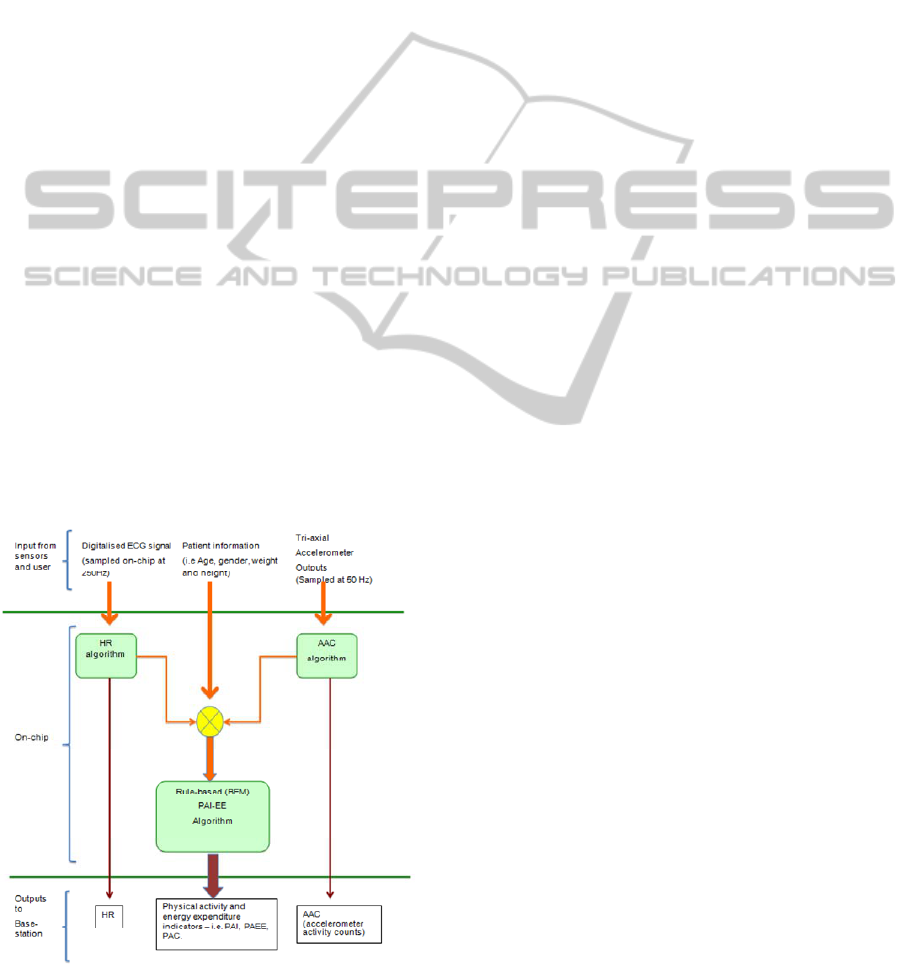
combining accelerometry with HR monitoring,
demonstrating improved estimation of these
parameters when tested in 12 male normal subjects.
The approach relies on a set of rules, regression
equations and thresholds to estimate the PA or EE.
Thus, these parameters are estimated by means of the
selected piecewise function (i.e. one out of the four
available in the branched model) which best suit to the
level and intensity of the activity currently performed.
Later, the BEM approach was implemented in
the ActiHeart® (CamNTech, Cambridge, UK). This
device was shown to be reliable in estimating PA
intensity reliably in several studies (Brage et al.,
2005, Crouter et al., 2007), particularly for activities
such as walking and running. However, one
important drawback of this device is its inability to
transmit data in real time. The latter has a negative
impact on different aspects. First of all, the device
may need to be applied to and removed from the
patient several times until downloaded data reflects
the adequacy of electrode placement. Secondly, the
data logging capability is limited. For these reasons,
this device is neither an option for long-term follow-
up studies requiring several weeks or months; nor for
a final product intended for continuous real-time
feedback of physical activity and calorie expenditure.
3 SENSIUM PA-EE ESTIMATION
The approach adopted for PA-EE estimation can be
explained from Figure 1 as follows:
Figure 1: Block diagram of the Sensium™ PA-EE
algorithm.
Raw ECG and tri-axial accelerometer data are
collected by the Sensium
TM
body worn device at
sampling frequencies of 250 and 50 Hz respectively,
whereas patient information (i.e. age, gender, weight
and height) is manually entered by the user into the
system. The ECG and accelerometer data are fed to
the HR and AAC modules in fixed epoch durations
of 15 seconds.
The HR module was based on the Open Source
ECG Algorithm (OSEA) (Hamilton and Tompkins,
1986, Pan and Tompkins, 1985). The authors of
OSEA have reported high reliability and accuracy of
OSEA when tested using ECG data from the MIT-
BIH database. Nevertheless, a number of
modifications were necessary to adapt the algorithm
to the Sensium™. Firstly, the Sensium™ is
positioned at a non-standard position (lower chest
region, parallel to the Lead 1 position).
Subsequently, the threshold that is used in QRS peak
detection has been adjusted accordingly. Secondly,
extra rules have been included to reject signals
corrupted by motion artefacts. A preliminary
evaluation indicated that these changes did not affect
the efficacy of this algorithm. These results are
available on request to the authors.
The AAC algorithm is based on previous work
by Bouten and colleagues. Firstly, the signal is
filtered using a Butterworth fourth-order band-pass
filter (0.25-6Hz), designed for rejecting spurious
noise without distorting the information
corresponding to physical activities associated with
the intended user population. Of particular interest,
the upper limit of the filter bandwidth was chosen to
attenuate high frequency disturbances occurring
when the swinging foot impacts the ground during
walking at initial contact. This frequency band is in
the region of 15 Hz (Antonsson and Mann, 1985).
After filtering, the accelerometer data corresponding
to each axis is individually rectified and integrated
over 15 seconds to obtain AAC.
In the final stage of the algorithm, the HR and
AAC information are used to estimate the physical
activity intensity. As discussed above, such
estimation is possible by means of a rule-based
algorithm that relies on a set of pre-defined
thresholds, regression equations and weights,
expressing the existing relationship between the
duple HR/AAC and energy expenditure derived
from oxygen consumption (VO2).
BIODEVICES 2012 - International Conference on Biomedical Electronics and Devices
220