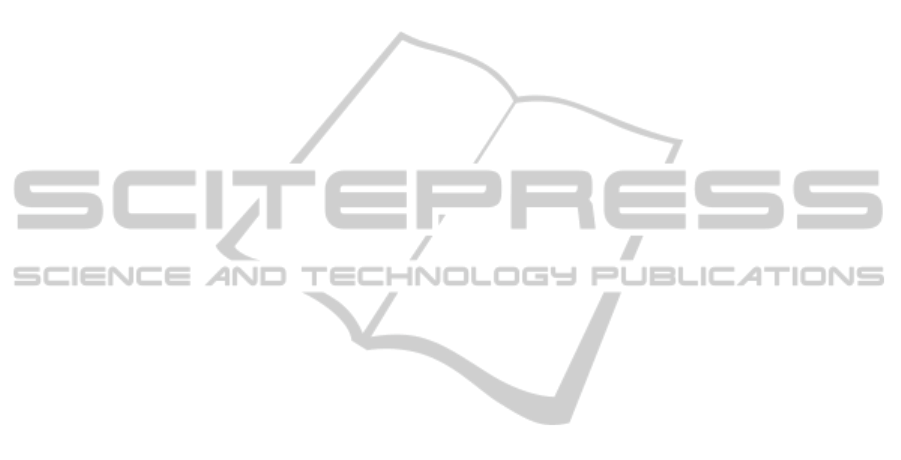
Papageorgiou (2000) showed that compositions
of simple features like Haar-wavelet turned out to
have a great advantage in speed while not suffering
much from precision drop. Viola (2004) introduced
the canonical Cascade Classifier. The problem of
shape-based methods is that they are all aimed for
one specific kind of visual object. If we force the
training samples to contain various kinds of objects,
the output will be a detector poor in both hit rate and
false-alarm rate. If we train a detector for each kind
of objects, the computational resources required
during detecting will be overwhelming.
3 PIPELINE
3.1 Color Space Analysis
Among the many color spaces that can be used for
color space analysis, our system selects HSV
because it is the most coherent with the intuition of
human conception. Rendering a road image in H, S
and V channels, we found that road signs stand out
prominently in H and S channels, but not so much in
V channel. Via setting upper and lower thresholds
on H and S channels, we can approximately pick out
pixels that belong to a road sign. These pixels will
connect with each other to form irregular regions,
which, after certain image processing techniques,
can be used to calculate bounding boxes that most
tightly contain them. These bounding boxes form the
ROIs for the next pipeline stage.
3.2 Contour Analysis
Computer Vision toolset like OpenCV usually
provides some contour analysis tools, which can be
used to extract contours from a binary image, to
match a contour against a template contour, etc.
Contour analysis is based on the binary image output
of color thresholding. Contour matching algorithms
take as input two contours and output a real number
indicating the extent to which they match. A
threshold can be put on this number to rule out
candidates whose contours are too far away from the
wanted contour. Contour algorithms do not use
sliding window, thus is much faster than algorithms
that are performed in a sliding window manner.
3.3 Haar-wavelet Cascade Detector
The design of the cascade detector is the same as
Viola (2004). Because cascade detector is used in a
sliding window manner, it is the biggest time
consumer of the whole system, and the major way of
speeding up is thus to reduce of area of regions this
sliding window is performed on.
3.4 RANSAC Shape-fitting
When we get some points that are believed to be
generated from the edge of certain shape, in
principle we can recover the generating shape (i.e.
its parameters) from the information provided by
these points. Typically we will have much more
points than theoretically needed. RANSAC
(RANdom SAmple Consensus) (Fischler, 1981) is a
method that exploits this redundant information to
improve the precision and stability of shape fitting.
It randomly selects points that are mathematically
sufficient to calculate the shape parameters, and
repeat this procedure certain times to get multiply
sets of calculated parameters. The final values of
parameters are decided by a voting among these
calculated parameter sets.
In addition to gaining the values of shape
parameters, RANSAC can also be used to check our
presumption of shape model. For example, if we
assume the generating shape is a circle, but the
calculated parameter sets differ too much from each
other, i.e. the statistical deviation exceeds some
criteria, then we should forgo our previous
assumption and claim that the shape would not be a
circle. RANSAC is used in this way as a shape
validator in our system.
4 OPTIMIZATION
To stabilize the sizes of ROIs, we implemented a
tracking mechanism. When a sign is detected and
ensured (for example by a consecutive series of
appearances), subsequent detection will be
performed only on its neighborhood, with a
thorough detection every several frames to allow for
new signs.
There are 25 kinds of signs as shown in Figure 2.
Theoretically we should train one cascade detector
for each sign, leading to 25 cascade detectors in total.
The speed requirement cannot afford such an
amount of computing, thus we grouped the signs
into 9 groups and trained a detector for each group,
as shown in Figure 2. Multiple detectors also open
the possibility of parallelization.
For trade-off between hit rate and false-alarm,
we prefer lowering false-alarm rate to lifting hit rate
during parameter adjusting, because a low false-
alarm rate can serve both to precision and speed, and
VISAPP 2012 - International Conference on Computer Vision Theory and Applications
676