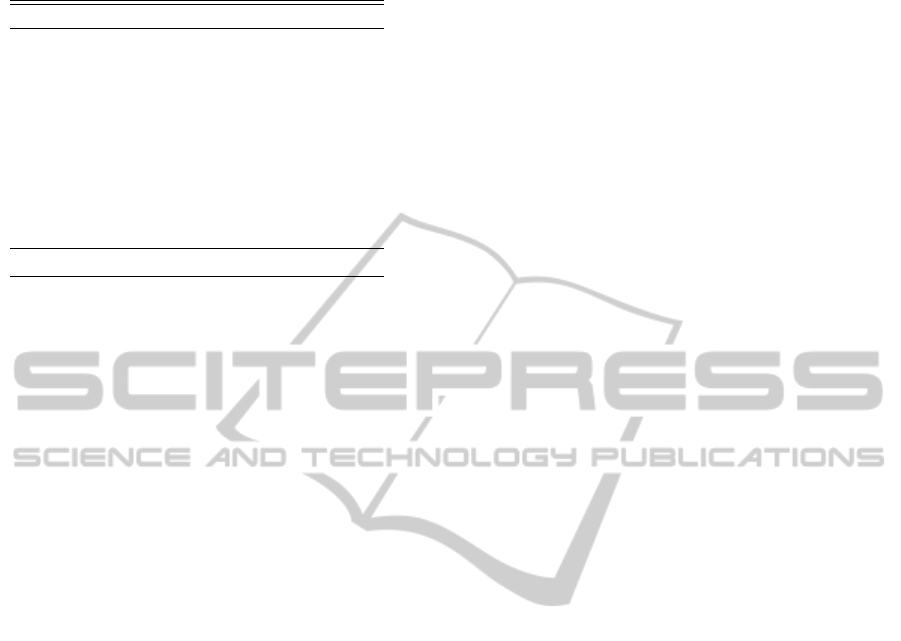
Table 6: 2% Salt&Pepper noise; Wavelet invariants; N=300;
M=5; V=10%; T=15%; P=75%.
Gesture Correct Opposite Total Wrong
R. Rock 27.1 35.7 62.8 22.5
R. Paper 41.1 0.0 41.1 48.2
R. OK 24.8 5.1 29.8 17.8
R. Stop 31.0 17.5 48.4 25.4
R. Pointing 35.3 5.8 41.0 31.0
L. Rock 30.6 30.2 60.8 20.0
L. Paper 43.0 2.0 45.0 28.2
L. OK 50.0 8.3 58.3 9.9
L. Stop 40.8 33.6 74.3 18.4
L. Pointing 12.2 6.5 18.7 17.3
Noise – – – 22.3
Average 33.6 14.4 48.0 23.6
5 CONCLUSIONS
The goal of this paper was to investigate whether Hu’s
moment invariants, despite their proven mathematical
imprecision, are still a viable option for non-expert
users and small scale applications, thanks to their sim-
plicity. To address this question, I compared their
performance with the more recent set of wavelet in-
variants, in a severe low-resolution environment that
emulates a possible amateurish hardware scenario.
The results, albeit few in quantity, show how Hu’s
invariants perform greatly over still images, definitely
better than the more advanced wavelet set. I have
then tried to have Hu’s and wavelet sets detect trans-
lating gestures: results were, obviously, much worse,
but still in the 40–60% range, with Hu’s invariants
performing generally better than the wavelet’s also in
this case. Finally the test was conducted over noisy
images. In both cases there is a worsening of the re-
sults of about absolute 30%, but Hu’s invariants are
definitely better in this case also. The reason for
this is dual: firstly, the low resolution leads to prob-
lems in the discretization of the radial integral steps
for the wavelet invariants calculation, and secondly
the wavelet set is much more sensitive to the shape
changes, intrinsic in this application, than Hu’s set.
In conclusion, it is my opinion that Hu’s invari-
ants have proven their robustness under difficult con-
ditions, and therefore they are still a great choice, es-
pecially in order to allow amateurs of the field to try
and implement their ideas. Nevertheless, this doesn’t
mean that one must think of them as the panacea of
all shape recognition problems: in other applications
other approaches have proven their superiority, and
CV libraries maintainers should indeed start imple-
menting the newer tools in their collections, leaving
the responsibility of picking the right tool for each
problem to the developer.
ACKNOWLEDGEMENTS
The author would like to thank Prof. Koichi Shinoda
at Tokyo Institute of Technology for his suggestions,
and all Shinoda Lab’s members for their cooperation.
REFERENCES
Ahuja, N., Lertrattanapanich, S., and Bose, N. (2005).
Properties determining choice of mother wavelet. Vi-
sion, Image and Signal Processing, IEEE Proceed-
ings, 152(5):659–664.
Flusser, J. (2000). On the independence of rotation moment
invariants. Pattern Recognition, 33(9):1405–1410.
Flusser, J. and Suk, T. (1994). Affine moment invariants: a
new tool for character recognition. Pattern Recogni-
tion Letters, 15(4):433–464.
Hu, M.-K. (1962). Visual pattern recognition by moment
invariants. In Information Theory, IRE Transactions
on, volume 8, pages 179–187. IEEE Computer Press.
Imaging, M. (2011). Mesa imaging product description,
2011.
Li, Y. (1992). Reforming the theory of invariant moments
for pattern recognition. Pattern Recognition, 25:723–
730.
Reiss, T. (1991). The revised fundamental theorem of mo-
ment invariants. IEEE Trans. Pattern Anal. Mach. In-
tell, 13(8):830–834.
Schmidt, D., Ki Chong, M., and Gellersen, H. (2010).
Handsdown: hand–contour–based user identification
for interactive surfaces. NordiCHI 2010 Proceedings
of the 6th Nordic Conference on Human-Computer In-
teraction: Extending Boundaries, pages 432–441.
Shen, D. (1999). Discriminative wavelet shape descriptors
for recognition of 2-d patterns. Pattern Recognition,
32:151–165.
Soutschek, S., Penne, J., Hornegger, J., and Kornhuber, J.
(2008). 3-d gesture-based scene navigation in medi-
cal imaging applications using time-of-flight cameras.
Computer Vision and Pattern Recognition Workshops,
2008. CVPRW ’08. IEEE Computer Society Confer-
ence on, pages 1–6.
Unser, M., Aldroubi, A., and Eden, M. (1992). On the
asymptotic convergence of b-spline wavelets to gabor
functions. IEEE Trans. Inform. Theory, 38(2):864–
872.
WillowGarage (2011). Opencv official documentation.
Wong, R. Y. and Hall, E. L. (1978). Scene matching with
invariant moments. Computer Graphics and Image
Processing, 8(1):16–24.
Wong, W., Siu, W., and Lam, K. (1995). Generation of
moment invariants and their uses for character recog-
nition. Pattern Recognition Letters, 16(2):115–123.
Zhang, F., Liu, S.-q., Wang, D.-b., and Guan, W. (2009).
Aircraft recognition in infrared image using wavelet
moment invariants. Image and Vision Computing,
27(4):313–318.
VISAPP 2012 - International Conference on Computer Vision Theory and Applications
316