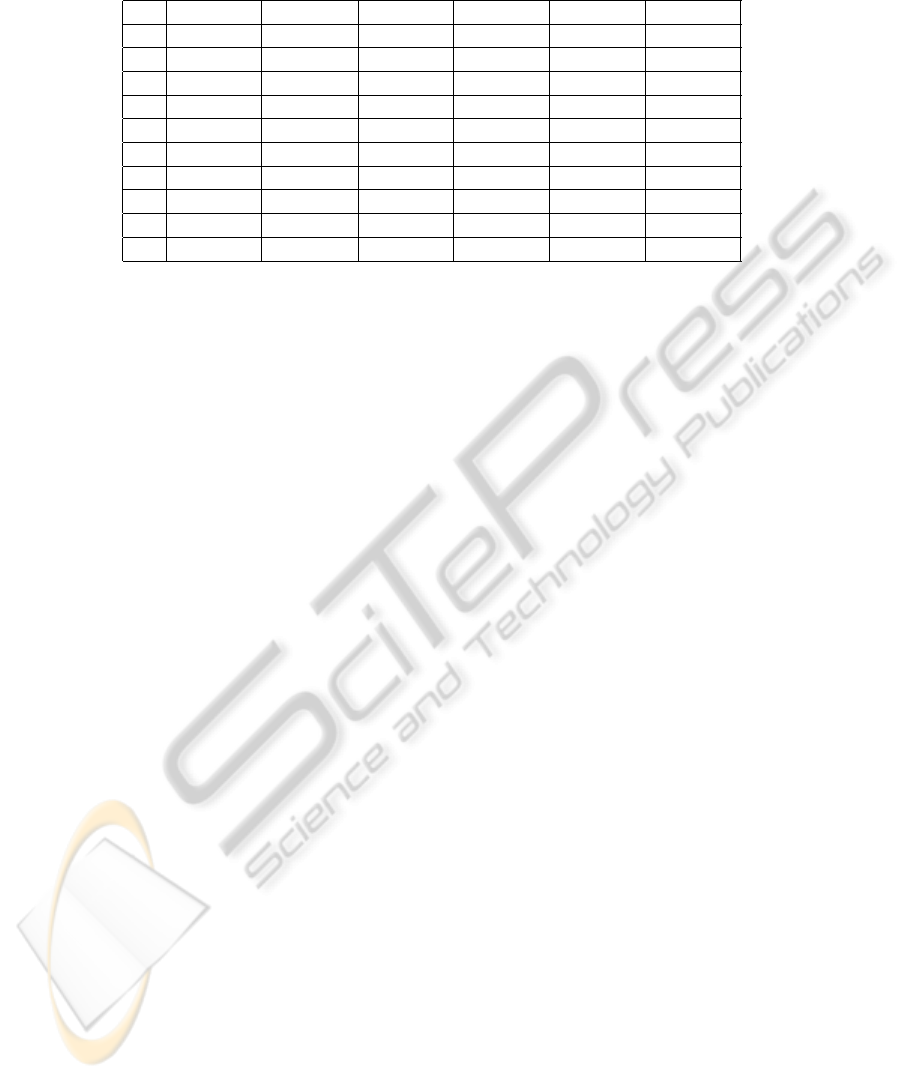
Table 5: Twonorm problems. Average number of clusters in the validated partitions for each of the six validation approaches
considered. The first column shows the dimensionality of the problem.
d AIC BIC D J
B
D J
U
D J
US
D J
UG
2 3.0±0.8 2.0±0.0 2.3±0.8 2.1 ±0.4 2.0±0.0 2.0 ±0.0
4 3.6±0.6 2.0 ±0.0 2.8±0.7 2.6±0.7 2.0±0.0 2.0±0.0
6 3.8±0.4 2.0 ±0.0 3.6±0.5 3.4±0.7 2.0±0.0 2.0±0.0
8 3.8±0.4 2.0 ±0.0 3.9±0.3 3.6±0.5 2.0±0.0 2.0±0.0
10 3.7±0.4 1.1±0.3 4.0 ±0.1 3.8±0.4 2.0±0.0 2.0±0.0
12 3.8±0.4 1.0±0.0 4.0 ±0.0 4.0±0.2 2.0±0.0 2.0±0.0
14 3.8±0.4 1.0±0.0 4.0 ±0.0 4.0±0.1 2.0±0.0 2.0±0.0
16 3.6±0.5 1.0±0.0 4.0 ±0.0 4.0±0.0 2.0±0.0 2.0±0.2
18 3.4±0.5 1.0±0.0 4.0 ±0.0 4.0±0.0 2.1±0.3 2.2±0.4
20 3.2±0.5 1.0±0.0 4.0 ±0.0 4.0±0.0 2.4±0.5 2.2±0.4
overlap problems, which means that the two clusters
are quite well separated. The difficulty in this case
arises from the high dimensionality. The results for
these problems are shown in tables 4 and 5. The
first column in both tables is the dimension. Table 4
shows the number of problems for which each of the
validation methods provides a solution with 2 clus-
ters. Table 5 shows the average number of clusters in
the assessed solutions. All the validation approaches
show a loss of performance when the dimension of the
problems increases, but the D J
US
and D J
UG
indices
are more robust than the others. BIC starts to fail at
d = 10, experimenting a sudden loss of accuracy. For
higher dimensions it tends to select one single cluster.
On the other hand, D J
US
and D J
UG
are very accurate
even for d = 16, and their loss of accuracy for higher
dimensions is more gradual. Finally, the D J
B
, D J
U
and
AIC approaches provide very poor results, and show
a strong tendency to overestimate the number of clus-
ters.
7 CONCLUSIONS
The aim of this paper was to systematically study
the performance of negentropy-based cluster valida-
tion in synthetic problems with increasing dimension-
ality. Negentropy-based indices are quite simple to
compute, as they only need to estimate the probabil-
ities and the log-determinants of the covariance ma-
trices for each cluster. However, the computation of
the log-determinants in regions with small number of
points introduces a strong bias that must be corrected
in order to properly estimate the negentropy index. A
heuristic based on a formal analysis of the bias can be
obtained to alleviate this effect.
In this paper we refined the correction of the ne-
gentropy index proposed in (Lago-Fern´andez et al.,
2011) in order to quantify the confidence levels of
the index value, thus obtaining a new, more formal
heuristic for the validation of clustering partitions.
Then we studied the performance of this and other
negentropy-based validation approaches in problems
with increasing dimensionality, and compared the re-
sults with two well established techniques such as
BIC and AIC. The performance of BIC in problems
where the ratio of the number of points to the di-
mension is high, is quite good. For problems where
there are clusters with a high overlap, it clearly out-
performs the negentropy-based indices. This was ex-
pected since BIC is optimal for Gaussian clusters,
which is the case for the synthetic data considered
here. The AIC criterion seems to produce very bad
results for the set of problems considered, providing a
strong overestimation of the number of clusters in all
the cases. Negentropy-based indices are designed for
crisp clustering, and they seek to detect compact and
well separated clusters. When we consider only prob-
lems where the clusters are not highly overlapped, the
performances of BIC and the negentropy-based index
are quite similar.
In order to test the behavior of the indices as
a function of the dimensionality, we constructed
a clustering benchmark database based on the
twonorm classification problem (Breiman, 1996).
This database is generated using two Gaussian clus-
ters of increasing dimensionality but constant degree
of overlap. The number of points in each cluster is
constant independently of the dimension. Therefore,
the effect of the dimensionality on the performance
of the indices is isolated. As the dimensionality in-
creases, the performance of BIC degrades quickly,
but the performance of the negentropy-based index
is quite stable, finding the correct solution for all the
problems up to d = 16, and experimenting a gradual
degradation for higher dimension.
In conclusion we showed, using the synthetic
database twonorm, that our approach to negentropy-