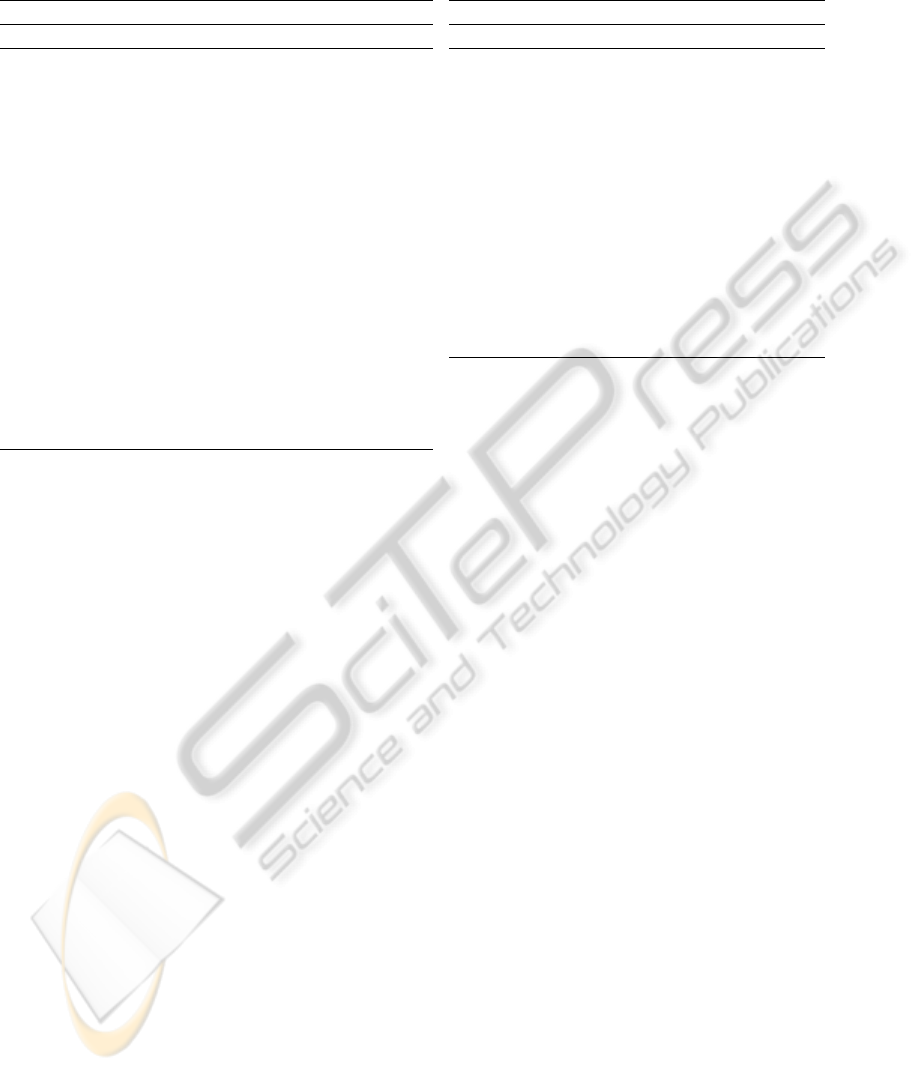
Table 4: Mean accuracy for the best values of q and C for the set of SVM classifiers obtained by the boosting algorithm, using
one Rician mixture per class with K = 4,5,6 components and embeddings
e
e,
¯
e and
b
e. Results with state-of-the-art methods
for ROIs intensity histograms using leave-one-out are also reported.
Boosting
No. of components 4 5 6
Embedding
e
e
Jensen-Shannon 78.55 78.23 77.74
Jensen-Tsallis 79.68 80.16 79.03
Weighted JT
e
k
q
80 79.03 78.39
Weighted JT k
q
79.68 80.16 79.03
Embedding
¯
e
Jensen-Shannon 75 75.97 77.42
Jensen-Tsallis 78.71 78.06 79.84
Weighted JT
e
k
q
78.23 78.06 77.58
Weighted JT k
q
78.71 78.39 78.55
Embedding
b
e
Jensen-Shannon 77.90 76.94 76.61
Jensen-Tsallis 79.35 78.39 78.39
Weighted JT
e
k
q
81.77 78.39 78.06
Weighted JT k
q
80.48 77.90 78.39
State-of-the-art methods
Methodology Accuracy
SVM Best Single ROI
(Cheng et al., 2009a) 73.4
Dissimilarity representations
(Ulas et al., 2011) 78.07
SVM Multiple ROIs
Constellation probab. model + Fisher kernel
(Cheng et al., 2009b) 80.65
Combined dissimilarity representations
(Ulas et al., 2010) 79
Dissimilarity representations
(Ulas et al., 2011) 76.32
a boosting algorithm. The experimental results show
that the proposed methodology outperforms the pre-
vious state-of-the-art methods on the same dataset.
REFERENCES
Abramowitz, M. and Stegun, I. (1972). Handbook of Math-
ematical Functions. Dover, New York.
Bosch, A., Zisserman, A., and Munoz, X. (2006). Scene
classification via plsa. In Proc. of ECCV.
Burbea, J. and Rao, C. (1982). On the convexity of some di-
vergence measures based on entropy functions. IEEE
Trans. on Information Theory, 28(3):489–495.
Cheng, D., Bicego, M., Castellani, U., Cerutti, S., Bel-
lani, M., Rambaldelli, G., Atzori, M., Brambilla, P.,
and Murino, V. (2009a). Schizophrenia classification
using regions of interest in brain MRI. In IDAMAP
Workshop.
Cheng, D., Bicego, M., Castellani, U., Cristani, M., Cerruti,
S., Bellani, M., Rambaldelli, G., Aztori, M., Bram-
billa, P., and Murino, V. (2009b). A hybrid gener-
ative/discriminative method for classification of re-
gions of interest in schizophrenia brain MRI. In MIC-
CAI09 Workshop on Probabilistic Models for Medical
Image Analysis.
Cristianini, N. and Shawe-Taylor, J. (2000). An introduction
to Support Vector Machines and other kernel-based
learning methods. Cambridge University Press.
Dempster, A., Laird, N., and Rubin, D. (1977). Maxi-
mum likelihood from incomplete data via the EM al-
gorithm. Jour. the Royal Statistical Soc. (B), 39:1–38.
Figueiredo, M. and Jain, A. K. (2002). Unsupervised learn-
ing of finite mixture models. IEEE Trans. on Pattern
Analysis and Machine Intelligence, 24:381–396.
Freund, Y. and Schapire, R. (1997). A decision-theoretic
generalization of online learning and an application to
boosting. Jour. Comp. System Sciences, 55:119–139.
Gudbjartsson, H. and Patz, S. (1994). The rician distribution
of noisy MRI data. Magnetic Resonance in Medicine,
34:910–914.
Jaakkola, T. and Haussler, D. (1999). Exploiting generative
models in discriminative classifiers. In Neural Infor-
mation Processing Systems – NIPS.
Lasserre, J., Bishop, C., and Minka, T. (2006). Principled
hybrids of generative and discriminative models. In
Proc. Conf. Computer Vision and Patt. Rec. – CVPR.
Lin, J. (1991). Divergence measures based on Shannon en-
tropy. IEEE Trans. Information Theory, 37:145–151.
Martins, A. F., Smith, N. A., Aguiar, P. M., and Figueiredo,
M. A. T. (2009). Nonextensive information theoretic
kernels on measures. Journal of Machine Learning
Research, 10:935 – 975.
Ng, A. and Jordan, M. (2002). On discriminative vs gener-
ative classifiers: A comparison of logistic regression
and naive Bayes. In Neural Information Processing
Systems – NIPS.
Perina, A., Cristani, M., Castellani, U., Murino, V., and
Jojic, N. (2009). A hybrid generative/discriminative
classification framework based on free-energy terms.
In Proc. Int. Conf. Computer Vision – ICCV, Kyoto.
Rice, S. O. (1944). Mathematical analysis of random noise.
Bell Systems Tech. J., 23:282–332.
Rubinstein, Y. and Hastie, T. (1997). Discriminative vs in-
formative learning. In Proc. 3rd Int. Conf. Knowledge
Discovery and Data Mining, Newport Beach.
GENERATIVE EMBEDDINGS BASED ON RICIAN MIXTURES - Application to Kernel-based Discriminative
Classification of Magnetic Resonance Images
121