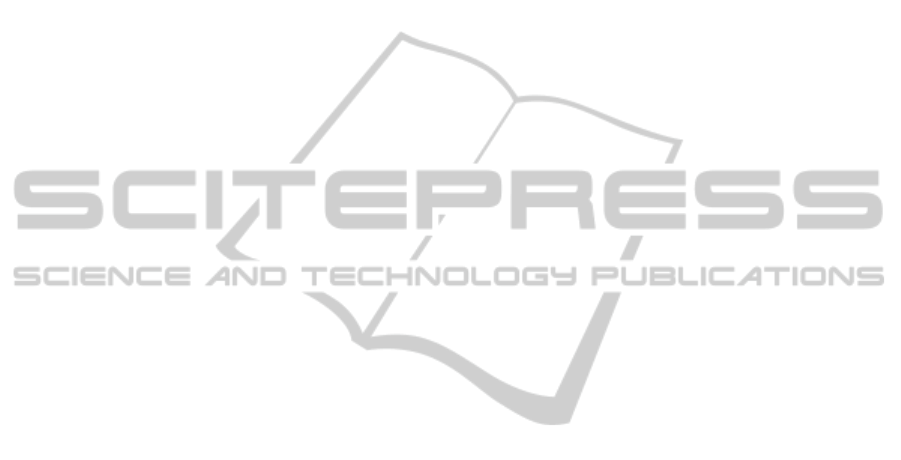
positive affection or negative affection. After the
evaluation, we can build two emotional backchannel
lexicons. By detecting features of the speaker, the
system can analyze emotional state and randomly
select one type of backchannel from the similar
emotional backchannel lexicon of agent listener in
accordance with the empathy strategy.
With the empathy strategy and emotional
backchannel lexicon, the virtual agent listener can
‘feel’ the speaker’s emotional state and give
appropriate feedbacks.
It is obvious that detecting the speaker’s features
is the foundation of the system. Performance of the
speaker’s emotional state detection will greatly
influence the performance of our system. We
propose to combine the speaker’s facial expressions
and speeches detected by camera and microphone to
analyze his emotion. Although emotional facial
expression and emotional speech recognition have
developed for years, recognition results are not
perfect for various emotions especially in real-time
systems. We concern the implementation of our
system, so we only divide emotional states into two
kinds which are positive affection and negative
affection and build two corresponding emotional
backchannel lexicons. These two emotional states
can be recognized correctly in current real-time
experiments. Therefore we can apply the recognition
technology in the backchannel system.
4 CONCLUSIONS
In face-to-face interaction between a human speaker
and a virtual agent listener, addressing backchannel
feedbacks to virtual agent listener gives the agent
human-like conversation skills and creates rapport in
Human-Computer Interaction. In recent years,
researchers have made great efforts in predicting
backchannel for the agent listener. In this position
paper, we have argued the limitations of current
approaches. It is time for us to look for new methods
to improve the backchannel prediction and
generation.
Following the two hypotheses emphasizing on
the timing and type of backchannel, we introduce an
improved system to enhance the agent listener’s
performance. In the backchannel prediction part,
using Newcastle Personality Assessor before
parasocial consensus sampling and neural networks
will enable us to obtain the personality rules related
to the number of backchannel. Then we can easily
select different backchannel timing thresholds for
specific agent listener’s personality. In the
backchannel generation part, we intend to build two
emotional backchannel lexicons showing positive
affection and negative affection respectively after
conducting a context-free perceptual study. The
system will randomly select one type of backchannel
from the similar emotional backchannel lexicon in
accordance with the empathy strategy. Further steps
may include asking some volunteers to assess the
system and keeping on developing it according to
the evaluation results. These efforts will help to
make the system more suitable for different
conversation occasions. Implementation of the
proposed system will greatly increase the
naturalness between the human speaker and the
agent listener.
ACKNOWLEDGEMENTS
This work is supported by the National Nature
Science Foundation of China (No.61103097,
No.60873269), International Science and
Technology Cooperation Program of China
(No.2010DFA11990).
REFERENCES
Bavelas, J. B., Coates, L., Johnson, T. (2002). Listener
responses as a collaborative process: The role of gaze.
Journal of Communication, 52(3), 566-580.
Bavelas, J. B., Coates, L., Johnson, T. (2000). Listeners as
conarrators. Journal of Personality and Social
Psychology, 79(6), 941-952.
Cathcart, N., Carletta, J., Klein, E. (2003). A shallow
model of backchannel continuers in spoken dialogue.
Proceedings of the Conference of the European
chapter of the Association for Computational
Linguistics, 51-58.
Duncan, Starkey, Jr. and Fiske D. (1977). Face-to-face
Interaction. New York: Halsted Press.
Duncan, Starkey, Jr. (1974). On the structure of speaker-
auditor interaction during speaking turns. Language in
Society, 3(2): 161-180.
Gratch, J., Okhmatovskaia, A., Lamothe, F., Marsella, S.,
Morales, M., Werf, R. J. V. D., Morency, L. (2006).
Virtual Rapport. Proceedings of the International
Conference in Intelligent Virtual Agent, 14-27.
Huang L., Morency, L., Gratch, J. (2010a). Parasocial
Consensus Sampling: Combining Multiple
Perspectives to Learn Virtual Human Behaviour.
Proceedings of AAMAS 2010, 1265-1272.
Huang L., Morency, L., Gratch, J. (2010b). Learning
backchannel prediction model from parasocial
consensus sampling. Proceedings of the International
Conference in Intelligent Virtual Agent, 159-172.
ICAART 2012 - International Conference on Agents and Artificial Intelligence
262