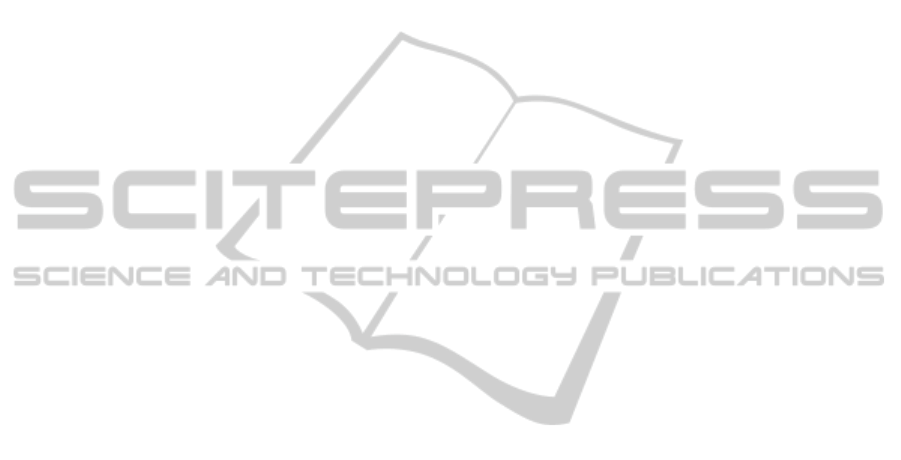
bution must be simultaneous if the analyst is to com-
pare the distributions of the different clusters. The
bundling and C
1
continuity of bundled curves are es-
sential for this comparison to occur, for these fea-
tures allow the viewer to be aware of both clusters
and distribution simultaneously. Bundling exploits
the Gestalt principle of proximity, visually grouping
the lines of a cluster in the middle of the plot. C
1
con-
tinuity exploits the Gestalt principle of continuity to
maintain this visual grouping on the value axes, where
the distribution is represented. This allows the viewer
to compare the distributions of different clusters. As a
secondary benefit, the C
1
continuity allows this clus-
ter identification to be maintained across value axes,
the membership reinforced at each bundle axis.
The same bundling strength was used for both
the correlation and the cluster counting series. This
demonstrates that each task can be achieved without
sacrificing the other.
4 CONCLUSIONS AND FUTURE
WORK
The user study conducted in this work supports the
following conclusions: Firstly, curve bundling is ef-
fective in displaying clustering information purely
based on geometry. Secondly, with a properly cho-
sen bundling strength, bundled curve plots retain the
same strength as polyline plots in revealing correla-
tions between visualized variables. Hence one of the
core aspects of analysis using parallel coordinates car-
ries over using bundling.
The high effectiveness of curved plots compared
with polyline plots was not obvious. The results of
our user study might trigger further perceptual in-
vestigations of variants of parallel-coordinates plots.
It could be the case that other forms of parallel-
coordinates plots might be even more effective than
bundled curves—not only for cluster visualization but
other applications.
ACKNOWLEDGEMENTS
In parts, this work was supported by DFG within SFB
716 / D.5.
REFERENCES
Artero, A. O., de Oliveira, M. C. F., and Levkowitz, H.
(2004). Uncovering clusters in crowded parallel coor-
dinates visualizations. In IEEE Symposium on Infor-
mation Visualization, pages 81–88. IEEE Computer
Society.
Berthold, M. R. and Hall, L. O. (2003). Visualizing fuzzy
points in parallel coordinates. IEEE Transactions on
Fuzzy Systems, 11:369–374.
Caat, M. T. and Maurits, N. M. (2007). Design and evalu-
ation of tiled parallel coordinate visualization of mul-
tichannel EEG data. IEEE Transactions on Visualiza-
tion and Computer Graphics, 13(1):70–79.
Cook, D. and Swayne, D. F. (2003). Interactive and Dy-
namic Graphics for Data Analysis: With Examples
Using R and GGobi. Springer.
Fua, Y.-H., Ward, M. O., and Rundensteiner, E. A. (1999).
Hierarchical parallel coordinates for exploration of
large datasets. In IEEE Visualization, pages 43–50.
IEEE Computer Society.
Graham, M. and Kennedy, J. (2003). Using curves to en-
hance parallel coordinate visualisations. In Interna-
tional Conference on Information Visualization (IV),
pages 10–16. IEEE Computer Society.
Harrower, M. A. and Brewer, C. A. (2003). Color-
Brewer.org: an online tool for selecting color schemes
for maps. The Cartographic Journal, 40(1):27–37.
Heinrich, J., Bachthaler, S., and Weiskopf, D. (2011a).
Progressive splatting of continuous scatterplots and
parallel coordinates. Computer Graphics Forum,
30(3):653–662.
Heinrich, J., Luo, Y., Kirkpatrick, A. E., Zhang, H., and
Weiskopf, D. (2011b). Evaluation of a bundling tech-
nique for parallel coordinates. Technical Report Com-
puter Science TR-2011-08, Visualization Research
Center, University of Stuttgart.
Heinrich, J. and Weiskopf, D. (2009). Continuous parallel
coordinates. IEEE Transactions on Visualization and
Computer Graphics, 15(6):1531–1538.
Henley, M., Hagen, M., and Bergeron, R. D. (2007). Eval-
uating two visualization techniques for genome com-
parison. In International Conference on Information
Visualization (IV), pages 551–558. IEEE Computer
Society.
Holten, D. (2006). Hierarchical edge bundles: Visualiza-
tion of adjacency relations in hierarchical data. IEEE
Transactions on Visualization and Computer Graph-
ics, 12(5):741–748.
Holten, D. and van Wijk, J. J. (2010). Evaluation of cluster
identification performance for different PCP variants.
Computer Graphics Forum, 29(3):793–802.
Inselberg, A. (1985). The plane with parallel coordinates.
The Visual Computer, 1(2):69–92.
Inselberg, A. (2009). Parallel Coordinates: Visual Multidi-
mensional Geometry and Its Applications. Springer,
New York.
Inselberg, A. and Dimsdale, B. (1990). Parallel coordinates:
A tool for visualizing multi-dimensional geometry. In
IEEE Visualization, pages 361–378. IEEE Computer
Society.
Jain, A. K. and Dubes, R. C. (1988). Algorithms for Clus-
tering Data. Prentice-Hall, Upper Saddle River, NJ.
EVALUATION OF A BUNDLING TECHNIQUE FOR PARALLEL COORDINATES
601