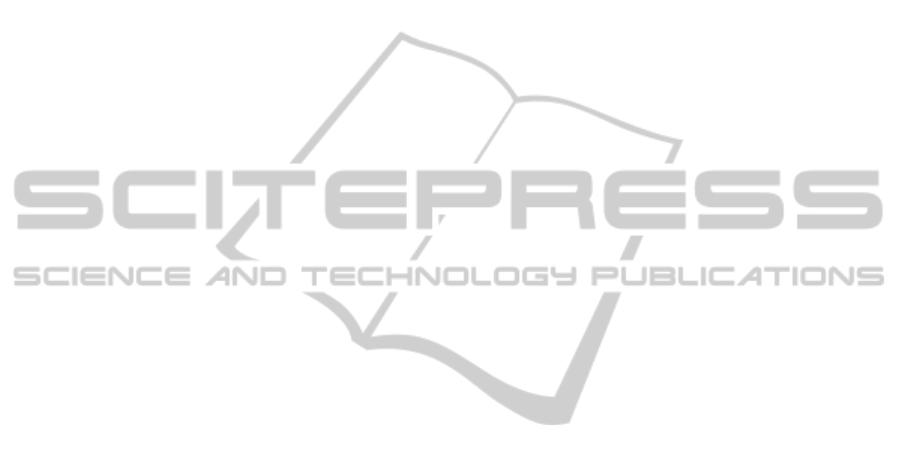
REFERENCES
Baldi, P. and Brunak, S. (2001). Bioinformatics: the ma-
chine learning approach. MIT Press.
Ben-Hur, A., Ong, C. S., Sonnenburg, S., Scholkopf, B.,
and Ratsch, G. (2008). Support vector machines and
kernels for computational biology. PLoS computa-
tional biology.
Black, D. L. (2003). Mechanisms of alternative pre-
messenger RNA splicing. Annual Review of Biochem-
istry.
Blum, A. and Mitchell, T. (1998). Combining labeled
and unlabeled data with Co-Training. In Proceedings
of the eleventh annual conference on Computational
learning theory. ACM.
Brefeld, U. and Scheffer, T. (2004). Co-EM support vec-
tor learning. In In Proceedings of the International
Conference on Machine Learning.
Chasin, L. A. (2007). Searching for splicing motifs. Ad-
vances in Experimental Medicine and Biology.
Chow, L. T., Gelinas, R. E., Broker, T. R., and Roberts, R. J.
(1977). An amazing sequence arrangement at the 5’
ends of adenovirus 2 messenger RNA. Cell.
Collins, M. and Singer, Y. (1999). Unsupervised models
for named entity classification. In In Proceedings of
the Joint SIGDAT Conference on Empirical Methods
in Natural Language Processing and Very Large Cor-
pora.
Dai, W., Xue, G., Yang, Q., and Yu, Y. (2007). Transfer-
ring naive bayes classifiers for text classification. In
In Proceedings of the 22nd AAAI Conference on Arti-
ficial Intelligence.
Dempster, A. P., Laird, N. M., and Rubin, D. B. (1977).
Maximum likelihood from incomplete data via the
EM algorithm. Journal of the Royal Statistical So-
ciety.
Dong, A. and Bhanu, B. (2003). A new semi-supervised
EM algorithm for image retrieval. Computer Vision
and Pattern Recognition.
Dror, G., Sorek, R., and Shamir, R. (2005). Accurate iden-
tification of alternatively spliced exons using support
vector machine. Bioinformatics (Oxford, England).
Gammerman, A., Vovk, V., and Vapnik, V. (1998). Learning
by transduction. In In Uncertainty in Artificial Intelli-
gence. Morgan Kaufmann.
Goldberg, A. B. and Zhu, X. (2006). Seeing stars when
there aren’t many stars: graph-based semi-supervised
learning for sentiment categorization. In Proceedings
of the First Workshop on Graph Based Methods for
Natural Language Processing. Association for Com-
putational Linguistics.
Huang, J. and Ling, C. X. (2005). Using a u c and accuracy
in evaluating learning algorithms. IEEE Transactions
on Knowledge and Data Engineering.
Kabat, J. L., Barberan-Soler, S., McKenna, P., Clawson, H.,
Farrer, T., and Zahler, A. M. (2006). Intronic alterna-
tive splicing regulators identified by comparative ge-
nomics in nematodes. PLoS computational biology.
Kall, L., Canterbury, J. D., Weston, J., Noble, W. S., and
MacCoss, M. J. (2007). Semi-supervised learning
for peptide identification from shotgun proteomics
datasets. Nature methods.
Lawrence, C. E. and Reilly, A. A. (1990). An expec-
tation maximization (EM) algorithm for the identifi-
cation and characterization of common sites in un-
aligned biopolymer sequences. Proteins.
McCallum, A. and Nigam, K. (1998). A comparison of
event models for naive bayes text classification. Di-
mension Contemporary German Arts And Letters.
Moreno, P. J. and Agarwal, S. (2003). An experimental
study of semi-supervised EM. Technical report, HP
Labs.
Nagaraj, S. H., Gasser, R. B., and Ranganathan, S. (2007).
A hitchhiker’s guide to expressed sequence tag (est)
analysis. Briefings in bioinformatics.
Nesvizhskii, A. I., Keller, A., Kolker, E., and Aebersold, R.
(2003). A statistical model for identifying proteins by
tandem mass spectrometry. Analytical Chemistry.
Nigam, K. and Ghani, R. (2000). Analyzing the effective-
ness and applicability of Co-Training. In Proceedings
of the 9th International Conference on Information
and Knowledge Management. ACM.
Nigam, K., McCallum, A. K., Thrun, S., and Mitchell, T.
(2000). Text classification from labeled and unlabeled
documents using EM. Machine Learning.
Pertea, M., Mount, S. M., and Salzberg, S. L. (2007). A
computational survey of candidate exonic splicing en-
hancer motifs in the model plant Arabidopsis thaliana.
BMC bioinformatics.
Provost, F. J., Fawcett, T., and Kohavi, R. (1998). The
case against accuracy estimation for comparing induc-
tion algorithms. In Proceedings of the Fifteenth Inter-
national Conference on Machine Learning. Morgan
Kaufmann Publishers Inc.
Ratsch, G., Sonnenburg, S., and Scholkopf, B. (2005).
Rase: recognition of alternatively spliced exons in
C.elegans. Bioinformatics (Oxford, England).
Rosenberg, C., Hebert, M., and Schneiderman, H. (2005).
Semi-supervised self-training of object detection
models. In Proceedings of the Seventh IEEE Work-
shops on Application of Computer Vision. IEEE Com-
puter Society.
Vapnik, V. N. (1995). The nature of statistical learning the-
ory. Springer-Verlag New York, Inc.
Weston, J., Kuang, R., Leslie, C., and Noble, W. (2006).
Protein ranking by semi-supervised network propaga-
tion. BMC Bioinformatics.
Wu, C. F. J. (1983). On the convergence properties of the
EM algorithm. Annals of Statistics, Vol. 11, No. 1.
Yarowsky, D. (1995). Unsupervised word sense disam-
biguation rivaling supervised methods. In Proceed-
ings of the 33rd annual meeting on Association for
Computational Linguistics.
Zhang, Y.-Q. and Rajapakse, J. C. (2009). Machine learning
in bioinformatics. Wiley.
SEMI-SUPERVISED LEARNING OF ALTERNATIVELY SPLICED EXONS USING EXPECTATION
MAXIMIZATION TYPE APPROACHES
245