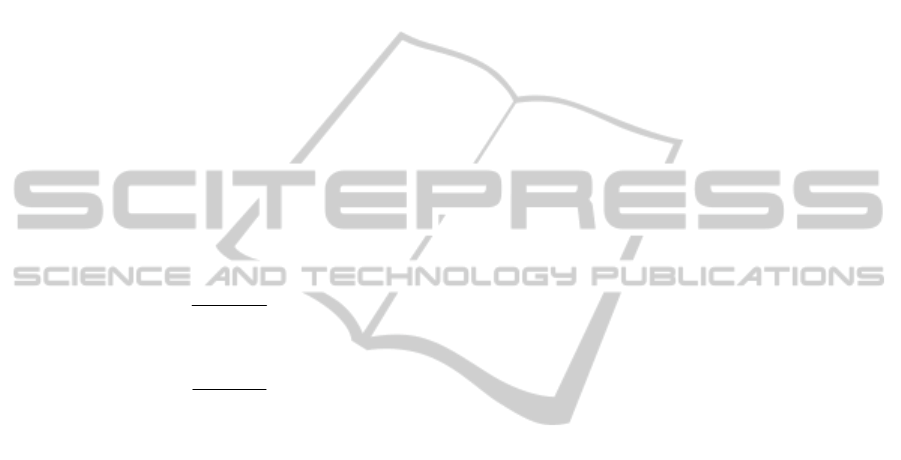
weights). The device was used on the waist. The
four volunteers were invited to act as natural as
possible in all situations. Fall simulations were
performed with the help of a protection mattress so
that the volunteers could reproduce a fall without
any constrain of injury.
Each individual performed 3 tests for each
situation. The obtained results evidence a very good
overall performance of the device. For a total of 180
tests only 9 tests were misclassified, which
represents a 95% of correctly classified cases. More
important, no false negatives were found, meaning
that the device is able to detect all the fall events
tested. The failed cases can be explained by the
acceleration and angular velocities profiles. The
sitting down, jumping and to go on all fours
movements could have similar profiles to a fall.
Following the proposal of Noury et al. (2008) for
the classification and evaluation of fall detection
systems, Sensitivity and Specificity criteria were
used to assess the performance of the proposed
scheme.
TP
Sensitivity
TP FN
=
+
(13)
TN
Specificity
TN FP
=
+
(14)
where TP, FN, TN and FP are the True Positive,
False Negative, True Negative and False Positive
cases, respectively.
The obtained Sensitivity and Specificity
coefficients were 100% and 91.67%, respectively.
5 CONCLUSIONS
In this work a fall detection device based on a neural
network was proposed. This approach revealed to be
very effective in identifying falls, presenting a
Sensitivity coefficient of 100%. It was not so
efficient classifying non-fall events, presenting some
false positives cases.
Considering that not all the tests for the same
type of motion were wrongly classified, this can
indicate an underfitting training. Increasing the
number of training examples could help to improve
the classifier performance in this point.
REFERENCES
Benocci, M., Tacconi, C., Farella, E., Benini, L., Chiari,
L., Vanzago, L., 2010. Accelerometer-based fall detection
using optimized ZigBee data streaming.
Microelectronics Journal, 41(11), 703-710
Bianchi, F., Redmond, S. J., Narayanan, M. R., Cerutti, S.,
Lovell, N.H., 2010. Barometric Pressure and Triaxial
Accelerometry-Based Falls Event Detection. Ieee
Transactions on Neural Systems and Rehabilitation
Engineering, 18(6), 619-627.
Bourke, A. K., O'Brien, J. V., Lyons, G. M., 2007a.
Evaluation of a threshold-based tri-axial accelerometer
fall detection algorithm. Gait & Posture, 26(2),
194-199.
Bourke, A. K., van de Ven, P., Gamble, M., O'Connor, R.,
Murphy, K., Bogan, E., McQuade, E., Finucane, P.,
ÓLaighin, G. Nelson, J., 2010b. Assessment of Waist-
worn Tri-axial Accelerometer Based Fall-detection
Algorithms using Continuous Unsupervised Activities.
2010 Annual International Conference of the Ieee
Engineering in Medicine and Biology Society (Embc),
2782-2785.
CDC. (2011, December 8 2010). Falls among older
Adults: an overview. Retrieved 04-06-2011, from
http://www.cdc.gov/HomeandRecreationalSafety/Falls
/adultfalls.html
Hausdorff, J. M., Rios, D. A., Edelberg, H. K., 2001. Gait
variability and fall risk in community-living older
adults: A 1-year prospective study. Archives of
Physical Medicine and Rehabilitation, 82, 1050-1056.
Hornbrook, M. C., Stevens, V. J., Wingfield, D. J., Hollis,
J. F., Greenlick, M. R., Ory, M. G., 1994. Preventing
Falls among Community-Dwelling Older Persons -
Results from a Randomized Trial. Gerontologist,
34(1), 16-23.
Kangas, M., Konttila, A., Lindgren, P., Winblad, I., &
Jamsa, T., 2008. Comparison of low-complexity fall
detection, algorithms for body attached
accelerometers. Gait & Posture, 28(2), 285-291.
Laguna, M. A., Marques, J. M., Tirado, M. J., Finat, J.,
2010. Fall detection system: a solution based on low
cost sensors. Systems and Information Technologies,
89-94.
Noury, N., Rumeau, P., Bourke, A. K., Olaighin, G.,
Lundy, J. E., 2008. A proposal for the classification
and evaluation of fall detectors. Irbm, 29(6), 340-349.
Nyan, M. N., Tay, F. E. H., Murugasu, E., 2008. A
wearable system for pre-impact fall detection. Journal
of Biomechanics, 41(16), 3475-3481.
Rumelhart, D. E., Hinton, G. E., & Williams, R. J., 1986.
Learning Representations by Back-Propagating Errors.
Nature, 323(6088), 533-536.
BIODEVICES 2012 - International Conference on Biomedical Electronics and Devices
358