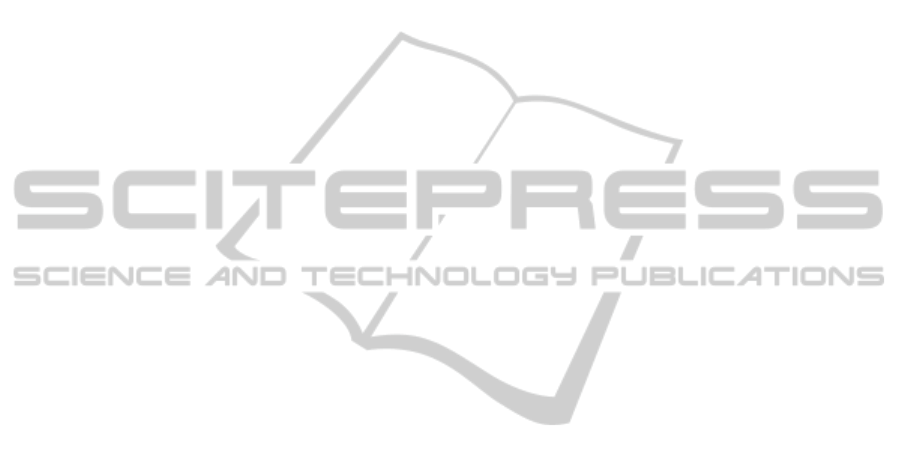
stitution groups that produces more general reactive
motifs. Used as input features of SVM, generated
FCL-based reactive motifs provide highest coverage,
F-measure, precision and recall values without affect-
ing the FP and FN values in the prediction model.
Rule-based learning methods can be investigated
to provide meaningful and understandable informa-
tion to biologists. Among rule-based methods, asso-
ciative classification technique (Liu et al., 1998; Li
et al., 2001; Belohlvek et al., 2007) is recognized to
be more accurate over traditional classification tech-
niques i.e. C4.5 for very large number of classes to
predicted. In the future work, we will increase both
accuracy and explanatory ability of the protein func-
tion classification model using reactive motifs as in-
put features to an associative classification.
ACKNOWLEDGEMENTS
The authors gratefully acknowledge the Thailand Re-
search Fund (TRF) and Kasetsart University for finan-
cial support through the Royal Golden Jubilee Ph.D.
scholarship program (1.0.KU/49/A.1).
REFERENCES
Apweiler, R., Bairoch, A., Wu, C. H., Barker, W. C.,
Boeckmann, B., Ferro, S., Gasteiger, E., Huang,
H., Lopez, R., Magrane, M., Martin, M. J., Natale,
D. A., O’Donovan, C., Redaschi, N., and Yeh, L.-
S. L. (2004). Uniprot: the universal protein knowl-
edgebase. Nucleic Acids Research, 32(Database-
Issue):115–119.
Bairoch, A. (1993). The prosite dictionary of sites and pat-
terns in proteins, its current status. Nucleic Acids Re-
search, 21(13):3097–3103.
Bairoch, A. and Apweiler, R. (2000). The swiss-prot protein
sequence database and its supplement trembl in 2000.
Nucleic Acids Res, 27:49–54.
Belohlvek, R., Baets, B. D., Outrata, J., and Vychodil, V.
(2007). Inducing decision trees via concept lattices.
In CLA, volume 331 of CEUR Workshop Proceedings.
CEUR-WS.org.
Bennett, S. P., Lu, L., and Brutlag, D. L. (2003). 3matrix
and 3motif: a protein structure visualization system
for conserved sequence motifs. Nucleic Acids Re-
search, 31(13):3328–3332.
Boser, B. E., Guyon, I., and Vapnik, V. (1992). A training
algorithm for optimal margin classifiers. In COLT,
pages 144–152.
Cristianini, N. and Shawe-Taylor, J. (2010). An Introduction
to Support Vector Machines and Other Kernel-based
Learning Methods. Cambridge University Press.
Eidhammer, I., Jonassen, I., and Taylor, W. R. (1999).
Structure comparison and structure patterns. JOUR-
NAL OF COMPUTATIONAL BIOLOGY, 7:685–716.
Elloumi, S., Youssef, C. B., and Yahia, S. B. (2004). The
fuzzy classifier by concept localization in a lattice of
concepts. In Proceedings of the CLA 2004 Interna-
tional Workshop on Concept Lattices and their Appli-
cations (CLA).
Henikoff, S. and Henikoff, J. G. (1992). Amino acid sub-
stitution matrices from protein blocks. Proceedings
of the National Academy of Sciences, 89(22):10915–
10919.
Huang, J. Y. and Brutlag, D. L. (2001). The emotif database.
Nucleic Acids Research, 29(1):202–204.
Li, W., Han, J., and Pei, J. (2001). Cmar: Accurate and effi-
cient classification based on multiple class-association
rules. In Proceedings of the 2001 IEEE International
Conference on Data Mining (ICDM), pages 369–376.
Liu, B., Hsu, W., and Ma, Y. (1998). Integrating classi-
fication and association rule mining. In Knowledge
Discovery and Data Mining, pages 80–86.
Nakai, K., Kidera, A., and Kanehisa, M. (1988). Cluster
analysis of amino acid indices for prediction of protein
structure and function. Protein Eng, 2(2):93–100.
Quinlan, J. R. (1993). C4.5: programs for machine learn-
ing. Morgan Kaufmann Publishers Inc., San Fran-
cisco, CA, USA.
Ramu, C., Sugawara, H., Koike, T., Lopez, R., Gibson, T. J.,
Higgins, D. G., and Thompson, J. D. (2003). Multi-
ple sequence alignment with the clustal series of pro-
grams. Nucleic Acids Research, 31(13):3497–3500.
S., K. (2003). Cluster based efficient generation of fuzzy
concepts. In Neural Network World, pages 521–530.
Sander, C. and Schneider, R. (1991). Database of
homology-derived protein structures and the structural
meaning of sequence alignment. Proteins: Structure,
Function, and Genetics, 9(1):56–68.
Schomburg, I., Chang, A., Ebeling, C., Gremse, M., Heldt,
C., Huhn, G., and Schomburg, D. (2004). BRENDA,
the enzyme database: updates and major new de-
velopments. Nucleic acids research, 32(Database
issue):D431–433.
Smith, O., T., A. M., and S., C. (1990). Finding se-
quence motifs in groups of functionally related pro-
teins. Proceedings of the National Academy of Sci-
ences, 87(2):826–830.
Waiyamai, K., Liewlom, P., Kangkachit, T., and Rakthan-
manon, T. (2008). Concept lattice-based mutation
control for reactive motifs discovery. In PAKDD,
pages 767–776.
Yahia, S. B. and Jaoua, A. (2001). Discovering knowledge
from fuzzy concept lattice, pages 167–190. Physica-
Verlag GmbH, Heidelberg, Germany, Germany.
BIOINFORMATICS 2012 - International Conference on Bioinformatics Models, Methods and Algorithms
330