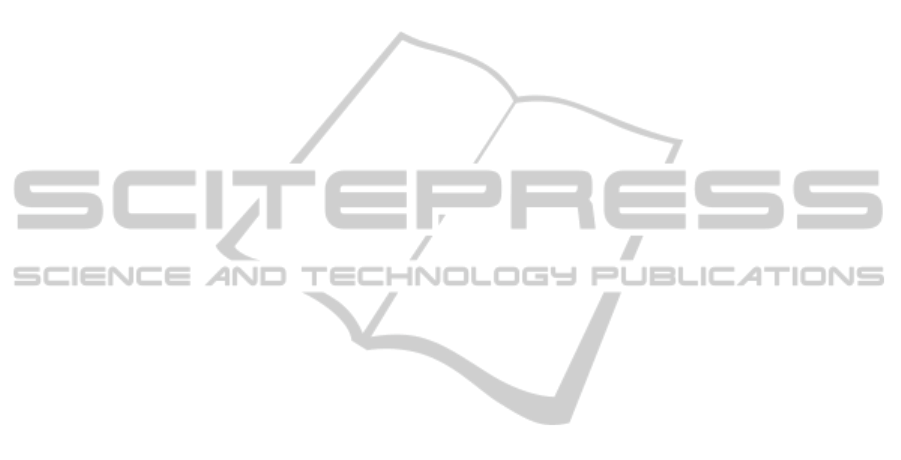
tion Trajectories, whereas (Abdelkader et al., 2002)
utilizes self-similarity plots resulting from periodic
motion. Unfortunately the research work of Weinland
et al. and Rao et al. do not analyze rotation invari-
ance satisfactorily. The approach of Abdelkader et al.
achieves high accuracies for a wide range of different
camera angles. For a 1-nearest neighbor classifier and
using normalized cross correlation of foreground im-
ages 7 out of 8 angles have an accuracy above 0.60.
A comparison of this work to our work is not possible
due to the fact, that Abdelkader et al. consider only
one class for their classification process.
He and Debrunner compute Hu moments for re-
gions with motion in each frame (He and Debrun-
ner, 2000). Afterwards their system counts the num-
ber of frames until a Hu Moment repeats and define
this number as the motion’s frequency. Hu moments
are invariant for translation, planar rotation, reflection
and scaling. Here the periodic trajectory of an object
cannot be ascertained. A strongly related work to our
approach is given by (Meng et al., 2006). This pa-
per depicts a time shift invariant technique for repeat-
ing movements, but it depends on the MLD-System
(Moving Light Displays).
If we compare our approach to other approaches we
find out, that other approaches do not comprise all dif-
ferent types of invariance as entirely as our method.
8 CONCLUSIONS
In this paper we have shown a scale, view, transla-
tion, reflection and time shift invariant approach for
classifying video sequences. The classification pro-
cess is performed by AAFIs, which represent aver-
age amplitudes of frequency intervals. Frequency
spectra are figured by transforming spatio-temporal
image moment trajectories via FFT. In addition a
novel radius based classifier (RBC) was proposed,
which improved the performance of the system. The
stated accuracies in the experimental phase result
from both selected features and RBC. Other clas-
sifiers (k-nearest neighbor, bayes, average link) we
tested do not achieve same accuracy levels as RBC.
The system’s robustness against different camera
properties (zoom, angle, slide, pan, tilt) is useful for
classifying clips from varying sources. Furthermore it
stays an open issue to adapt and analyze the presented
approach for real time action recognition.
REFERENCES
Abdelkader, C.B., Cutler, R., and Davis, L. (2002). Motion-
based recognition of people in eigengait space. In
Proceedings of the Fifth IEEE Int. Conf. on Automatic
Face and Gesture Recognition, pages 267–277.
Ayyildiz, K. and Conrad, S. (2011). Video classification
by main frequencies of repeating movements. In 12th
International Workshop on Image Analysis for Multi-
media Interactive Services (WIAMIS 2011).
Bashir, F., Khokhar, A., and Schonfeld, D. (2006). View-
invariant motion trajectory-based activity classifica-
tion and recognition. Multimedia Systems, 12(1):45–
54.
Bobick, A. F., Davis, J. W., Society, I. C., and Society, I. C.
(2001). The recognition of human movement using
temporal templates. IEEE Transactions on Pattern
Analysis and Machine Intelligence, 23:257–267.
Chen, X., Schonfeld, D., and Khokhar, A. (2008). Ro-
bust null space representation and sampling for view-
invariant motion trajectory analysis. Computer Vi-
sion and Pattern Recognition, IEEE Computer Society
Conference on, 0:1–6.
Fanti, C., Zelnik-manor, L., and Perona, P. (2005). Hybrid
models for human motion recognition. In IEEE Inter-
national Conf. on Computer Vision, pages 1166–1173.
He, Q. and Debrunner, C. (2000). Individual recognition
from periodic activity using hidden markov models.
In Workshop on Human Motion, pages 47–52.
Lienhart, R. (1996). Indexing and retrieval of digital video
sequences based on automatic text recognition. In
Fourth ACM int. conf. on multimedia, pages 419–420.
Meng, Q., Li, B., and Holstein, H. (2006). Recognition of
human periodic movements from unstructured infor-
mation using a motion-based frequency domain ap-
proach. IVC, pages 795–809.
Niebles, J. C. and Fei-fei, L. (2007). A hierarchical model
of shape and appearance for human action classifica-
tion. In IEEE Computer Society Conference on Com-
puter Vision and Pattern Recognition, pages 1–8.
Patel, N. and Sethi, I. (1996). Audio characterization for
video indexing. In SPIE on Storage and Retrieval for
Still Image and Video Databases, pages 373–384.
Pei, S. and Chen, F. (2003). Semantic scenes detection and
classification in sports videos. In Conf. on Computer
Vision, Graphics and Image Proc., pages 210–217.
Rao, C., Gritai, A., Shah, M., and Syeda-Mahmood, T.
(2003). View-invariant alignment and matching of
video sequences. IEEE International Conference on
Computer Vision, pages 939–945.
Weinland, D., Ronfard, R., and Boyer, E. (2006). Free
viewpoint action recognition using motion history vol-
umes. In Computer vision and image understanding,
pages 249–257.
Wong, W., Siu, W., and Lam, K. (1995). Generation of
moment invariants and their uses for character recog-
nition. Pattern Recognition Letters, 16:115–123.
Zhang, R., Vogler, C., and Metaxas, D. (2004). Human gait
recognition. In Proc. of the 2004 Conf. on Computer
Vision and Pattern Recognition, pages 18–27.
VISAPP 2012 - International Conference on Computer Vision Theory and Applications
666