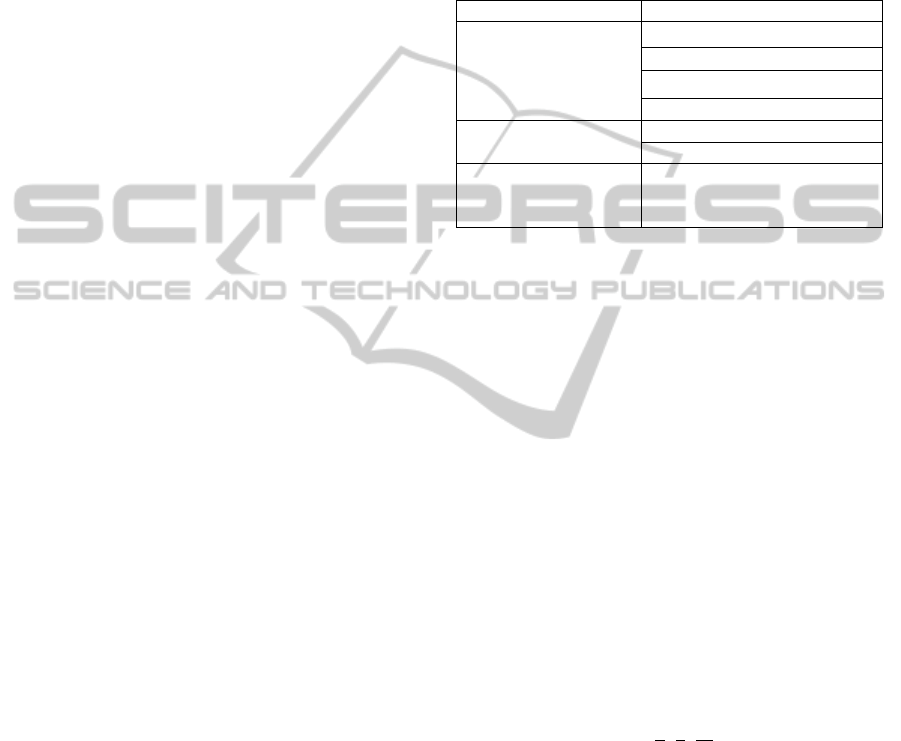
Example-based painterly rendering algorithms
present a new approach for painterly rendering.
Hertzmann et al. present a novel framework called
“image analogy” for transforming photos into
painting-like images by examples (Hertzmann et al.,
2001). In their work, painterly rendering is
formulated as a problem of finding analogy. The
output image is constructed by a block-wised multi-
resolution synthesis. The central idea of this
synthesis technique is to search for the best match
image block from the examples and the input image.
In summary, stroke-based algorithms simulate
the actual painting process. Since brush strokes are
placed one by one on the canvas just as artists do, it
is extremely difficult to derive the reverse process.
Exampled-based painterly rendering algorithms
produce the output by investigating and synthesizing
the pixel level features of examples. However, the
reverse process remains rather difficult because
photographic image details are lost during the
synthesis process. Although various algorithms were
proposed to solve painterly rendering problem, none
of them addressed its reverse process. This is
particularly useful for studies in heritage and
forensic science where photorealistic images of
paintings may be needed. We will introduce reverse
painterly rendering (RPR) algorithm in the next
section.
3 REVERSE PAINTERLY
RENDERING (RPR)
3.1 Problem Formulation
Assume the original photographic image is x(i,j),
and its painted version is y(i,j), the problem of
painterly rendering (particularly with oil painting
effect) can be formulated as:
(
i, j
)
= ∗( ∗((i, j)))+ . (1)
Where n is signal independent noise, which are
caused by adding background textures such as the
texture of canvas to photographic image x. c denotes
a filtering process which incurs signal dependent
noise or distortion to x. For instance, c particularly
causes the distortion of x’s edges and gradient. h is a
2d filter that transforms x’s colour style from
photographic colours to painted colours , * is 2D
convolution.
Therefore, the problem of RPR is to estimate the
original photographic image x through the painterly
rendered image y.
3.2 Solving RPR
3.2.1 Features Extraction
A number of image features are extracted. They can
be categorized as: colour-related, texture-related and
wavelet-related features (Table 1).
Table 1: Extracted image features.
Categories Specific Image Features
Colour- related
Features
Smoothness of colour
Colour palette
Colour saturation
Prevalent colour coverage
Texture-related
Features
Global Gabor filter responses
Local Gabor filter responses
Wavelet-related
Features
First order statistics of first
scale wavelet sub-band
coefficients
In colour-related features, Smoothness of colour
presents the spatial variation of colour in an image
plane. Colour palette accounts for the number of
unique colours in an image. Colour saturation is the
saturation of pixel colours indicated by H channel in
HSV colour space. We extract above three features
using methodology described by (Cutzu et al.,
2005). Prevalent colour coverage presents the
coverage of the most frequently appearing colour in
an image, which was used to distinguish
photographs from graphics on the web in (Athitsos
et al., 1997).
Following the methodology introduced by
(Bianconi and Fernandez, 2007), we extract texture
features using statistics of Gabor filter responses at
five different scales and four different orientations.
Global Gabor filter response is obtained by
averaging Gabor filter responses from all scales and
orientations of a gray scale image. Local Gabor
filter responses are Gabor filter responses from five
different scales (0, 0.2, 0.4, 0.6, 0.8) and four
different orientations (0,
,
,
), respectively.
The four first order statistics (mean, variance,
skewness and kurtosis) of wavelet sub-band
coefficients were used in (Lyu and Farid, 2005) and
(Wang and Moulin, 2006) to distinguish computer
generated photorealistic images from real
photographic images. Similarly, we adopt first order
statistics of first scale wavelet sub-band coefficients
as wavelet-related features.
All the features described above are normalized
by the size of the image so that we obtain a score for
each individual feature. RGB colour images are
converted to greyscale images to extract texture and
GRAPP 2012 - International Conference on Computer Graphics Theory and Applications
242