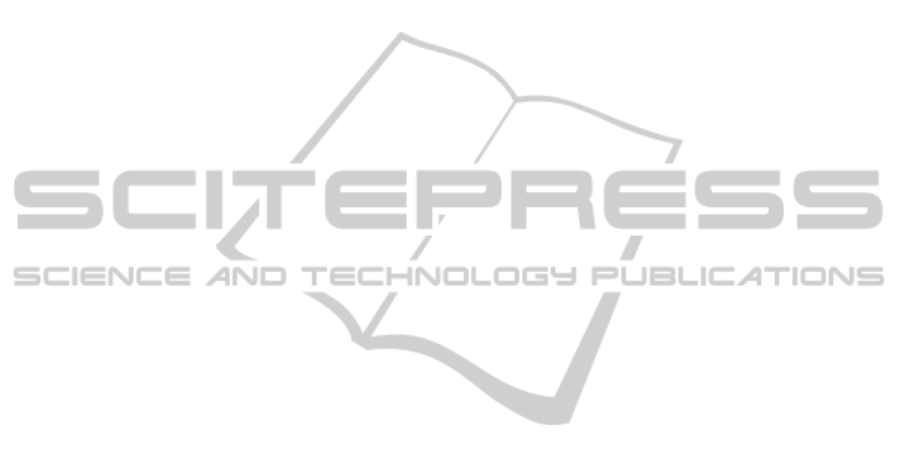
GNARC and A
p
=2.0 for FNARCs) can be achieved
over certain subsets of the uncertainty range of K
using adaptive control. A trade-off is clearly obser-
vable between the number of controllers and the ma-
ximum achievable value of the performance index A
p
.
We were able to meet or exceed the robust perfor-
mance specifications and cover an even larger uncer-
tainty space than that of Martin et al. (1987) with a
smaller number of controllers (5 vs 7).
We have argued that overly simplified physiologi-
cal assumptions may lead to models which fail to de-
scribe the real plant with sufficient accuracy, increa-
sing the risk of degraded performance or even instabi-
lity. In this paper, we have reiterated a few examples
of this and we have reasoned, on the basis of simple
physiological knowledge, that additional uncertainty
in the parameters α and T should be accounted for.
As risk minimisation is a paramount aspect of medical
technology (Malagutti et al., 2011), and perhaps one
of the key reasons behind the lack of success of the
one and only ever commercialised device for this ap-
plication, the IVAC Titrator (IVAC Corporation, San
Diego, CA, USA) (Bequette, 2007), we believe that
improved robustness has an important role to play in
making automatic drug administration systems safer
and more widely acceptable in critical clinical set-
tings. In this context, the use of µ synthesis as a con-
troller design technique represents an improvement
over previous approaches as it offers a mathematical
guarantee of robust closed-loop stability and perfor-
mance over the expected range of uncertainty.
Despite the promising results, the proposed adap-
tation of a robust MMAC architecture to this drug de-
livery problem will require further work before it can
be proposed to a clinical audience. Effective stabilisa-
tion of a patient using the safe controller designs pre-
sented herein relies on the assumption that the correct
patient gain K can be identified and the corresponding
controller placed in the loop at all times. This is a cri-
tical assumption which has not been analysed in detail
here. Indeed, Malagutti et al. (2011) have advocated
caution against scenarios of instability or poor perfor-
mance caused by the insertion of the wrong controller
in the loop as a result of incorrect model identifica-
tion. The issues of reliably matching the plant with
a candidate model and safely placing a suitable con-
troller in the loop (avoiding the insertion of inappro-
priate controllers or placing a safe upper bound on the
time they can remain in the loop for) are critical in
MMAC systems and will be investigated as the next
steps in our research towards a complete and safe con-
trol architecture for this medical application.
A final remark concerns the focus of the control
task on blood pressure, which is only one of a num-
ber of parameters which characterise the state of the
cardiovascular system. In this context, stabilisation
of MAP alone may constitute an insufficient clinical
outcome. Following further consultation with clini-
cal professionals, future work may need to consider
multivariable models which include other key car-
diovascular variables (cardiac output, vascular resis-
tance, atrial pressures, etc.) and other administrable
drugs. Some experimental work exists in the litera-
ture addressing simultaneous control of MAP and car-
diac output (Yu et al., 1992; Rao et al., 2001), howe-
ver, to the authors’ knowledge, MMAC architectures
have not yet been utilised for this purpose. The same
approach presented here has the potential to be ex-
tended, with minor variations, to handle a multivaria-
ble case.
ACKNOWLEDGEMENTS
This work was supported in part by the ARC
Discovery-Projects Grant DP1095290.
REFERENCES
Bailey, J. and Haddad, W. (2005). Drug dosing control
in clinical pharmacology. IEEE Contr Syst Mag,
25(2):35–51.
Batzel, J. J., Kappel, F., Schneditz, D., and Tran, H. T.
(2007). Cardiovascular and Respiratory Systems
Modeling, Analysis, and Control. SIAM.
Bequette, B. W. (2007). Modeling and control of drug infu-
sion in critical care. J Process Control, 17:571–594.
Feakri, S., Athans, M., and Pascoal, A. (2006). Issues,
progress and new results in robust adaptive control.
In Adaptive Control and Signal Processing.
Furutani, E., Araki, M., Sakamoto, T., and Maetani, S.
(1995). Blood pressure control during surgical opera-
tions. IEEE Trans Biomed Eng, 42(10):999–1006.
Gu, D.-W., Petkov, P. H., and Konstantinov, M. M. (2005).
Robust Control Design with Matlab. Springer.
Isaka, S. and Sebald, A. V. (1993). Control strategies for
arterial blood pressure regulation. IEEE Trans Biomed
Eng, 40:353363.
Malagutti, N., Dehghani, A., and Kennedy, R. A. (2011).
Safety issues in adaptive control systems for auto-
matic administration of vasoactive drugs. In roceed-
ings of 18th IFAC World Congress, Milan, Italy.
Martin, J. F., Schneider, A. M., and Smith, N. T. (1987).
Multiple-model adaptive control of blood pressure
using sodium nitroprusside. IEEE Trans Biomed Eng,
34(8):603611.
Rao, R. R., Palerm, C. C., Aufderheide, B., and Bequette,
B. W. (2001). Automated regulation of hemodynamic
variables. IEEE Eng Med Biol Mag, 20(1):24–38.
IMPROVED ROBUST PERFORMANCE IN A SYSTEM FOR AUTOMATIC ADMINISTRATION OF A
VASOACTIVE DRUG
289