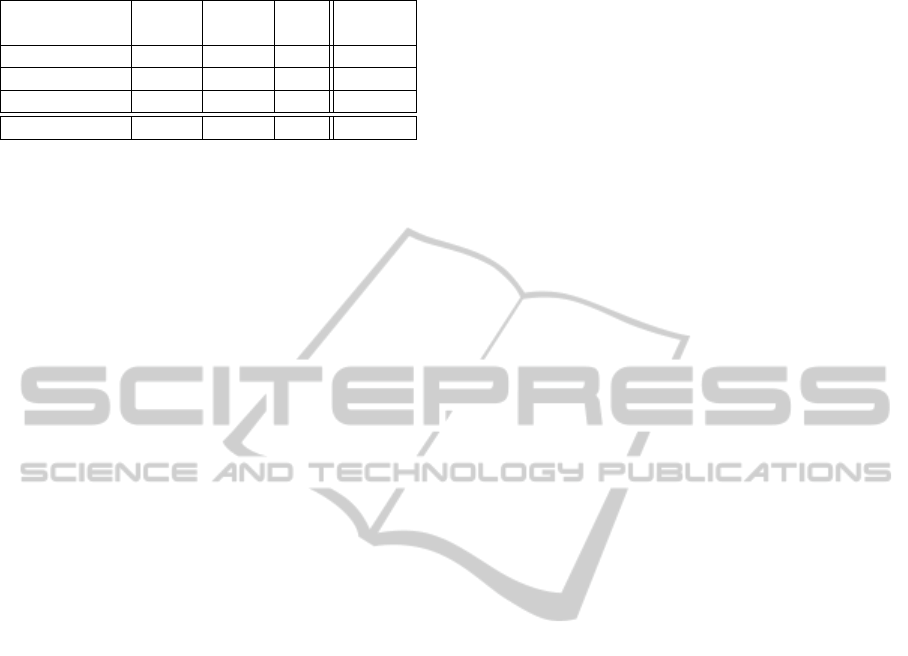
Table 4: Classification results.
P
P
P
P
P
P
P
clinical
detection
NL MCI AD accuracy
NL 11 7 3 52.4%
MCI 1 14 4 73.7%
AD 0 1 9 90.0%
predictive value 91.7% 63.6% 56.3 68.0%
ance normalize in each variable.
Table 4 shows the confusion matrices and the
statistics of classification results using two-phase
classifiers consist of NB
NL/CI
and NB
MCI/AD
. The re-
sults indicate that both the accuracy rate of AD and
the predictive value of NL are equal to or more than
90%. This means that no subject in AD groups are
misclassified into NL group (only one is misclassified
into MCI group), and that subjects classified into NL
group are not all patient with AD (only one should be
in MCI group). This suggests that proposed approach
is adequate practical to screen the elderly with cogni-
tive impairment. The results that the accuracy rate of
MCI is 73.7% and that most of subjects misclassified
are classified into AD group are both relative accept-
able performance for screening tool.
5 CONCLUSIONS
We developed a new technology for early detec-
tion of cognitive impairment in the elderly, focus-
ing on the brain activity during cognitive task. The
detection method is based on the data mining ap-
proach using Bayesian classification and is simple and
non-invasiveprocedure using functional near-infrared
spectroscopy (fNIRS). We proposed a Bayesian clas-
sifier using fNIRS signals, which can discriminate
among elderly individuals with three clinical groups:
normal cognitive abilities (NL), patients with mild
cognitive impairment (MCI), and Alzheimer’s disease
(AD). This paper also reported the examination of the
detection performance by cross-validation, and the re-
sults that both the accuracy rate of AD and the predic-
tive value of NL are equal to or more than 90%. Con-
sequently, the empirical results suggested that pro-
posed approach is adequate practical to screen the el-
derly with cognitive impairment.
ACKNOWLEDGMENTS
We are grateful to SHIMADZU Corporation, Na-
tional Center for Geriatrics and Gerontology, and If-
com Co. Ltd. for fNIRS measurement system, clin-
ical data collection environment, and data collec-
tion, respectively. This work was supported in part
by SENTAN, Japan Science and Technology Agency
(JST), and part by Adaptable and Seamless Tech-
nology Transfer Program through target-driven R&D,
JST, and part by Suzuken Memorial Foundation.
REFERENCES
Buschke, H., Kuslansky, G., Katz, M., Stewart, W. F., Sli-
winski, M. J., Eckholdt, H. M., and Lipton, R. B.
(1999). Screening for dementia with the Memory Im-
pairment Screen. Neurology, 52(2):231–238.
de Leon, M. J., DeSanti, S., Zinkowski, R., Mehta, P. D.,
Pratico, D., Segal, S., Clark, C., Kerkman, D., De-
Bernardis, J., Li, J., Lair, L., Reisberg, B., Tsui, W.,
and Rusinek, H. (2004). Mri and csf studies in the
early diagnosis of alzheimer’s disease. Journal of In-
ternal Medicine, 256(3):205–223.
de Leon, M. J., Mosconi, L., De Santi, K. B. S., Zinkowski,
R., Mehta, P. D., Pratico, D., Tsui, W., Saint Louis,
L. A., Sobanska, L., Brys, M., Li, Y., Rich, K., Rinne,
J., and Rusinek, H. (2007). Imaging and CSF stud-
ies in the preclinical diagnosis of Alzheimer’s disease.
Annals of New York Academy of Sciences, 1097:114–
145.
Domingos, P. and Pazzani, M. (1996). Beyond Indepen-
dence: Conditions for the Optimality of the Simple
Bayesian Classifier. In Proc. of International Confer-
ence on Machine Learning, pages 105–112.
Draper, N. and Smith, H. (1998). Applied Regression Anal-
ysis (3rd edition). John Wiley & Sons.
Folstein, M. F., Folstein, S. E., and McHugh, P. R. (1975).
“Mini-Mental State”: A practical method for grading
the cognitive state of patients for the clinician. J. Psy-
chiat. Res, 12(3):189–198.
Imai, Y. and Hasegawa, K. (1994). The revised Hasegawa’s
Dementia Scale (HDS-R): evaluation of its usefulness
as a screening test for dementia. J. Hong Kong Coll.
Psychiatr., 4(SP2):20–24.
Kato, S., Suzuki, Y., Kobayashi, A., Kojima, T., Itoh, H.,
and Homma, A. (2011). Statistical analysis of the
signal and prosodic sign of cognitive impairment in
elderly-speech: a preliminary study. In Proc. of 5th
International Jount Conference on Biomedical Engi-
neering Systems and Technologies (BIOSTEC 2011),
pages 322–327.
Langley, P., Iba, W., and Thompson, K. (1992). An anal-
ysis of Bayesian classifiers. In Proc. of The Tenth
National Conference on Artificial Intelligence (AAAI-
92), pages 223–228.
Morris, J. C. (1993). The Clinical Dementia Rating
(CDR): Current version and scoring rules. Neurology,
43(11):2412–2414.
Mosconi, L., Berti, V., Glodzik, L., Pupi, A., De Santi, S.,
and de Leon, M. J. (2010). Pre-clinical detection of
Alzheimer’s disease using FDG-PET, with or with-
out amyloid imaging. Journal of Alzheimers’ Disease,
20(3):843–854.
BAYESIAN-BASED EARLY DETECTION OF COGNITIVE IMPAIRMENT IN ELDERLY USING fNIRS SIGNALS
DURING COGNITIVE TESTS
123