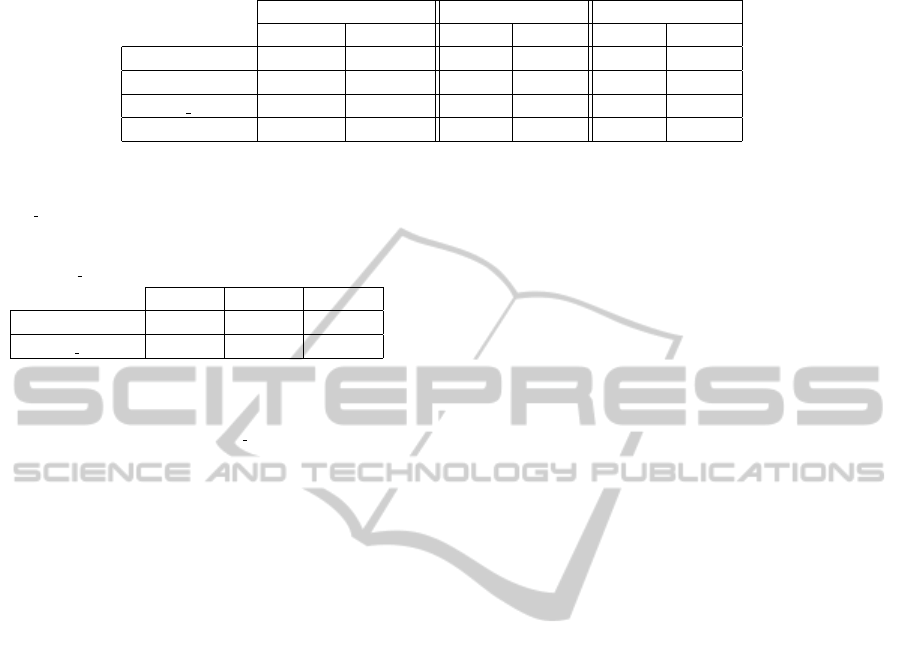
Table 2: Global average precision (MAP), average precision for Mb and average precision for ER for different classifiers.
mAP AP for Mb AP for ER
µ(mAP) σ(mAP) µ(AP) σ(AP) µ(AP) σ(AP)
k-NN 84.22 2.56 94.81 2.02 73.64 5.63
UNN
s
86.04 2.54 94.48 1.90 77.60 5.46
UNN
s adaptive
87.67 1.93 89.27 2.26 86.08 3.78
SVM 76.46 4.55 95.58 2.38 57.34 10.67
Table 1: This table shows the percentage of prototypes
number selected from the training set by both UNN
s
and
UNN
s adaptive
: We report the total number (N
t
), the one in
the class Mb (N
Mb
), and in the class ER (N
ER
). The distribu-
tion of selected prototypes on both classes is more balanced
using UNN
s adaptive
.
N
t
N
Mb
N
ER
UNN
s
69.24% 50.20% 19.03%
UNN
s adaptive
47.69% 28.58% 19.11%
classification arises on the minority class (ER) using
k-NN , thus giving an average precision (AP) of about
73% (see Tab. 2). Using UNN
s adaptive
classification
improved MAP of the minority class up to 86% thus
13% better than k-NN
˙
For the SVM classification, the
result in Tab. 2 shows that there is an important classi-
fication error on ER cells where the AP is about only
57%.
4 CONCLUSIONS
In this paper, we have presented a novel algorithm for
automatic segmentation and classification of cellular
images based on different subcellular distributions of
the NIS protein. First of all, our method relies on
extracting highly discriminative descriptors based on
bio-inspired histograms of Difference-of-Gaussians
(DoG) coefficients on cellular regions. Then, we
propose a supervised classification algorithm, called
UNN, for learning the most relevant prototypical
samples that are to be used for predicting the class of
unlabeled cellular images according to a leveraged k-
NN rule. We evaluated UNN performances on a sig-
nificantly large database of cellular images that were
manually annotated. Although being the very early
results of our methodology for such a challenging ap-
plication, performances are really satisfactory (aver-
age global precision of 87.5% and MAP of the mi-
nority class up to 86%) and suggest our approach as a
valuable decision-support tool in cellular imaging.
REFERENCES
Bel haj ali, W., Debreuve, E., Kornprobst, P., and Bar-
laud, M. (2011). Bio-Inspired Bags-of-Features for
Image Classification. In International Conference
on Knowledge Discovery and Information Retrieval
(KDIR 2011).
Dayem, M., Basquin, C., Navarro, V., Carrier, P., Marsault,
R., Chang, P., Huc, S., Darrouzet, E., Lindenthal, S.,
and Pourcher, T. (2008). Comparison of expressed
human and mouse sodium/iodide symporters reveals
differences in transport properties and subcellular lo-
calization. Journal of Endocrinology, 197(1):95–109.
Field, D. J. (1994). What is the goal of sensory coding?
Neural Computation, 6(4):559–601.
Lowe, D. G. (2004). Distinctive image features from scale-
invariant keypoints. International Journal of Com-
puter Vision, 60(2):91–110.
Oliva, A. and Torralba, A. (2001). Modeling the shape
of the scene: A holistic representation of the spatial
envelope. International Journal of Computer Vision,
42:145–175. 10.1023/A:1011139631724.
Peyrottes, I., Navarro, V., Ondo-Mendez, A., Marcellin, D.,
Bellanger, L., Marsault, R., Lindenthal, S., Ettore, F.,
Darcourt, J., and Pourcher, T. (2009). Immunoanalysis
indicates that the sodium iodide symporter is not over-
expressed in intracellular compartments in thyroid and
breast cancers. European Journal of Endocrinology,
160(2):215–25.
Piro, P., Nock, R., Nielsen, F., and Barlaud, M. (2010).
Multi-Class Leveraged k-NN for Image Classification.
In Proceedings of the Asian Conference on Computer
Vision (ACCV 2010).
Piro, P., Nock, R., Nielsen, F., and Barlaud, M. (2012).
Leveraging k-nn for generic classification boosting.
Neurocomputing, 80:3–9.
Schapire, R. E. and Singer, Y. (1999). Improved boosting al-
gorithms using confidence-rated predictions. Machine
Learning, 37:297–336.
Van Rullen, R. and Thorpe, S. J. (2001). Rate coding versus
temporal order coding: what the retinal ganglion cells
tell the visual cortex. Neural Comput, 13(6):1255–
1283.
VISAPP 2012 - International Conference on Computer Vision Theory and Applications
584