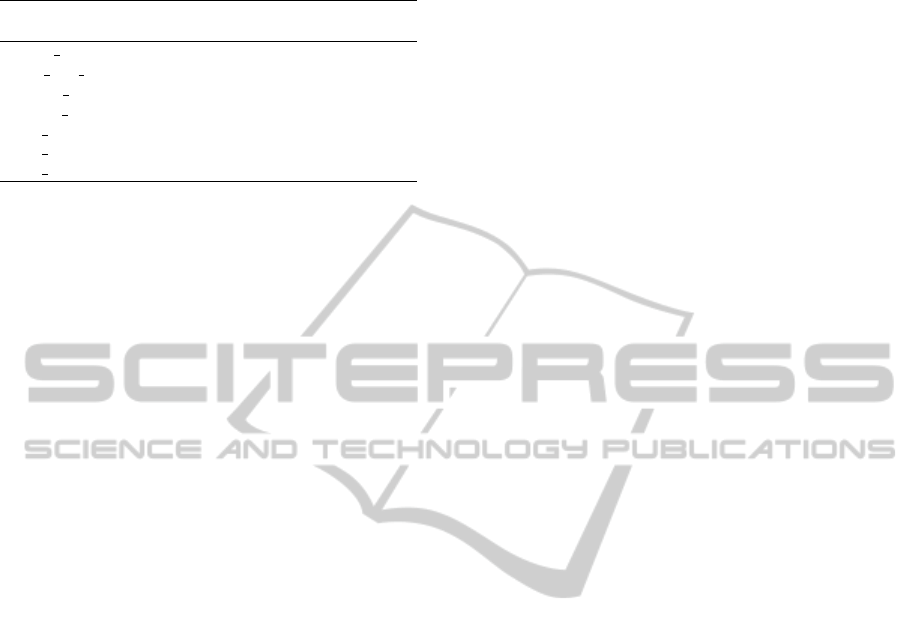
Table 2: Differences between a full processing and process-
ing only X −T . Strict values are given in round brackets.
Clip name Hit diff. Hit rate diff.
(frames) (Percentage Points)
BELA WALK 3 (1) 7.5 (2.5)
KTH P06 HANDWAVE 4 (4) 4 (4)
DARIA JUMP 0 (6) 0 (10)
MAHA TRAFFIC 5 (6) 2.6 (3.2)
GET LTR1 0 (0) 0 (0)
GET RTL1 -1 (-1) -5 (-5)
GET TD 2 (2) 6.7 (6.7)
spatial saliency independently, which enables differ-
ent spatial and temporal resolutions for this integra-
tion. The visualized output from the experiments
shows reasonable saliency deployment and the hit
counts reflect good results for most of the test clips
from a heterogeneous set. Top-down experiments
were conducted to show how the model can be in-
fluenced to prefer a direction of motion, the mecha-
nism can be extended to include further features. Our
system is able to perform online on continuous in-
put. The result lags behind up to a few seconds which
is due to the concept (collecting a volume first) and
computation time. We demonstrated that by using
only X − T slices, the lag can be reduced with only
little influence on the quality of the outcome. In fu-
ture work we will integrate spatiotemporal with spa-
tial saliency processing and focus on grouping the re-
gions to from “real” objects based on the attentional
results to enable a quantitative comparison with man-
ually marked test clips or eye-tracker data.
ACKNOWLEDGEMENTS
This work was supported by the German Re-
search Foundation (DFG) under grant Me 1289/12-
1(AVRAM). The authors also wish to thank Kon-
stantin Werkner for improvements suggested for the
algorithms and Dr. Zaheer Aziz for his useful com-
ments on the manuscript.
REFERENCES
Aziz, M. Z. (2009). Behavior adaptive and real-time model
of integrated bottom-up and top-down visual atten-
tion. Dissertation, Universit
¨
at Paderborn.
Aziz, M. Z. and Mertsching, B. (2008a). Fast and robust
generation of feature maps for region-based visual at-
tention. In IEEE Transactions on Image Processing,
volume 17, pages 633–644.
Aziz, M. Z. and Mertsching, B. (2008b). Visual search in
static and dynamic scenes using fine-grain top-down
visual attention. In ICVS, volume 5008, pages 3–12.
Belardinelli, A., Pirri, F., and Carbone, A. (2008). Mo-
tion saliency maps from spatiotemporal filtering. In
WAPCV, pages 112–123.
CAVIAR (2001). EC funded caviar project/IST 2001
37540; http://groups.inf.ed.ac.uk/vision/CAVIAR/
CAVIARDATA1/. [Online; accessed 5-September-
2011].
Cui, X., Liu, Q., and Metaxas, D. (2009). Temporal spec-
tral residual: Fast motion saliency detection. In Proc.
ACM Multimedia, pages 617–620. ACM.
Goodale, M. A. and Milner, A. D. (1992). Separate visual
pathways for perception and action. Trends in Neuro-
sciences, 15(1):20–25.
Gorelick, L., Blank, M., Shechtman, E., Irani, M., and
Basri, R. (2007). Actions as space-time shapes. In
IEEE PAMI, volume 29, pages 2247–2253.
Guo, C., Ma, Q., and Zhang, L. (2008). Spatio-temporal
saliency detection using phase spectrum of quaternion
fourier transform. In IEEE CVPR, pages 1–8.
Hou, X. and Zhang, L. (2007). Saliency detection: A spec-
tral residual approach. In IEEE CVPR, pages 1–8.
Itti, L. and Baldi, P. F. (2006). Bayesian surprise attracts
human attention. In NIPS, pages 547–554.
Itti, L., Koch, C., and Niebur, E. (1998). A model of
saliency-based visual attention for rapid scene anal-
ysis. In IEEE PAMI, volume 20, pages 1254–1259.
K. Rapantzikos S. Kollias, T. A. (2009). Spatiotemporal
saliency for video classification. Signal Processing:
Image Communication, 24:557–571.
Livingstone, M. and Hubel, D. (1987). Psychophysical evi-
dence for separate channels for the perception of form,
color, movement, and depth. The Journal of Neuro-
science, 7(11):3416–3468.
Mahadevan, V. and Vasconcelos, N. (2010). Spatiotempo-
ral saliency in dynamic scenes. In IEEE PAMI, vol-
ume 32, pages 171–177.
Mahapatra, D., Winkler, S., and Yen, S.-C. (2008). Mo-
tion saliency outweighs other low-level features while
watching videos. In SPIE, volume 6806.
Quigley, M., Conley, K., Gerkey, B. P., Faust, J., Foote, T.,
Leibs, J., Wheeler, R., and Ng, A. Y. (2009). ROS: An
open-source robot operating system. In ICRA Work-
shop on Open Source Software.
Sch
¨
uldt, C., Laptev, I., and Caputo, B. (2004). Recognizing
human actions: A local svm approach. In ICPR, pages
32–36.
Seo, H. J. and Milanfar, P. (2009). Static and space-time
visual saliency detection by self-resemblance. Journal
of Vision, 9(12).
T
¨
unnermann, J. (2010). Biologically inspired spatiotem-
poral saliency processing to enhance a computational
attention model. Master’s thesis, Universit
¨
at Pader-
born.
Wischnewski, M., Belardinelli, A., Schneider, W. X., and
Steil, J. J. (2010). Where to look next? Combin-
ing static and dynamic proto-objects in a TVA-based
model of visual attention. Cognitive Computation,
2(4):326–343.
CONTINUOUS REGION-BASED PROCESSING OF SPATIOTEMPORAL SALIENCY
239