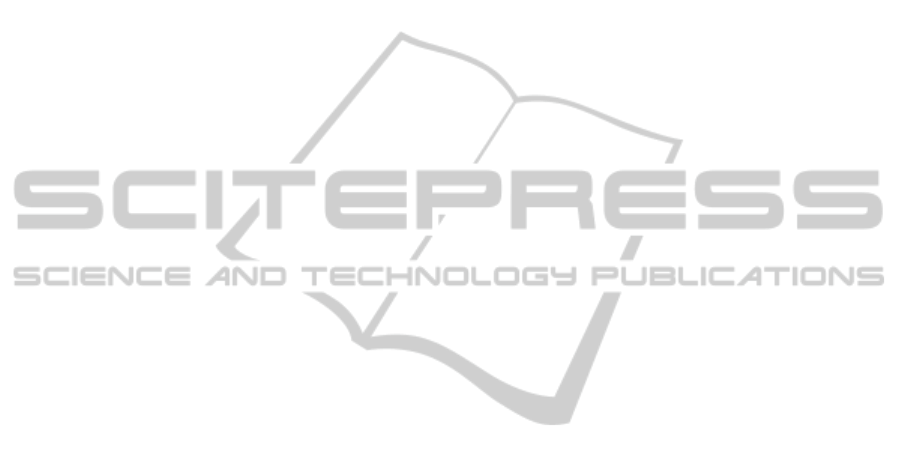
stable periodic states, as shown in Figure 4 (a) and
(b), can lead to logic ‘low level’ or ‘high level’ and
can in principle create large memory capacity as
input data bit streams in digital network systems. Its
implementation also still requires much engineering
improvements, such as arriving target at a spatial
resolution speckle, and suppression of its tendency
to chaos. We will focus on a more realistic case of N
weighted pumps, i.e., one v
p
, one v
s
and v
n1
, v
n2
,
v
n3
…
nn
. The optical sensor based neural networks is
typically configured into an array for the sensor
networks in optical fiber. This sensor concept can be
used to form either adaptive sensor arrays which are
similar to our researched neural network system, or
used simply as an embedded sensor inside structures
or materials.
4 CONCLUSIONS
Controlling of chaotic instabilities in optical fiber
sensor networks has been implemented under chaos-
induced transient instability in optical systems.
Controlling also leads to the possible logic theory
with ‘low level’ or ‘high level’, as logic ‘0’ and ‘1’
with stable and periodic states. It’s used for neural
networks as a neural net. It is theoretically possible
to apply the multi-stability data regimes as an optical
large memory device for multi encoding-decoding
messages. It can be also applied for complex data
transmission in TDM networks and other optical
communications. It can be possible to create large
optical memory capacity.
REFERENCES
Cotter, D., 1983. “Stimulated Brillouin scattering in
Monomode Optical Fiber.” J. Opt. Com. 4, 10-19.
Yong, K, Kim., Choon, B, Park., 2003. “Study of chaotic
and instability effect of optical fiber using on the
internet.” SPIE. 5246, 648-655.
W. B. Lyons and E. Lewis, 2000, “Neural networks and
pattern recognition techniques applied to optical fibre
sensors,” Transaction of the Institute of Measurement
and Control 22(5), 385-404.
D. king, W. B. Lyons, C. Flanggan and E. Lewis, 2003,
“An optical fibre ethanol concentration sensor
utilizing Furier transform signal processing analysis
and artificial neural network pattern recognition,” J.
Opt. A: Pure Appl.Opt. 5, S69-S75.
Tariq, S., Habib, M, K., 1998. “Neural operation using
stimulated Brillouin scattering in optical fiber.” Opt.
Eng. 37, 1823-1826.
Agrawal, G, P., 2001. “Nonlinear Fiber Optics, 3rd,
Academic press”, London.
V. I. Kovalev and R. G. Harrison, 2006. “Suppression of
stimulated Brillouin scattering in high-power single
frequency fiber amlifiers,” Opt. Lett.31, 161-163.
C. Wang, F. Zhang, Z. Tong, T. Ning, and S. Jian., 2008.
“Suppression of stimulated Brillouin scattering in
high-power single-frequency multicore fiber
amplifiers,” Opt. Fiber Technol. 14, 328-338.
J. B. Coles, B. P. P. Kuo, N. Alic, S. More, C. S. Bres, J.
M. Chavez Boggio, P. A. Andrekson, M. Karlsson,
and S. Radic., 2010. “Bandwidth efficient phase
modulation techniques for stimulated Brillouin
scattering suppression in fiber optic parametric
amplifiers,” Opt. Express 18, 18138-18150.
Yang Jiang, J. Yu, Binfchen Han, Li Zhang, Wenrui Wang,
Lital Zhang, and Enze Yang., 2009. “Millimeter-wave
subcarrier generation utilizing four-wave mixing and
dual-frequency Brillouin pump suppression,” Opt. Lett.
48(3), 030502-1 – 030502-2.
S. Mussot, M. Le Parquier, P. Szriftgiser., 2010. “Thermal
noise for SBS suppression in fiber optical parametric
amplifiers,” Opt. Communication. 283, 2607-2610.
SENSORNETS 2012 - International Conference on Sensor Networks
180