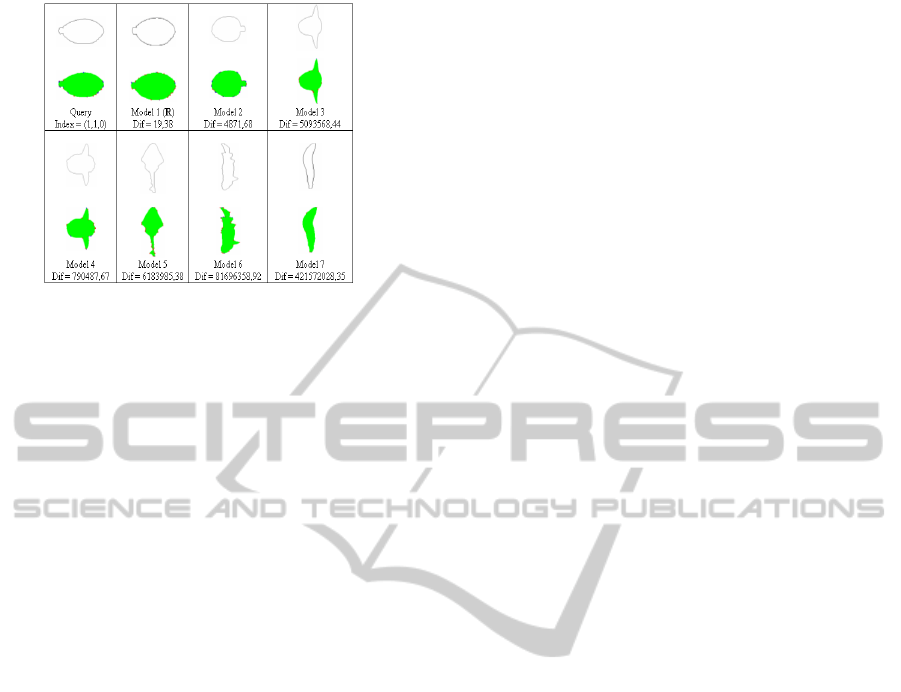
Figure 9: Second example in Mokhtarian database for the
recognition process.
7 CONCLUSIONS
In this paper, we proposed a new method for
silhouettes recognition. Textual description,
smoothing and indexing were previously performed
(Larabi et al, 2003; Aouat and Larabi, 2010; Aouat
and Larabi, 2009).
We have seen the importance of applying
efficient similarity measures to achieve the
recognition process.
Two similarity measures have been proposed:
- The use of parts areas: indeed when two
objects are almost similar, the difference
between their areas is close to zero. The use
of this measure is not sufficient because
different parts may have the same area.
- The computation of geometric quasi-
invariants in order to efficiently compare the
query silhouettes geometry with the models
geometry.
Conducted experiments, performed on two
known databases, showed the method efficiency
and its usefulness to resolve the problem of the
recognition process.
REFERENCES
Alvarado, C., Oltmans, M., Davis, R., 2002. A Framework
for Multi-Domain Sketch Recognition.
In the proc. Of
AAAI Spring Symposium on Sketch Understanding
.
Aouat S, Larabi S., 2009. Coarse Comparison of
Silhouettes using XLWDOS Language. Int. journal
for Computational Vision and Biomechanics
. Volume
2 Number 1.
Aouat, S., Larabi, S., 2010. Matching Descriptors of Noisy
Outline Shapes.
Int. Journal of Image and Graphics.
World Scientific Publisher.
Arandjelovic, R., Zisserman, A., 2010. Efficient image
retrieval for 3D structures. BMVC: 1-11.
Chang, M. C., Kimia, B. B, 2011. Measuring 3D shape
similarity by graph-based matching of the medial
scaffolds. CVIU 115(5): 700-720.
Chetverikov, D., 2003. A Simple and Efficient Algorithm
for Detection of High Curvature Points in Planar
Curves.
10th Int. Conference, CAIP, Netherlands,
August 25-27.
Graham, R. L., 1972. An efficient algorithm for
determining the convex hull of a finite planar set.
Information Processing Letters.
Gros, P., 1994. Using Quasi-Invariant for Automatic
Model Building and Object Recognition: An
Overview,
Proc. of the NSF-ARPA Workshop on
Object Representations in Computer Vision
, New
York, USA.
Keysers, D., Deselaers, T., Breuel, T. M., 2007. Optimal
geometric matching for patch-based object detection.
Electronic Letters on computer vision and image
analysis
, 6(1): 44-54.
Lamiroy, B., Gros, P., 1996. Rapid Object Indexing and
Recognition Using Enhanced Geometric Hashing. 4th
European Conference on Computer Vision
,
Cambridge, England.
Larabi, S., Bouagar, S., Trespaderne, F. M. , Lopez, E. F.,
2003. LWDOS: Language for Writing Descriptors of
Outline Shapes. In the LNCS proceeding of
Scandinavian Conference on Image Analysis
, June 29
- July 02, Gotborg, Sweden.
Latecki, L. J., Lakaemper, R., Wolter, D., 2005. Optimal
partial shape similarity.
Image and vision computing,
23(2): 227-236.
Leibe, B., Schiele, B., 2003. Analyzing Appearance and
Contour Based Methods for Object Categorization.
In
Int. Conference on Computer Vision and Pattern
Recognition
, Madison, Wisconsin.
Ma, T., Latecki, L. J., 2011: From partial matching
through local deformation to robust global shape
similarity for object detection. CVPR: 1441-1448.
Mokhtarian, F., 1995. Silhouette-Based isolated object
recognition through curvature scale space.
IEEE
Trans. on PAMI
, 17(5): 539-544.
Mokhtarian, F., Abbasi, S., Kittler, J., 1996. Efficient and
Robust Retrieval by Shape Content through Curvature
Scale Space.
In proc. of Int. Workshop on Image
Databases and Multimedia Search
, 35-42,
Amsterdam, The Netherlands.
VISAPP 2012 - International Conference on Computer Vision Theory and Applications
400