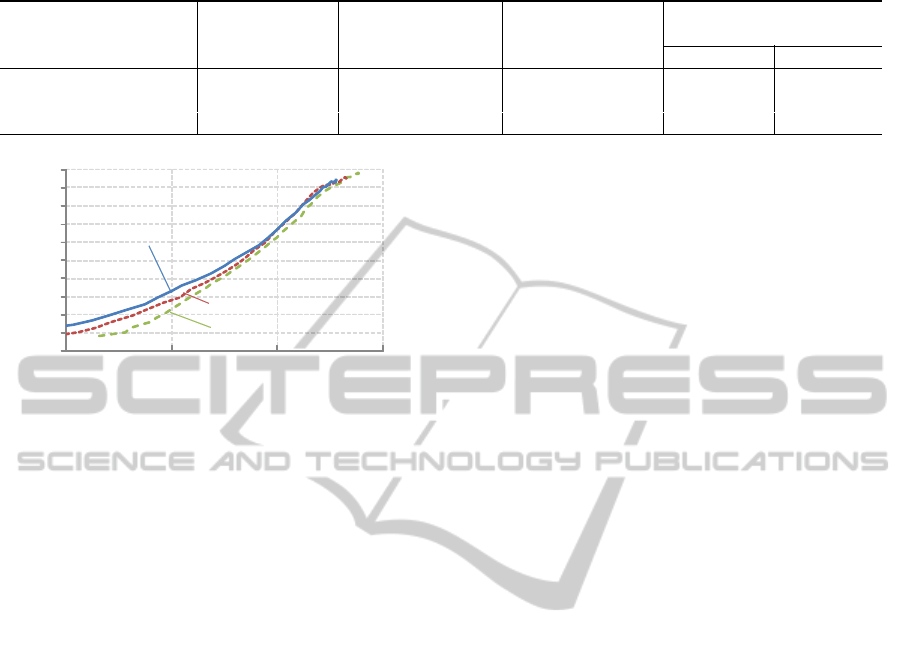
Table 1: Specifications of the proposed method and the comparative methods.
Methods Generation of Classifier Initial inputs of Num. of images used for
training images pedestrian images construction of classifiers
Ped. Non-Ped.
Proposed method yes multiple two-class 200 15,660 35,500
Comparative method 1
yes simple two-class 200 15,660 35,500
Comparative method 2
no simple two-class 15,660 15,660 35,500
0.0
0.1
0.2
0.3
0.4
0.5
0.6
0.7
0.8
0.9
1.0
0.01 0.10 1.00 10.00
Detection rate
False positive per frame
Comparative method 1
Proposed method
Comparative method 2
Figure 9: ROC curves of the proposed method and the com-
parative methods.
three comparative methods. Table 1 shows the spec-
ifications of the proposed method and the compara-
tive methods. The proposed method used 15,660 syn-
thesized pedestrian images (generated from only 200
pedestrian images) and 35,500 non-pedestrian images
to construct the multiple classifiers. Although the
comparative method 1 used the same images with
the proposed method, it applied a simple two-class
classifier. The comparative method 2 used 15,660
pedestrian images including the initial inputs of the
proposed method obtained manually. These methods
were simpler versions of a previous work (Enzweiler
et al., 2009), where they did not employ bootstrapping
iteration when gathering negative samples, compared
to the original method. Since it is difficult to segment
pedestrian regions manually from 15,660 pedestrian
images due to its cost, we could not compare the per-
formance with their multiple classifier versions using
all pedestrian images.
3.4 Results and Discussions
Figure 9 shows the ROC curves of the three methods.
The proposed method and the comparative method
1 outperformed the comparative method 2. Fig-
ure 10 shows examples of the detection results where
the proposed method and the comparative method 1
could detect pedestrians correctly but the comparative
method 2 could not. Here, each result is the result
from a classifier giving the highest performance (F-
measure) for each method.
As can be seen in the first and the second columns
of Fig. 10, although the comparative method 2 could
not detect pedestrians, the proposed method and the
comparative method 1 could detect pedestrians cor-
rectly. In general, to detect pedestrians with complex
backgrounds, the classifier should be trained by us-
ing various training samples including complex back-
grounds. Since the comparative method 2 could not
train various complex backgrounds, it could not de-
tect such pedestrians. In contrast, since the proposed
method and the comparative method 1 synthesized
various pedestrian images with various backgrounds,
these pedestrians could be detected correctly. Also,
the proposed method and the comparative method 1
synthesized variously posed pedestrians for training.
Therefore, these method could also detect pedestrians
whose poses were not included in the initial pedes-
trian images. Thus, we can say that the generative
learning method outperformed the simple gathering
method for the controlled synthesis.
As can be seen in Fig. 9, the performance of
the proposed method outperformed the comparative
method 1. The detection results of these methods
are shown in Fig. 10. From these results, we can
say that the proposed method could detect variously
posed pedestrians in comparison with the comparative
method 1. Especially, it can be observed that the pro-
posed method could detect not only walking pedes-
trians but also standing posed pedestrians. Since the
proposed method constructed multiple classifiers op-
timized for various poses, the detection performance
improved against variously posed pedestrians.
In the proposed method, outputs of the con-
structed multiple classifiers were simply combined by
taking the maximum of the detection scores. How-
ever, the detection performance may be highly af-
fected by an incorrect output of a classifier. There-
fore, we will investigate other methods for combining
the outputs from multiple classifiers.
4 CONCLUSIONS
This paper proposed a novel method for detecting var-
iously posed pedestrians. The proposed method con-
structed multiple classifiers optimized for each pose
INTEGRATION OF GENERATIVE LEARNING AND MULTIPLE POSE CLASSIFIERS FOR PEDESTRIAN
DETECTION
571