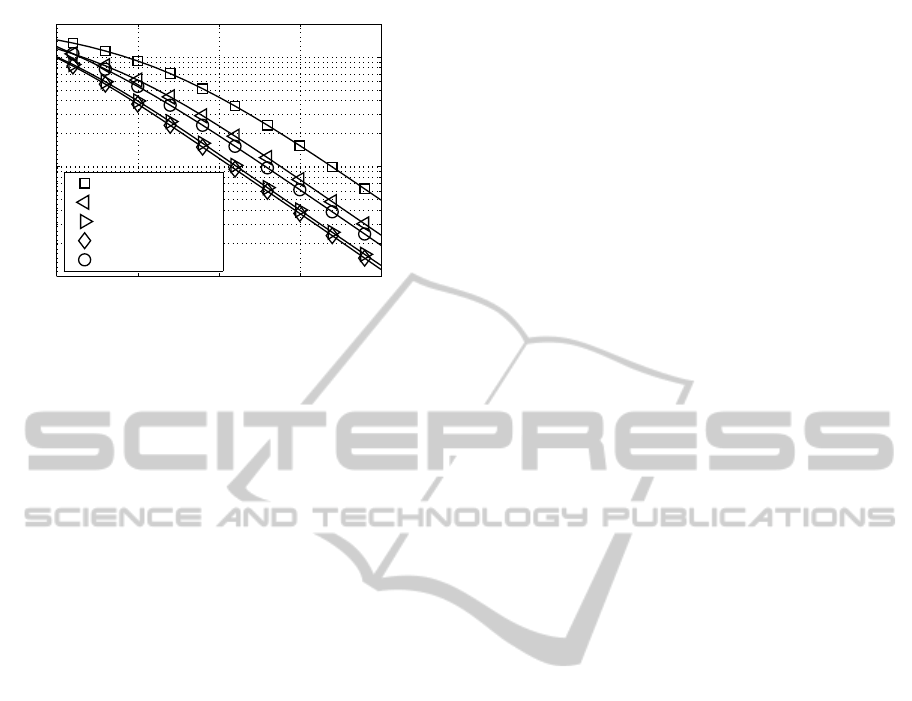
10 15 20 25 30
10
−3
10
−2
10
−1
10 ·log
10
(E
s
/N
0
) (indB) →
bit-error rate →
(256,0,0, 0) QAM
(64,4,0, 0) QAM
(16,16,0, 0) QAM
(16,4,4, 0) QAM
(4,4,4 , 4) QAM
Figure 9: SVD-based user-specific BERs when using the
transmission modes introduced in Table 1 and transmitting
8 bit/s/Hz over uncorrelated frequency non-selective chan-
nels.
5 CONCLUSIONS
This contribution has analyzed and simulated a MU-
MIMO system composed of n
T
transmit and n
R
re-
ceive antennas in conjunction with SVD-assisted sig-
nal processing and taking into account the correlation
effect among antennas both at the transmit and receive
sides. Additionally it was assumed a uniform distri-
bution of the transmit power along the MIMO system
activated layers.
By comparing the results obtained from computer
simulations it can be concluded that in the presence of
correlation among antennas, in the considered non-
linear spatial distribution (square spacing in the ex-
ample) the bit error rate increases for a given fixed
SNR (signal-to-noise-ratio), taking as reference the
case in which no correlation is present into consider-
ation. Moreover, that increment in the BER is larger
than the one produced when using an antenna linear
distribution affected by correlation.
Consequently, for a fixed bit-error rate the lin-
ear distribution is able to give better results than the
squared distribution. This is due to the reduction of
the dependency with neighbours antennas as the sep-
arations between them become larger. Additionally
we observe that for reaching the best performance it
is not required that all layers were activated.
REFERENCES
Ahrens, A. and Benavente-Peces, C. (2010). Modulation-
Mode and Power Assignment for SVD-assisted and
Iteratively Detected Downlink Multiuser MIMO Sys-
tems. In International Conference on Wireless Infor-
mation Networks and Systems (WINSYS), pages 107–
114, Athens (Greece).
Ahrens, A. and Benavente-Peces, C. (2011). Modulation-
Mode and Power Assignment for SVD-assisted
Downlink Multiuser MIMO Systems. In International
Conference on Pervasive and Embedded Computing
and Communication Systems (PECCS), pages 333–
338, Algarve (Portugal).
Ahrens, A. and Lange, C. (2008). Modulation-Mode and
Power Assignment in SVD-equalized MIMO Sys-
tems. Facta Universitatis (Series Electronics and En-
ergetics), 21(2):167–181.
Asztly, D. (1996). On Antenna Arrays in Mobile Commu-
nication Systems Fast Fading and GSM Base Station
Receiver Algorithms. PhD thesis, Royal Institute of
Technology, Stockholm.
Benavente-Peces, C., Cano-Broncano, F., Aust, S., and
Ahrens, A. (2010). Modulation-Mode Assignment
for SVD-assisted Multiuser MIMO Systems with
Correlations. In 18th International Conference on
Microwave, Radar and Wireless Communications
(MIKON), pages 415–418, Vilnius (Lithuania).
Durgin, G. D. and Rappaport, T. S. (1999). Effects of Multi-
path Angular Spread on the Spatial Cross-Correlation
of Received Voltage Envelopes. In IEEE Vehicu-
lar Technology Conference (VTC), pages 996–1000,
Houston, Texas, USA.
Haykin, S. S. (2002). Adaptive Filter Theory. Prentice Hall,
New Jersey.
K¨uhn, V. (2006). Wireless Communications over MIMO
Channels – Applications to CDMA and Multiple An-
tenna Systems. Wiley, Chichester.
McKay, M. R. and Collings, I. B. (2005). Capacity
and Performance of MIMO-BICM with Zero-Forcing
Receivers. IEEE Transactions on Communications,
53(1):74– 83.
Mueller-Weinfurtner, S. H. (2002). Coding Approaches for
Multiple Antenna Transmission in Fast Fading and
OFDM. IEEE Transactions on Signal Processing,
50(10):2442–2450.
Narasimhan, R. (2003). Spatial Multiplexing with Trans-
mit Antenna and Constellation Selection for Corre-
lated MIMO Fading Channels. IEEE Transactions on
Signal Processing, 51(11):28292838.
Oestges, C. (2006). Validity of the Kronocker Model for
MIMO Correlated Channels. In Vehicular Technology
Conference, volume 6, pages 2818–2822, Melbourne.
Zelst, A. v. and Hammerschmidt, J. S. (2002). A Single Co-
efficient Spatial Correlation Model for Multiple-Input
Multiple-Output (MIMO) Radio Channels. In 27th
General Assembly of the International Union of Ra-
dio Science, Maastricht.
Zheng, L. and Tse, D. N. T. (2003). Diversity and
Multiplexing: A Fundamental Tradeoff in Multiple-
Antenna Channels. IEEE Transactions on Information
Theory, 49(5):1073–1096.
Zhou, Z., Vucetic, B., Dohler, M., and Li, Y. (2005). MIMO
Systems with Adaptive Modulation. IEEE Transac-
tions on Vehicular Technology, 54(5):1073–1096.
ANALYSIS OF MIMO SYSTEMS WITH ANTENNAS CORRELATION WITH LINEAR AND NON-LINEAR
SPATIAL DISTRIBUTION
283