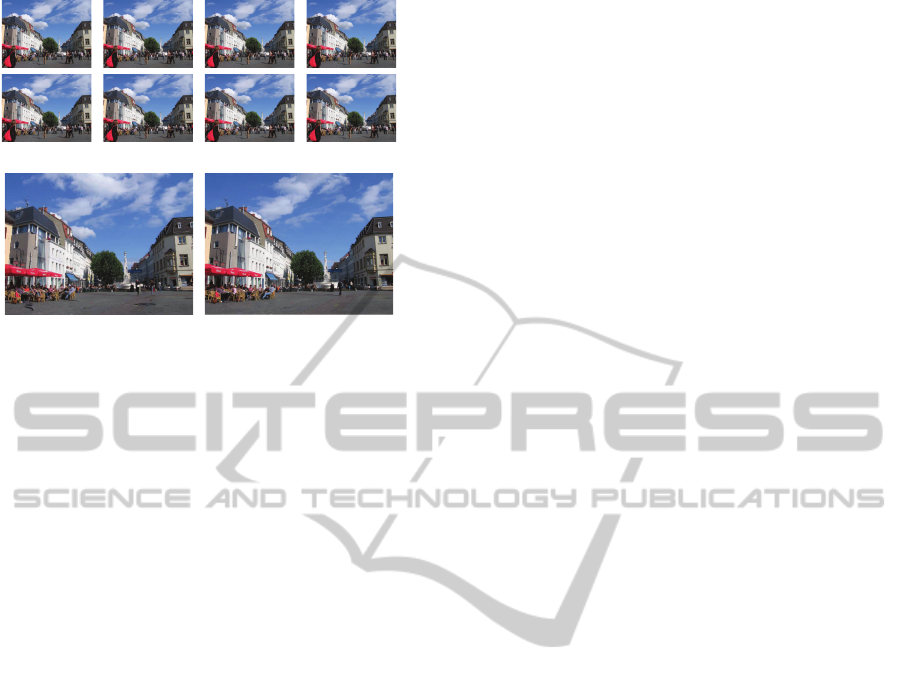
(a) Original images
(b) Our method (c) Agarwala et al.
Figure 5: (a) Images of the Market scene. Estimations us-
ing: (b) Our method, (c) Agarwala et al., 2004.
method of Agarwala et al. requires user interactions
for refinement. For instance, when the estimated
background is still containing foreground objects, the
user must selects these regions which will be replaced
by new ones offered by the system. In some cases,
this interactive step must be repeatedly performed to
achieve an acceptable result. Moreover, Agarwala et
al. apply additional steps as, for instance, gradient-
domain fusion to remove image artifacts. By contrast,
our method is simpler and straightforward.
5 CONCLUSIONS
In this paper, we presented a method to back-
ground estimation containing moving/transient ob-
jects, which uses depth information for such purpose.
Usually, this information is unavailable for monocular
cameras. However, we recovered information about
proximity/distantness of a region in an image, which
is enough for our purpose. This segmentation is used
to found the background by penalizing close regions
in a cost function, which integrates color, motion, and
depth terms. We minimized the cost function by using
a graph cuts approach.
We tested our approach with sequences taken un-
der different conditions (e.g., moving/static camera,
temporal/non-temporal coherence, low/high-quality).
Experimental results shown that our method signifi-
cantly outperforms the median filter approach. Also,
our approach is comparable to state-of-the-art meth-
ods. Unlike Agarwala et al., we perform this task au-
tomatically, without any user intervention.
As further work, we plan to complement our
approach with a gradient-domain fusion to remove
artifacts that are still present in dissimilar images.
Finally, we plan to focus on selecting appropriate
frames to compose the background since many frames
in a sequence do not contribute to the final estimation.
ACKNOWLEDGEMENTS
This work is supported by Spanish MICINN
projects TRA2011-29454-C03-01, TIN2011-29494-
C03-02, Consolider Ingenio 2010: MIPRCV
(CSD200700018), and Universitat Aut
`
onoma de
Barcelona.
REFERENCES
Agarwala, A., Dontcheva, M., Agrawala, M., Drucker, S.,
Colburn, A., Curless, B., Salesin, D., and Cohen,
M. (2004). Interactive Digital Photomontage. ACM
Trans. Graph., 23:294–302.
Black, M. J. and Fleet, D. J. (2000). Probabilistic detection
and tracking of motion boundaries. Int. J. Comput.
Vision, 38:231–245.
Boykov, Y., Veksler, O., and Zabih, R. (2001). Efficient ap-
proximate energy minimization via graph cuts. IEEE
Trans. Pattern Anal. Mach. Intell., 20(12):1222–1239.
Chen, X., Shen, Y., and Yang, Y. H. (2010). Background
Estimation using Graph Cuts and Inpainting. In Proc.
of Graphics Interface Conf., pages 97–103.
Cohen, S. (2005). Background Estimation as a Labeling
Problem. In IEEE Int. Conf. Comput. Vision, pages
1034–1041.
Delong, A., Osokin, A., Isack, H., and Boykov, Y. (2011).
Fast Approximate Energy Minimization with Label
Costs. Int. J. Comput. Vision, pages 1–27.
Goldstein, B. (2010). Sensation and Perception. Wadsworth
Cengage Learning, Belmont, California, USA.
Granados, M., Seidel, H.-P., and Lensch, H. P. A. (2008).
Background Estimation from Non-Time Sequence
Images. In Proc. of Graphics Interface Conf., pages
33–40.
Harville, M., Gordon, G., and Woodfill, J. (2001). Adaptive
Video Background Modeling using Color and Depth.
In Int. Conf. Image Process., volume 3, pages 90–93.
Kwatra, V., Sch
¨
odl, A., Essa, I., Turk, G., and Bobick, A.
(2003). Graphcut Textures: Image and Video Synthe-
sis using Graph Cuts. ACM Trans. Graph., 22:277–
286.
Nedovic, V., Smeulders, A., Redert, A., and Geusebroek,
J. M. (2010). Stages As Models of Scene Geometry.
IEEE Trans. Pattern Anal. Mach. Intell., 32(9):1673–
1687.
Radke, R., Andra, S., Al-Kofahi, O., and Roysam, B.
(2005). Image Change Detection Algorithms: A
Systematic Survey. IEEE Trans. Image Process.,
14(3):294–307.
Saxena, A., Sun, M., and Ng, A. (2009). Make3D: Learning
3D Scene Structure from a Single Still Image. IEEE
Trans. Pattern Anal. Mach. Intell., 31(5):824 –840.
VISAPP 2012 - International Conference on Computer Vision Theory and Applications
328