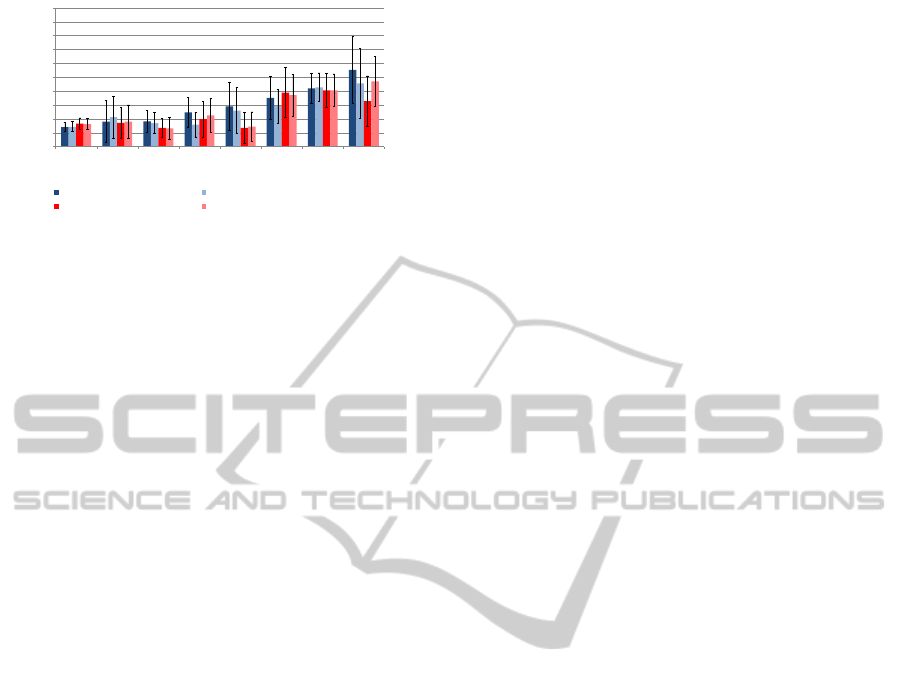
0%
10%
20%
30%
40%
50%
60%
70%
80%
90%
100%
Audible EMG, no Spectral Mapping
Audible EMG, with Spectral Mapping
Whispered EMG, no Spectral Mapping
Whispered EMG, with Spectral Mapping
Figure 9: Word Error Rates of an EMG-based Speech Rec-
ognizer trained on audible and whispered EMG, with and
without spectral mapping. The error bars give the confi-
dence interval at a 5% confidence level.
are created during the training process of the recog-
nizers, allowed the decision tree creation algorithm
to split tree nodes according to the speaking mode,
and then considered the entropy gains which are as-
sociated with tree node splits due to a speaking mode
question.
We showed that the differences in silent speak-
ing style between speakers may be drastic, and that
an evaluation of the decision tree entropy gains
well characterizes the speaker’s ability to speak
silently. Comparing audible speech to silently
mouthed speech, we ascertained that the maximal en-
tropy gain which is due to a speaking mode question
may be used as a measure for the discrepancy between
speaking modes, and that this measure remains stable
even when the spectral mapping algorithm is applied.
Building upon this, we trained, for the first time,
an EMG-based speech recognizer on EMG record-
ings of both audible and whispered speech. It turned
out that whispered speech is, for most speakers, quite
compatible to audible speech, but that in the EMG-
UKA corpus, there is one speaker where the discrep-
ancy between audible and whispered speech in quite
large. We also showed that some accuracy gain can
be achieved with the spectral mapping algorithm.
Based on our decision tree analysis method, pos-
sible future work includes a more detailed phonetic
analysis of the discrepancy between audible and silent
speech, as well as the improvement of the spectral
mapping algorithm to take phone information into ac-
count.
REFERENCES
Bahl, L. R., de Souza, P. V., Gopalakrishnan, P. S., Nahmoo,
D., and Picheny, M. A. (1991). Decision Trees for
Phonological Rules in Continuous Speech. In Proc.
of the IEEE International Conference of Acoustics,
Speech, and Signal Processing (ICASSP), pages 185
– 188, Toronto, Ontario, Canada.
Denby, B., Schultz, T., Honda, K., Hueber, T., and Gilbert,
J. (2010). Silent Speech Interfaces. Speech Commu-
nication, 52(4):270 – 287.
Finke, M. and Rogina, I. (1997). Wide Context Acoustic
Modeling in Read vs. Spontaneous Speech. In Proc.
ICASSP, volume 3, pages 1743–1746.
Janke, M., Wand, M., and Schultz, T. (2010a). A Spec-
tral Mapping Method for EMG-based Recognition of
Silent Speech. In Proc. B-INTERFACE.
Janke, M., Wand, M., and Schultz, T. (2010b). Impact
of Lack of Acoustic Feedback in EMG-based Silent
Speech Recognition. In Proc. Interspeech.
Jou, S.-C., Schultz, T., Walliczek, M., Kraft, F., and Waibel,
A. (2006). Towards Continuous Speech Recogni-
tion using Surface Electromyography. In Proc. Inter-
speech, pages 573 – 576, Pittsburgh, PA.
Kirchhoff, K. (1999). Robust Speech Recognition Using
Articulatory Information. PhD thesis, University of
Bielefeld.
Metze, F. and Waibel, A. (2002). A Flexible Stream Archi-
tecture for ASR Using Articulatory Features. In Proc.
of the International Conference on Spoken Language
Processing (ICSLP), pages 2133 – 2136, Denver, Col-
orado, USA.
Schultz, T. and Waibel, A. (2001). Language Indepen-
dent and Language Adaptive Acoustic Modeling for
Speech Recognition. Speech Communication, 35:31 –
51.
Schultz, T. and Wand, M. (2010). Modeling Coarticulation
in Large Vocabulary EMG-based Speech Recognition.
Speech Communication, 52:341 – 353.
Sch
¨
unke, M., Schulte, E., and Schumacher, U. (2006).
Prometheus - Lernatlas der Anatomie, volume [3]:
Kopf und Neuroanatomie. Thieme Verlag, Stuttgart,
New York.
Wand, M., Janke, M., and Schultz, T. (2011). Investiga-
tions on Speaking Mode Discrepancies in EMG-based
Speech Recognition. In Proc. Interspeech.
Wand, M., Jou, S.-C. S., Toth, A. R., and Schultz, T. (2009).
Impact of Different Speaking Modes on EMG-based
Speech Recognition. In Proc. Interspeech.
Wand, M. and Schultz, T. (2011). Session-independent
EMG-based Speech Recognition. In Proc. Biosignals.
Welch, P. (1967). The use of fast fourier transform for
the estimation of power spectra: A method based
on time averaging over short, modified periodograms.
Audio and Electroacoustics, IEEE Transactions on,
15(2):70–73.
DECISION-TREE BASED ANALYSIS OF SPEAKING MODE DISCREPANCIES IN EMG-BASED SPEECH
RECOGNITION
109