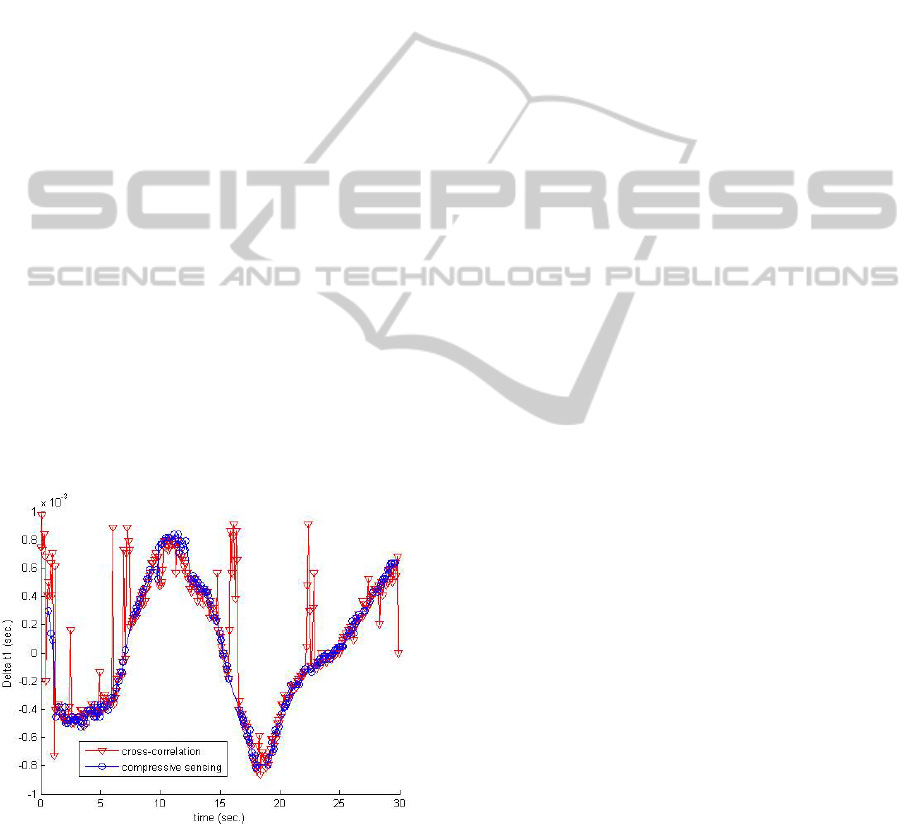
less than 1 during the initial segment in which the
cross-correlation result is unreliable as well. After
the initial silent segment, the confidence indicator is
infinity and the result matches with the cross-
correlation very well.
In the second experiment, the setup represents a
difficult environment in which echoes and noise are
abundant. In addition, the speaker is constantly
moving around. The sample rate for this experiment
is
kHzf
s
1.44=
. The result, compared with the
cross-correlation, is shown in Figure 7.
This is a difficult situation as is evident by many
erroneous results from the cross-correlation method.
Admittedly, the compressive sensing method made
many erroneous calculations as well, but the use of
the confidence indicator is able to identify and
eliminate the unreliable data points. The eliminated
data points from compressive sensing are not plotted
in Figure 7, which can be seen from the connected
line without the symbol. It is worthwhile to point out
that it is possible to apply certain processing to
eliminate the erroneous results from the cross-
correlation method as well. However, the purpose of
showing the result from the cross-correlation
method without further processing is to illustrate
that the environment used in this experiment is a
difficult one, and even in this difficult environment,
the compressive sensing method is able to produce
reasonable result with a high compression ratio of
more than 100 to 1.
Figure 7: Two sensors with noisy signals.
5 CONCLUSIONS
Compressive sensing is an effective technique for
localization of sound source in a sensing network.
Compressive measurements can be used to reliably
estimate the time difference of arrival (TDOA) of
sound signals at the sensors, without any assumption
on the sparseness of the sound source. We have
demonstrated reliable detection and tracking of
sound source by using compressive measurements
with a compression ratio of more than 100 times, as
compared to the traditional Nyquist sampling.
REFERENCES
Knapp, C. Y., Carter, G. C., 1976, The generalized
correlation method for estimation of time delay, IEEE
Trans. Acoust. Speech, Signal Processing, vol. ASSP-
24, pp. 320-327.
Valin, J., Michaud, F, Rouat, J, L´etourneau, D., 2003,
Robust Sound Source Localization Using a
Microphone Array on a Mobile Robot, Proceedings of
2003 IEEE/RSJ International Conference on
Intelligent Robots and Systems (IROS 2003), vol.2, pp.
1228 – 1233.
Benesty, J., Chen, J., Huang, Y., 2008, Adaptive
Eigenvalue Decomposition Algorithm, Microphone
Array Signal Processing, pp. 207-208. Berlin,
Germany: Springer-Verlag.
Carter, G. C., Nuttall, A. H., Cable, P. G., 1973, The
smoothed coherence transform,” Proc. IEEE, vol. 61,
pp. 1497-1498.
Candès, E., Romberg, J., Tao, T., 2006, Robust
uncertainty principles: Exact signal reconstruction
from highly incomplete frequency information, IEEE
Trans. on Information Theory, vol 52, no 2, pp. 489-
509.
Rife, D. D., Vanderkooy, J., 1989, Transfer-Function
Measurement with Maximum-Length Sequences,
Journal of the Audio Engineering Society, vol 37, no
6, pp. 419-444.
Lin, Y., Lee, D. D., Saul, L. K., 2004, Nonnegative
deconvolution for the time of arrival estimation, IEEE
International Conference on Acoustics, Speech, and
Signal Processing, Proceedings. (ICASSP '04), vol 2,
pp. 377-380.
Li, C., Jiang, H., Wilford, P. A., Zhang, Y., 2011, Video
coding using compressive sensing for wireless
communications, IEEE Wireless Communications and
Networking Conference (WCNC), 10.1109/WCNC.
2011. 5779474, pp. 2077 – 2082.
Jiang, H. Li, C., Haimi-Cohen, R., Wilford, P., Zhang, Y.,
2012, Scalable Video Coding using Compressive
Sensing, Bell Labs Technical Journal, vol. 16, no. 4.
SENSORNETS 2012 - International Conference on Sensor Networks
166