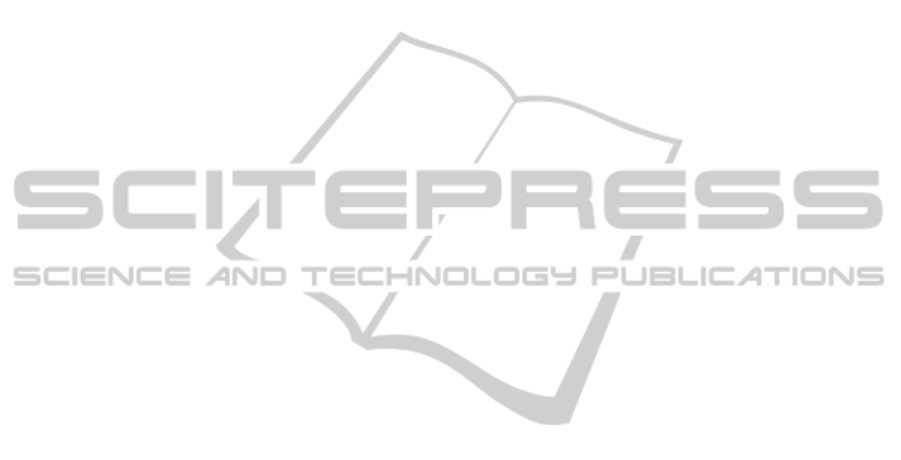
4 DISCUSSIONS AND
CONCLUSIONS
This research presents a method for localizing and
classifying, with high precision, high contrast details
clusters in phantom images. The next step of this
work consists of executing comparative tests
between the techniques presented here with
researches related to the theme.
Statistical measures of the software, which were
presented in the previous section, suggest that the
classification of the structures of interest closes with
the expert vision of the AGEVISA-PB.
Despite of the size of the structures which
simulate the microcalcifications and the possibility
of the confusion with noise, the classification of
these groups represented high success rate of the
system (99.41%). These results are due to the
reduction in size of search regions in the images in
each phantom, as well as the highest contrast of
these structures. With the well-defined boundaries of
the regions, the probability of artefacting the
artefacts and classification from the radiographic
film instead of structures of interest is reduced.
With the implementation of the system in the
AGEVISA-PB, planned for the coming months, it is
expected that the experts will learn how to use the
software and the reports generated by computer
analysis of the phantom images as an aid to the
visual inspection. Thus, part of the process for the
Quality Control in Mammography will be automated
and the subjectivity in the image evaluation may
well be reduced.
After usability tests with the experts and
improvements in the user interface, the system will
be introduced in the establishments which provide
mammography services, to execute their own quality
control in an efficient mode and with the appropriate
frequency.
ACKNOWLEDGEMENTS
CNPq, CAPES and SESU / MEC for the support in the
form of research scholarships and funding.
REFERENCES
Byng, J. W.,Yaffe, M. J., Lockwood, G. A., Little, L. E.,
Tritchler, D. L., Boyd, N. F., 1997. “Automated
analysis of mammographic densities and breast
carcinoma risk.” America Cancer Society. Cancer
80(1):66-74.
Barufaldi, B., Santana, E.F., Batista, L.V., Schiabel, H.,
2011. “Sistema para Controle de Qualidade de
Mamógrafos Através da Análise Computacional de
Imagens de Phantom Utilizando a Ferramenta
WEKA”. In Workshop de Visão Computacional,
Universidade Federal do Paraná, p.16-21.
Carvalho, J. F. G., Silva, M. F. M. P., Lins, M, F. B. P.,
Barbosa, H. T. L., Sousa, J. B., Silva, P. R., Caminha,
F. T., Bulcao, S. B., Morais, M. I., 2006. “AGEVISA-
PB – Relatório Anual de Atividades”.
CBR, 2001. Colégio Brasileiro de Radiologia. Boletim do
CBR nº 165, p. 21.
Gonzales, R. C., Woods, R. E., 2002. “Digital Image
Processing”, 2nd ed. Prentice Hall.
INCA, 2011. “Câncer de Mama: Controle de Qualidade
em Mamografia”. Avaiable em: <http://www2.inca.
gov.br/wps/wcm/connect/cancermama/site/home/contr
ole_qualidade_mamografia/>.
INCA, 2010. “Estimativa 2010: incidência de câncer no
Brasil / Instituto Nacional de Câncer”, ISBN 978-85-
7318-161-6 (98).
Martinez, E. H., Sanjurjo, R. L., 2009. “Minera de datos
aplicada a la detección de Cáncer de Mama”.
Universidad Carlos III de Madrid.
Medeiros, R. B., Elias, S., 2007. “Diagnóstico precoce e
mamografia digital”. Rev. imagem; 29(2): III-IV.
Porto, F. N., 2010 “Análise de Sensibilidade de um
Sistema CAD para Mamografia Digital”. Dissertação
de Mestrado, Universidade Federal de Minas Gerais.
Roveda Junior, D. et al. , 2007. Valores preditivos das
categorias 3, 4 e 5 do sistema BI-RADS em lesões
mamárias nodulares não-palpáveis avaliadas por
mamografia, ultra-sonografia e ressonância magnética.
Radiol Bras, São Paulo, v. 40, n. 2.
Soares, F. A. P., Lopes H. B. M., 2001. “Filme
Radiográfico e Processamento: Curso Técnico de
Radiologia”. Florianópolis: CEFET/SC.
Zweig, M. H.; Campbell, G., 1993. “Receiver-operating
Carachteristic (ROC) plots: a fundamental evaluation
tool in clinical medicine”. Clinical Chemistry, v.39,
n.4, p.361-577, 1993.
BIOSIGNALS 2012 - International Conference on Bio-inspired Systems and Signal Processing
418