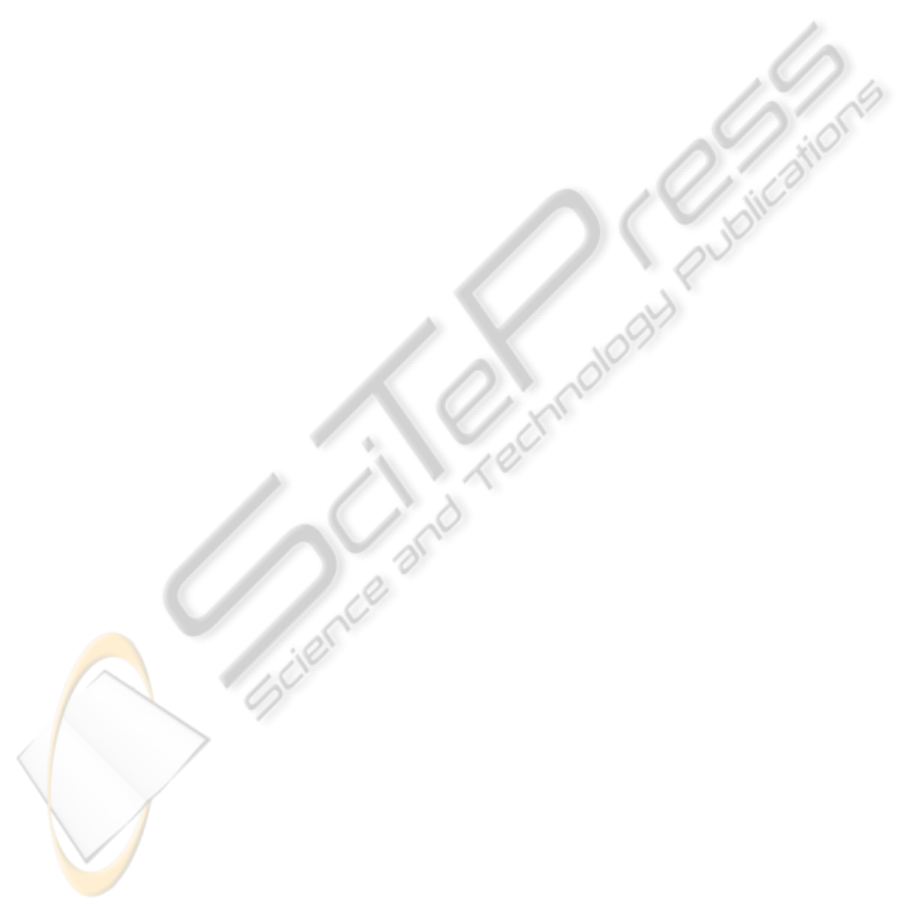
ACKNOWLEDGEMENTS
The authors would like to acknowledge support from
ARTICA (Alianza Regional para las TICs Aplicadas)
and the Cocreation project which is supported by Uni-
versidad de Antioquia, Universidad Nacional Sede
Medellin, Universidad Pontificia Bolivariana, Univer-
sidad EAFIT, Universidad de Medelln and UNE.
REFERENCES
Algergawy, A., Schallehn, E., and Saake, G. (2008). A
schema matching-based approach to xml schema clus-
tering. In Proceedings of the 10th International Con-
ference on Information Integration and Web-based
Applications & Services, pages 131–136, New York,
NY, USA. ACM.
Bae, E. and Bailey, J. (2006). Coala: A novel approach for
the extraction of an alternate clustering of high quality
and high dissimilarity. In IEEE International Confer-
ence on Data Mining, pages 53–62.
Caruana, R., Elhawary, M., Nguyen, N., and Smith, C.
(2006). Meta clustering. In Proceedings of the Sixth
International Conference on Data Mining, ICDM 06,
pages 107–118, Washington, DC, USA. IEEE Com-
puter Society.
Carvalho, F. (2007). Fuzzy c-means clustering methods for
symbolic interval data. Pattern Recognition Letters,
28(4):423–437.
Choubassi, M. E., Nefian, A., Kozintsev, I., Bouguet, J., and
Wu, Y. (2007). Web image clustering. In IEEE Inter-
national Conference on Acoustics, Speech and Signal
Processing, volume 4, pages 15–20.
Davidson, I. and Qi, Z. (2008). Finding alternative clus-
terings using constraints. In Proceedings of the 2008
Eighth IEEE International Conference on Data Min-
ing, pages 773–778, Washington, DC, USA. IEEE
Computer Society.
Dhillon, I. S. and Modha, D. S. (2001). Concept decom-
positions for large sparse text data using clustering.
Machine Learning, 42(1-2):143–175.
Dimitrova, N. and Golshani, F. (1995). Motion recovery
for video content classification. ACM Trans. Inf. Syst.,
13:408–439.
Dy, J. and Brodley, C. (2004). Feature selection for unsu-
pervised learning. Journal of Machine Learning Re-
search, 5:845–889.
Feng, Z., Bao, J., and Shen, J. (2010). Dynamic and adap-
tive self organizing maps applied to high dimensional
large scale text clustering. In Software Engineering
and Service Sciences ICSESS, pages 348–351. IEEE
International Conference.
Filippone, M., Camastra, F., Masulli, F., and Rovetta, S.
(2008). A survey of kernel and spectral methods for
clustering. Pattern Recognition, 41:176–190.
Forestier, G., Wemmert, C., and Gancarski, P. (2010). To-
wards conflict resolution in collaborative clustering.
In Intelligent Systems (IS), 2010 5th IEEE Interna-
tional Conference, pages 361–366.
Forestier, G., Wemmert, C., and Ganc¸arski, P. (2008). Mul-
tisource images analysis using collaborative cluster-
ing. EURASIP J. Adv. Signal Process, 2008:133:1–
133:11.
Francois, O., Ancelet, S., and Guillot, G. (2006). Bayesian
clustering using hidden markov random fields in spa-
tial population genetics. Genetics, 174:805–816.
Fred, A. and Jain, A. (2005). Combining multiple clus-
terings using evidence accumulation. IEEE Transac-
tions on Pattern Analysis and Machine Intelligence,
27(6):835–850.
Gancarski, P. and Wemmert, C. (2007). Collaborative multi-
step mono-level multi-strategy classification. Multi-
media Tools Appl., 35:1–27.
Halkidi, M., Batistakis, Y., and Vazirgiannis, M. (2002).
Cluster validity methods: part i. ACM SIGMOD
Record, 31(2).
Hashimoto, W., Nakamura, T., and Miyamoto, S. (2009).
Comparison and evaluation of different cluster valid-
ity measures including their kernelization. Journal of
Advanced Computational Intelligence, 13(3).
Hofmann, T., Scholkopf, B., and Smola, A. (2008). Kernel
methods in machine learning. The Annals of Statistcs,
36(3):1171–1220.
Hoi, S. and Lyu, M. (2008). A multimodal and multilevel
ranking scheme for large-scale video retrieval. Multi-
media, IEEE Transactions on, 10:607–619.
Hubert, L. and Arabie, P. (1985). Comparing partitions.
Journal of Classification, 2(1):193–218.
Hunter, J. and Choudhury, S. (2003). Implementing preser-
vation strategies for complex multimedia objects. In
Seventh European Conference on Research and Ad-
vanced Technology for Digital Libraries, ECDL 2003,
pages 473–486. Springer.
Jain, A., Murty, M., and Flynn, P. J. (1999). Data clustering:
a review. ACM Computing Surveys, 31(3):264–323.
Jain, A. K. (2010). Data clustering: 50 years beyond k-
means. Pattern Recognition Letters, 31(8):651–666.
Jiamthapthaksin, R., Eick, C. F., and Rinsurongkawong, V.
(2009). An architecture and algorithms for multi-run
clustering. In Computational Intelligence Symposium
on Data Mining CIDM 09, pages 306–313.
Kriegel, H.-P., Kunath, P., Pryakhin, A., and Schubert,
M. (2008). Distribution-based similarity for multi-
represented multimedia objects. In Proceedings of the
14th international conference on Advances in multi-
media modeling, MMM 08, pages 155–164, Berlin,
Heidelberg. Springer-Verlag.
Law, M. H. C., Topchy, A. P., and Jain, A. K. (2004). Mul-
tiobjective data clustering. In Proceedings of the 2004
IEEE computer society conference on Computer vi-
sion and pattern recognition, CVPR 04, pages 424–
430, Washington, DC, USA. IEEE Computer Society.
Liu, T., Liu, S., Chen, Z., and Ma, W. (2003). An evaluation
on feature selection for text clustering. In Proceed-
ings of the 20th International Conference on Machine
Learning, pages 448–495. AAAI Press.
ICPRAM 2012 - International Conference on Pattern Recognition Applications and Methods
142