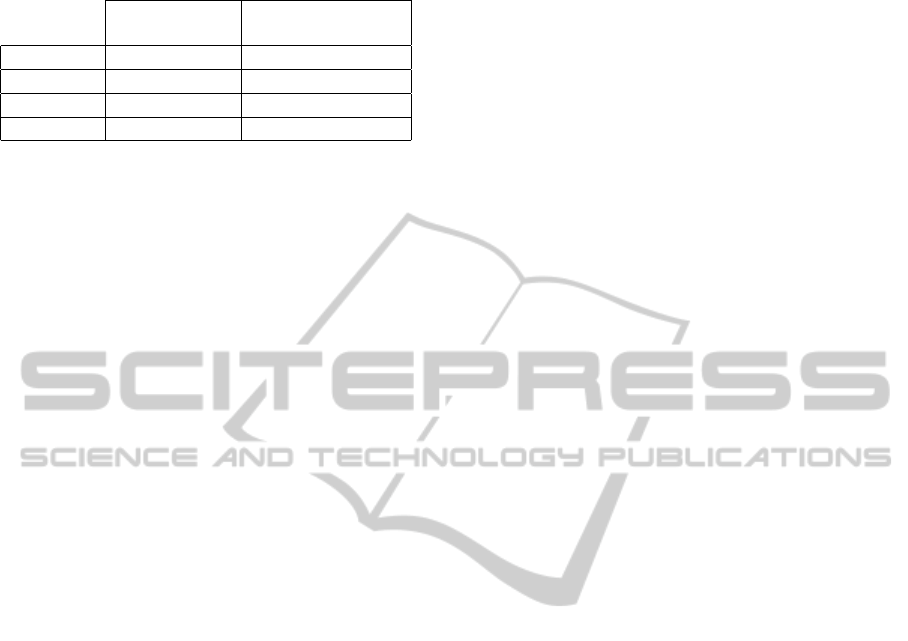
Table 1: Summary of detection results.
Detection rate Computation
(%) time per image (s)
DT 46.2 0.249
DT + GW 52.02 0.546
DT + CN 87.83 29.01
LCCS 97.35 0.093
DT = dynamic thresholding
DT+GW = dynamic threshold with Gray-World color constancy
DT+CN = dynamic threshold with comprehensive normalization
color constancy
LCCS = proposed method in log-chromaticity color space.
increasing the computation time by a factor two.
The comprehensive normalization color con-
stancy algorithm gives good results with a correct de-
tection rate of more than 87%. However, the pro-
cessing time of comprehensive normalization is very
high, which limits its use in real-time applications.
On the contrary, the proposed method based on log-
chromaticity color space achieves the best detection
results in the least computation time as shown in Ta-
ble 1. Note that the given computation time are obtain
with non optimized MATLAB codes using a 2.4 GHz
CPU and images of size 640 x 480.
The proposed method fails to correctly detect road
signs when the image is acquired in complete dark
condition, the road sign being only illuminated by the
headlights of the moving car. In such a case, all meth-
ods fail to detect the road signs.
5 CONCLUSIONS
In this paper, an efficient and robust road signs seg-
mentation method based on color information is pro-
posed. The method is based on finding boundaries
of clusters formed by distinctive road signs color in
the log-chromaticity color space. Based on invari-
ance properties of this color space, we propose an ef-
ficient segmentation method and experiments with a
large dataset show that the proposed method is par-
ticularly robust against severe illumination changes
for images taken under various conditions. Compar-
ison with other approaches based on color constancy
algorithms show that the proposed method achieves
the best segmentation results while requiring the least
computation time. The simplicity and the robustness
of the method make it suitable for real-time appli-
cations such as on-board drivers assistance systems.
Our future work include using the proposed method
as a first step of a road signs recognition system.
REFERENCES
Barnard, K., Martin, L., Coath, A., and Funt, B. (2002). A
Comparison of Computational Color Constancy Algo-
rithms - Part II: Experiments with Image Data. IEEE
Transactions on Image Processing, 11(9):985–996.
Bascon, S. M., Arroyo, S. L., Jimenez, P. G., Moreno,
H. G., and Ferreras, F. L. (2007). Road Sign Detection
and Recognition Based on Support Vector Machines.
IEEE Transactions on Intelligent Transportation Sys-
tem, 8(2):264–278.
Benallal, M. and Meunier, J. (2003). Real-time Color Seg-
mentation of Road Signs. In Proceedings of Canadian
Conference on Electrical and Computer Engineering
(IEEE CCECE 03), pages 1823–1826.
Ebner, M. (2004). Color Constancy Using Local Color
Shifts. In Proceedings of the European Conference
on Computer Vision, pages 276–287.
Escalera, A., Armingol, J. M., and Mata, M. (2003). Traffic
Sign Recognition and Analysis for Intelligent Vehi-
cles. Image and Vision Computing, 21:247–258.
Fang, C. Y., Chen, S. W., and Fuh, C. S. (2003). Road
Sign Detection and Tracking. IEEE Transactions on
Vehicular Technology, 52(5):1329–1341.
Finlayson, G., Drew, M., and Lu, C. (2004). Intrinsic Im-
ages by Entropy Minimization. In Proceedings of
the European Conference on Computer Vision, pages
582–595.
Finlayson, G. D., Schiele, B., and Crowley, J. L. (1998).
Comprehensive Colour Image Normalization . In Pro-
ceedings of the European Conference on Computer Vi-
sion, pages 475–490.
Fleyeh, H. (2004). Color Detection and Segmentation for
Road and Traffic Signs. In Proceedings of the 2004
IEEE Conference on Cybernetics and Intelligent Sys-
tems, pages 808–813.
Fleyeh, H. (2005). Traffic Signs Color Detection and Seg-
mentation in Poor Light Conditions. In MV A2005
IAPR Conference on Machine Vision Applications,
pages 306–309.
Gao, X., Podladchikova, L., Shaposhnikov, D., Hong, K.,
and Shevtsova, N. (2006). Recognition of traffic signs
based on their colour and shape features extracted us-
ing human vision models. Journal of Visual Commu-
nication and Image Representation, 17(4):675–685.
Le, T. T., Tran, S. T., Mita, S., and Nguyen, T. D. (2010).
Real Time Traffic Sign Detection Using Color and
Shape-Based Features. In Proceedings of Intelligent
Information and Database Systems, Second Interna-
tional Conference, pages 268–278.
Paclik, P., Novovicova, J., Pudil, P., and Somol, P. (2000).
Road Sign Classiffication Using Laplace Kernel Clas-
sifier. Pattern Recognition Letters, 21:1165–1173.
Zakir, U., Zafar, I., and Edirisinghe, E. A. (2011). Road
Sign Detection and Recognition by Using Local En-
ergy based Shape Histogram (LESH). International
Journal of Image Processing, 4(6):567–583.
VISAPP 2012 - International Conference on Computer Vision Theory and Applications
310