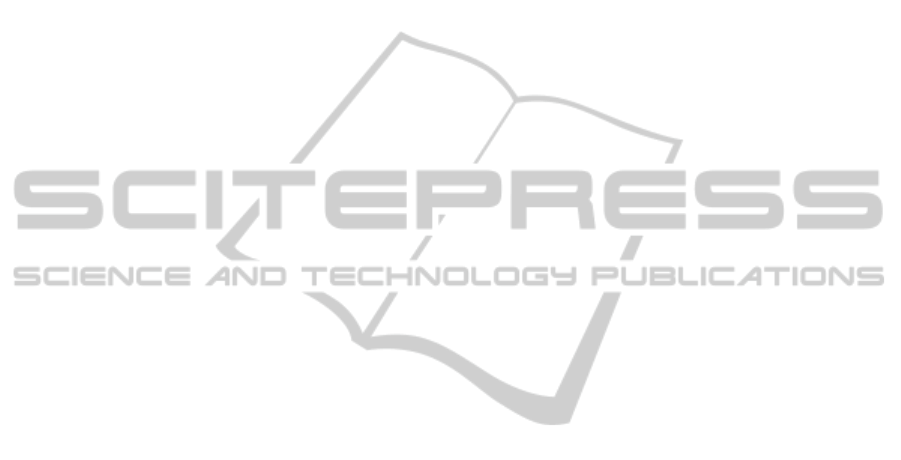
included in this study appear to have fully recovered
from surgery and to be stable. They are well enough
to either be discharged or be provided with a lower
level of care for their remaining time on the ward.
The multivariate model of normality was also
tested on “abnormal” vital-sign data from two patients
who deteriorated sufficiently after surgery to be re-
admitted to the ICU. The model was able to identify
patient deterioration up to 12 hours before the pa-
tient’s readmission to the ICU. These results are omit-
ted for brevity.
5 CONCLUSIONS AND FUTURE
WORK
We have presented the preliminary analysis of data
acquired from patients who were admitted to the
post-operative Upper GI ward after cancer surgery.
We studied the vital-sign distributions at the time of
the patient’s admission to the ward, halfway through
their stay, and near the time of discharge. Although
changes in vital-sign distributions from “normal” pa-
tients between admission to the ward and subsequent
discharge were found, no significant changes in these
distributions were observed from halfway through
their stay to the time of discharge, which suggests that
these patients could have been discharged earlier or
provided with a lower level of care from the halfway
point of their stay on the ward.
A multivariate model of the distribution of vital-
sign data from “normal” patients, which describe the
normal trajectories, was constructed using a kernel
density estimate. The model confirmed the results ob-
tained with the Sammon maps.
Future work will concentrate on the refinement of
existing techniques for the target population group,
and on the improvement of model construction using
more complex dynamical modelling methods. How-
ever, there are a number of limitations that must be
overcome in future analysis. The dataset used in the
analysis described by this paper consisted of measure-
ments of vital signs acquired periodically (every 2 or
4 hours) by ward staff during Phase I of the clinical
trial. These infrequent patient observations combined
with lower nurse:patient ratios (typically, 1:4 to 1:10
in step-down wards), can lead to unnoticed clinical
deterioration and, consequently, to increased numbers
of adverse events. We are developing a continuous
vital-sign monitoring system to provide early warn-
ing of patient deterioration in a robust manner with
low numbers of false alarms.
ACKNOWLEDGEMENTS
The work described in this paper was funded by the
NIHR Biomedical Research Centre Programme, Ox-
ford. MAFP was supported by the RCUK Digital
Economy Programme grant number EP/G036861/1
(Oxford Centre for Doctoral Training in Healthcare
Innovation), and Dr David Clifton was supported by
the Wellcome Trust and the EPSRC under grant num-
ber WT 088877/Z/09/Z. The authors also wish to
thank the support of all clinical staff involved in the
collection of the data used in this investigation.
REFERENCES
Bardell, T., Legare, J. F., Buth, K. J., Hirsch, G. M.,
and Ali, I. S. (2003). ICU readmission after car-
diac surgery. European Journal of Cardio-Thoracic
Surgery, 23(3):354–359.
Bishop, C. M. (2007). Pattern Recognition and Machine
Learning. Springer.
Hann, A. (2008). Multi-parameter monitoring for early
warning of patient deterioration. Ph.D. thesis, Uni-
versity of Oxford.
Kause, J., Smith, G., Prytherch, D., Parr, M., Flabouris,
A., and Hillman, K. (2004). A comparison of an-
tecedents to cardiac arrests, deaths and EMergency in-
tensive care admissions in australia and new zealand,
and the united kingdom–the ACADEMIA study. Re-
suscitation, 62(3):275–282.
Sammon, J. W. (1969). A nonlinear mapping for data struc-
ture analysis. IEEE Transactions on Computers, C-
18(5):401– 409.
Tarassenko, L., Hann, A., and Young, D. (2006). Integrated
monitoring and analysis for early warning of patient
deterioration. British Journal of Anaesthesia, 97(1):64
–68.
Thompson, J. S., Baxter, B. T., Allison, J. G., Johnson,
F. E., Lee, K. K., and Park, W. Y. (2003). Temporal
patterns of postoperative complications. Arch Surg,
138(6):596–603.
Veltkamp, S. C., Kemmeren, J. M., van der Graaf, Y.,
Edlinger, M., and van der Werken, C. (2002). Pre-
diction of serious complications in patients admitted
to a surgical ward. The British Journal of Surgery,
89(1):94–102.
VITAL-SIGN DATA FUSION MODELS FOR POST-OPERATIVE PATIENTS
413