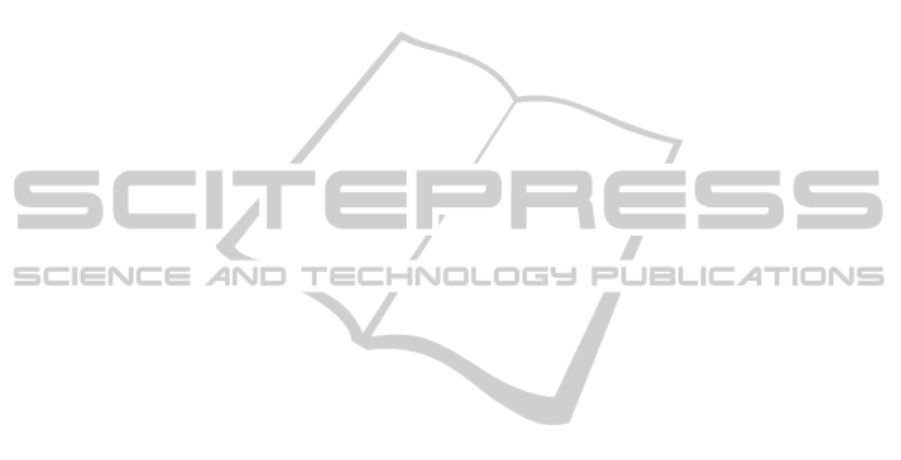
Jorgensen, C. and Dusan, S., 2010. Speech interfaces
based upon surface electromyography, Speech
Communication, Volume 52, Issue 4, pp. 354-366.
Jou, S. Schultz, T. Walliczek, M. Kraft, F. and Waibel, A.,
2006. Towards Continuous Speech Recognition Using
Surface Electromyography. International Conference
of Spoken Language Processing, Interspeech 2006 -
ICSLP, Pittsburgh, PA.
Jou, S. Schultz, T. Waibel, A., 2007. Continuous
Electromyographic Speech Recognition with a Multi-
Stream Decoding Architecture. Proceedings of the
IEEE International Conference on Acoustics, Speech,
and Signal Processing, ICASSP 2007, Honolulu,
Hawaii, US.
Junqua, J.-C. Fincke, S. and Field, K., 1999. The Lombard
effect: a reflex to better communicate with others in
noise. In Proc. IEEE Internat Conf. on Acoust. Speech
Signal Process. (ICASSP). pp. 2083–2086.
Maier-Hein, L. Metze, F. Schultz, T. and Waibel, A.,
2005. Session independent non-audible speech
recognition using surface electromyography, IEEE
Workshop on Automatic Speech Recognition and
Understanding, San Juan, Puerto Rico, pp. 331–336.
Magen, H. S, 1997. The extent of vowel-to-vowel
coarticulation. In English, J. Phonetics 25 (2), pp.
187–205.
Manabe, H. Hiraiwa, A. Sugimura, T., 2003. Unvoiced
speech recognition using EMG-mime speech
recognition. In: Proc. CHI, Human Factors in
Computing Systems, Ft. Lauderdale, Florida, pp. 794–
795.
Manabe, H. Zhang, Z., 2004. Multi-stream HMMfor
EMG-based speech recognition. In: Proc. 26th Annual
International Conf. of the IEEE Engineering in
Medicine and Biology Society, 1–5 September 2004,
San Francisco, California, Vol. 2, pp. 4389–4392.
Martins, P. Carbone, I. Pinto, A. Silva, A. and Teixeira,
A., 2008. European Portuguese MRI based speech
production studies. Speech Communication. NL:
Elsevier, Vol.50, No.11/12, ISSN 0167-6393,
December 2008, pp. 925–952.
Pêra, V. Moura, A. and Freitas, D., 2004. LPFAV2: a new
multi-modal database for developing speech
recognition systems for an assistive technology
application, In SPECOM-2004, pp. 73-76.
Plux Wireless Biosignals, 2011. Portugal, [online]
Available at: http://www.plux.info/ [Accessed 8
September 2011].
Rossato, S. Teixeira, A. and Ferreira, L., 2006. Les
Nasales du Portugais et du Français: une étude
comparative sur les données EMMA. In XXVI
Journées d'Études de la Parole. Dinard, France.
Sá, F. Afonso, P. Ferreira, R. and Pera, V., 2003.
Reconhecimento Automático de Fala Contínua em
Português Europeu Recorrendo a Streams Audio-
Visuais. In The Proceedings of COOPMEDIA'2003 -
Workshop de Sistemas de Informação Multimédia,
Cooperativos e Distribuídos, Porto, Portugal.
Schultz, T. and Wand. M., 2010. Modeling coarticulation
in large vocabulary EMG-based speech recognition.
Speech Communication, Vol. 52, Issue 4, April 2010,
pp. 341-353.
Seikel, J. A. King, D. W. and Drumright, D. G., 2010.
Anatomy and Physiology for Speech, Language, and
Hearing, 4rd Ed., Delmar Learning.
Srinivasan, S. Raj, B. and Ezzat, T., 2010. Ultrasonic
sensing for robust speech recognition. In Internat.
Conf. on Acoustics, Speech, and Signal Processing
2010.
Strevens, P., 1954. Some observations on the phonetics
and pronunciation of modern Portuguese, Rev.
Laboratório Fonética Experimental, Coimbra II, pp. 5–
29.
Teixeira, J. S., 2000. Síntese Articulatória das Vogais
Nasais do Português Europeu. PhD Thesis,
Universidade de Aveiro.
Teixeira, A. and Vaz, F., 2000. Síntese Articulatória dos
Sons Nasais do Português. Anais do V Encontro para
o Processamento Computacional da Língua
Portuguesa Escrita e Falada (PROPOR), ICMC-USP,
Atibaia, São Paulo, Brasil, 2000, pp. 183-193.
Teixeira, A. Moutinho, L. C. and Coimbra, R.L., 2003.
Production, acoustic and perceptual studies on
European Portuguese nasal vowels height. In Internat.
Congress Phonetic Sciences (ICPhS), pp. 3033–3036.
Toda, T. Nakamura, K. Nagai, T. Kaino, T. Nakajima, Y.
and Shikano, K., 2009. Technologies for Processing
Body-Conducted Speech Detected with Non-Audible
Murmur Microphone. In Proceedings of Interspeech
2009, Brighton, UK.
Tran, V.-A Bailly, G. Loevenbruck, H. and Toda, T.,
2009. Multimodal HMM-based NAM to-speech
conversion. In Proceedings of Interspeech 2009,
Brighton, UK.
Trigo, R. L., 1993. The inherent structure of nasal
segments, In Nasals, Nasalization, and the Velum,
Phonetics and Phonology, M. K. Huffman e R. A.
Krakow (eds.), Vol. 5, pp.369-400, Academic Press
Inc.
Wand, M. and Schultz, T., 2009. Towards Speaker-
Adaptive Speech Recognition Based on Surface
Electromyography. In Proc. Biosignals, pp. 155-162,
Porto, Portugal.
Wand, M. Schultz, T., 2011a. Investigations on Speaking
Mode Discrepancies in EMG-based Speech
Recognition, Interspeech 2011, Florence, Italy.
Wand, M. Schultz, T., 2011b. Analysis of Phone
Confusion in EMG-based Speech Recognition. IEEE
International Conference on Acoustics, Speech and
Signal Processing, ICASSP 2011, Prague, Czech
Republic.
Wand, M. Schultz, T., 2011c. Session-Independent EMG-
based Speech Recognition. International Conference
on Bio-inspired Systems and Signal Processing 2011,
Biosignals 2011, Rome, Italy.
Wilpon, J. G. and Jacobsen, C. N., 1996. A Study of
Speech Recognition for Children and the Elderly.
IEEE International Conference on Acoustics, Speech,
and Signal Processing. Atlanta, p. 349.
BIOSIGNALS 2012 - International Conference on Bio-inspired Systems and Signal Processing
100