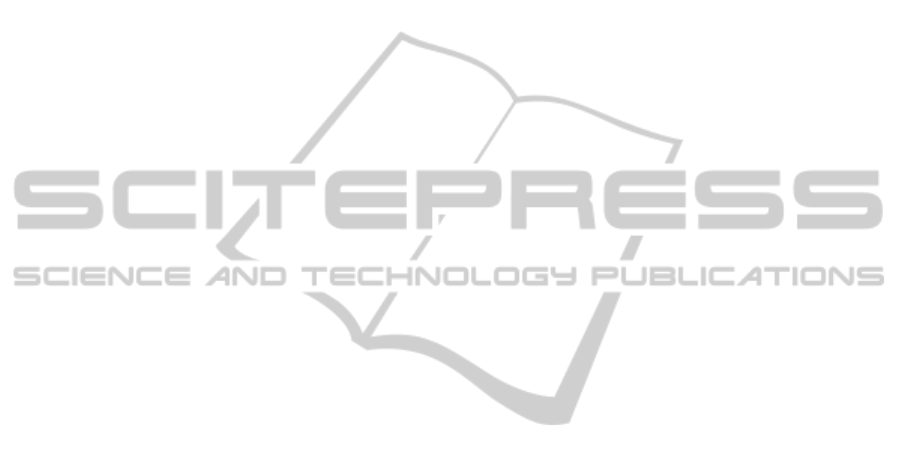
are more likely to reproduce the behavior in simple
structures and thus improve training efficiency and
performance.
Our main conclusion is that research in artificial
intelligence should be aware that there is no sin-
gle ‘correct’ machine learning structure for particular
task and that the results obtained may be substantially
influenced by the individual that is modeled in this
structure.
ACKNOWLEDGEMENTS
This work has been supported by the FEMtech pro-
gram of The Federal Ministry for Transport, Innova-
tion and Technology under FFG grant No. 318113. It
reflects only the authors’ views.
REFERENCES
Breiman, L. (2001). Random forests. Machine Learning,
45(1):5–32.
Breiman, L., Friedman, J., Stone, C. J., and Olshen, R. A.
(1993). Classification and Regression Trees. Chap-
man and Hall/CRC, Boca Raton, FL.
Cohen, J. (1988). Statistical Power Analysis for the Behav-
ioral Sciences. Lawrence Erlbaum Associates, Mah-
wah, NJ, 2 edition.
Donmez, P., Lebanon, G., and Balasubramanian, K. (2010).
Unsupervised supervised learning i: Estimating clas-
sification and regression errors without labels. J.
Mach. Learn. Res., 11:1323–1351.
Drury, C. G. (1978). Integrating human factors models into
statistical quality control. Human Factors: The Jour-
nal of the Human Factors and Ergonomics Society,
20(12):561–572.
Eitzinger, C., Heidl, W., Lughofer, E., Raiser, S., Smith,
J., Tahir, M., Sannen, D., and Van Brussel, H. (2009).
Assessment of the influence of adaptive components
in trainable surface inspection systems. Machine Vi-
sion and Applications, 21(5):613–626.
Garrett, S. K., Melloy, B. J., and Gramopadhye, A. (2001).
The effects of per-lot and per-item pacing on inspec-
tion performance. International Journal of Industrial
Ergonomics, 27(5):291–302.
Heidl, W., Thumfart, S., Lughofer, E., Eitzinger, C., and
Klement, E. P. (2010). Classifier-based analysis of
visual inspection: Gender differences in decision-
making. In Proceedings of SMC2010, IEEE Confer-
ence on Systems, Man and Cybernetics, pages 113–
120.
Heidl, W., Thumfart, S., Lughofer, E., Eitzinger, C., and
Klement, E. P. (2011). Decision tree-based analy-
sis suggests structural gender differences in visual in-
spection. In Proceedings of AIA2011, IASTED Inter-
national Conference on Artificial Intelligence and Ap-
plications, pages 142–149.
Jain, A. K., Prabhakar, S., and Hong, L. (1999). A mul-
tichannel approach to fingerprint classification. Pat-
tern Analysis and Machine Intelligence, IEEE Trans-
actions on, 21(4):348–359.
Moffat, S. D., Hampson, E., and Hatzipantelis, M. (1998).
Navigation in a ”virtual” maze: Sex differences and
correlation with psychometric measures of spatial
ability in humans. Evolution and Human Behavior,
19(2):73–87.
Peacock, A., Ke, X., and Wilkerson, M. (2004). Typing
patterns: a key to user identification. Security Privacy,
IEEE, 2(5):40–47.
Ruggieri, S., Pedreschi, D., and Turini, F. (2010). Data min-
ing for discrimination discovery. ACM Trans. Knowl.
Discov. Data, 4(2):9:1–9:40.
Stevens, R. and Soller, A. (2005). Machine learning models
of problem space navigation: The influence of gender.
Computer Science and Information Systems/ComSIS,
2(2):83–98.
Thumfart, S., Jacobs, R. A., Lughofer, E., Eitzinger, C.,
Cornelissen, F. W., Groißb
¨
ock, W., and Richter, R.
(2011). Modelling human aesthetic perception of vi-
sual textures. Accepted for publication in ACM Trans.
on Applied Perception.
Yu, K., Wang, Y., and Tan, T. (2004). Writer identifica-
tion using dynamic features. In Zhang, D. and Jain,
A. K., editors, Biometric Authentication, volume 3072
of Lecture Notes in Computer Science, pages 1–8.
Springer Berlin / Heidelberg.
Zhao, W., Chellappa, R., Phillips, P. J., and Rosenfeld, A.
(2003). Face recognition: A literature survey. ACM
Comput. Surv., 35(4):399–458.
ICAART 2012 - International Conference on Agents and Artificial Intelligence
418