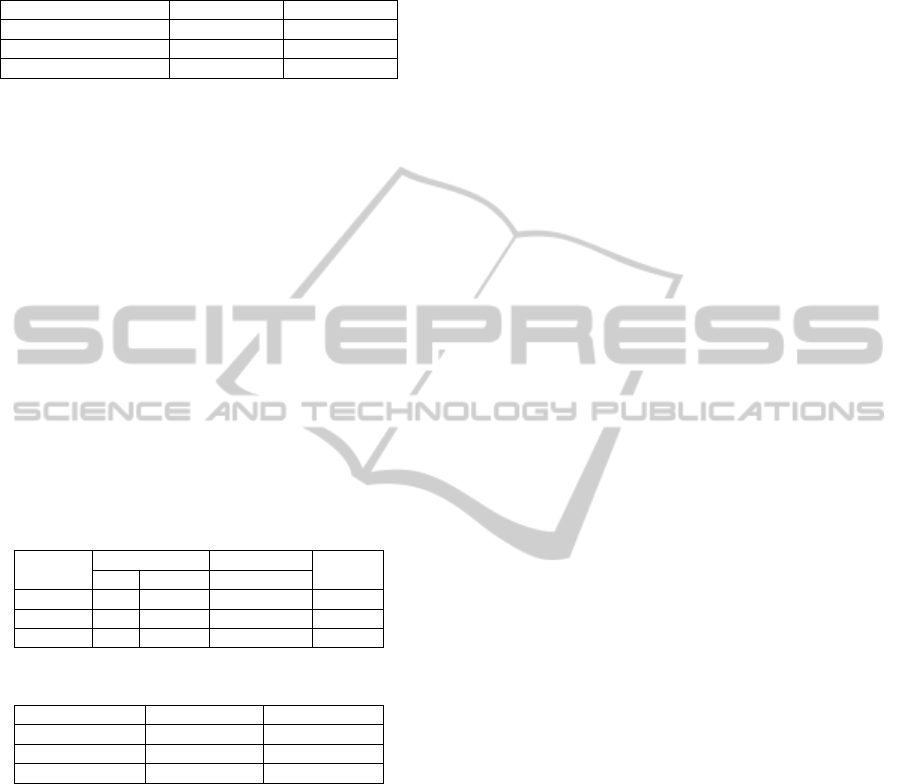
systems after data fusion operation, the intermediate
value for threshold has been exploited.
Table 1: Wearable accelerometer performance.
Shape Threshold Efficiency Reliability
Low (THL) 98.0 % 61.5 %
Medium (THM) 88.4 % 79.3 %
High (THH) 55.1 % 97.2 %
6.3 Data Fusion Results
The data fusion process improves the detection
performance thanks to the addition of both
analogous and complementary information. A
sample output of fall probability is shown in Table 2
in correspondence of two different kinds of falls and
one normal activity. From the rows of the table, it
can be seen as the merged fall probability (the
ALARM column) improves the fall probability of
each sub-system alone. In order to easy the
performance comparison, the scores related to each
standalone sub-system and the ones related to the
data fusion are reported jointly in Table 3. The
performance of the comprehensive framework
underlines a significant raise in both efficiency and
reliability.
Table 2: Sample output of fall probability.
Activity
Vision S. Wearable S.
ALARM
Fall Posture Fall
FL 0,87 Lying 0,82 0,95
FBWR 0,64 Sitting 0,73 0,44
SITF 0,40 Sitting 0,62 0,36
Table 3: System Performance.
Sub-system Efficiency Reliability
TOF camera 80.0 % 97.3 %
Accelerometer 88.4 % 79.3 %
Data fusion 94.3 % 98.2 %
7 CONCLUSIONS
A multi-sensor framework for indoor people fall
detection has been developed and experimental
results with real actors following state-of-the art
guidelines (Noury, 2009) have been performed first
for each single sensor sub-system and then for the
overall system. A fuzzy-based data fusion logic has
been proposed able to effectively handle the
uncertainty present in AAL contexts. The presented
system improves the performance of people fall
detection by processing multiple sensors data,
showing that SNs are a very promising approach for
critical event detection with low false alarm rate. In
addition, fuzzy rules can be easily modified and
adjusted in order to meet specific environment
constraints. Furthermore, the deployment of the
presented multi-sensor fall detection system in
apartments dwelled by elderly people is planed in
order to validate the system in real conditions.
ACKNOWLEDGEMENTS
The presented work has been carried out within the
Netcarity consortium funded by the EC, FP6.
REFERENCES
Brulin, D., Courtial, E., 2010, “Multi-sensors data fusion
system for fall detection,” In: Proceedings of 10th
IEEE ITAB, pp. 1-4.
Cucchiara, R., Prati, A., Vezzani, R., 2007, “A multi-
camera vision system for fall detection and alarm
generation,” Expert Syst J; vol 24, no. 5, pp. 334-45.
Grassi, M., Lombardi, A., Rescio, G., Ferri, M.,
Malcovati, P., Leone, A., Diraco, G., Siciliano, P.,
Malfatti, M., Gonzo, L., 2010, “An Integrated System
for People Fall-Detection with Data Fusion
Capabilities Based on 3D ToF Camera and Wireless
Accelerometer,” In: Proceedings of IEEE Sensors, pp.
1016-1019.
Hu, W., Hu, M., Zhou, X., Tan, T., Lou, J., Maybank, S.,
2006, “Principal axis-based correspondence between
multiple cameras for people tracking,” Pattern
Analysis and Machine Intelligence, IEEE Transactions
on; vol. 28, no. 4, pp. 663-671.
Leone, A., Diraco, G., Siciliano, P., 2009, “Detecting falls
with 3D range camera in ambient assisted living
applications: A preliminary study,” Medical
Engineering & Physics; vol. 33, no. 6, pp. 770-781.
Leone, A., Diraco, G., Siciliano, P., 2011, “Topological
and volumetric posture recognition with active vision
sensor in AAL contexts,” In: IEEE IWASI, pp. 110-
114
MESA Imaging AG, 2011, “SR4000 Data Sheet Rev.5.1,”
Zurich, Switzerland, 26 August 2011,
<http://www.mesa-imaging.ch>.
Netcarity UE Integrated Project, www.netcarity.org.
Noury, N., Fleury, A., Rumeau, P., Bourke, A. K.,
Laighin, G. O., Rialle, V., Lundy, J. E., 2009, “Fall
detection - Principles and Methods”, In: Proceedings
of 29th IEEE EMBS, pp. 1663-1666.
Shumway-Cook, A, Ciol, M. A., Hoffman, J., Dudgeon,
B., Yorkston, K., Chan, L., 2009, “Falls in the
Medicare population: incidence, associated factors,
and impact on health care,” Physical Therapy
Association; vol. 89, no. 4, pp. 324-32.
STMicroelectronics, 2008, “LIS3LV02DL Data Sheet
Rev.2,” January 2008, <http://www.st.com>.
A MULTI-SENSOR SYSTEM FOR FALL DETECTION IN AMBIENT ASSISTED LIVING CONTEXTS
219