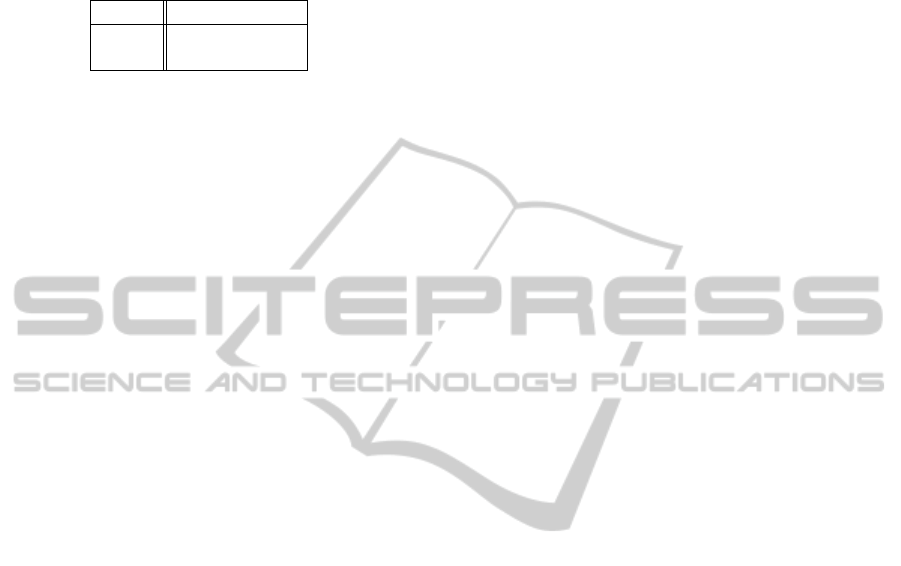
Table 3: Quantitative comparisons on the dimetrodon
sequence with a Lucas-Kanade term embed in a multi-
resolution scheme with successive convolutions without any
assimilation (CONV) and the proposed multi-resolution us-
ing variational assimilation (AMR).
CONV AMR
AAE 7.95
o
6.50
o
RMSE 0.98 0.62
a usual one using similar observation terms. This is a
promising behavior.
5.4 Key Message
Various situations have been tested with these three
experiments: the first one exhibits a flow with many
interactions between scales, the second one is submit-
ted to the aperture problem whereas the third one is
composed of a more complex velocity field. From
the associated qualitative and quantitative values, it is
very interesting to point out that for a similar tech-
nique (i.e. the Lucas & Kanade estimaion), the new
multi-resolution approach is more competitive in all
applications. This is the key message of these experi-
ments.
6 CONCLUSIONS
In this paper, we have introduced an original mean
to perform multi-resolution strategies commonly used
in computer vision. These techniques, used to man-
age efficiently some simplifications (as linearization),
generally suffer from one drawback: their inability to
correct errors of coarser resolutions. Errors are indeed
most of the time propagated along the scales. In this
study, we have exploited a framework issued from op-
timal control theory and in particular variational data
assimilation to solve this issue. The general idea of
variationaldata assimilation techniques consist in per-
forming a set of forward/backward integrations of a
dynamical system to estimate a system state. Applied
to a scale-space equations, we have derived a consis-
tent mathematical framework to perform any multi-
resolution scheme in a set of forward/backward inte-
grations that in practice correspond to a set of down-
scaling/upscaling estimations.
We have validated the idea on a simple Lucas-
Kanade motion estimation technique for three syn-
thetic pair of images corresponding to various situ-
ations. The experimental results reveal that for all
tested images, our multi-resolution approach outper-
forms classic ones, which is a very interesting and
promising conclusion. As future works, we will
use more advanced observation terms associated with
non-linear scale space dynamics able to preserve dis-
continuities.
REFERENCES
Alvarez, L., Weickert, J., and S´anchez, J. (2000). Reli-
able estimation of dense optical flow fields with large
displacements. International Journal of Computer Vi-
sion, 39(1):41–56.
Baatz, M. and Schaape, A. (2000). Multiresolution Seg-
mentation: an optimization approach for high qual-
ity multi-scale image segmentation. In Strobl, J., ed-
itor, Angewandte Geographische Informationsverar-
beitung XII. Beitr¨age zum AGIT-Symposium Salzburg
2000, Karlsruhe, Herbert Wichmann Verlag, pages
12–23.
Bajcsy, R. and Kovacic, S. (1989). Multiresolution elas-
tic matching. Computer Vision, Graphics, and Image
Processing, 46(1):1 – 21.
Baker, S. and Matthews, I. (2004). Lucas-Kanade 20 Years
On: A Unifying Framework. International Journal of
Computer Vision, 56(3):221–255.
Baker, S., Scharstein, D., Lewis, J., Roth, S., Black, M.,
and Szeliski, R. (2007). A Database and Evaluation
Methodology for Optical Flow. In Int. Conf. on Comp.
Vis., ICCV 2007.
Baker, S., Scharstein, D., Lewis, J. P., Roth, S., Black, M. J.,
and Szeliski, R. (2010). A Database and Evaluation
Methodology for Optical Flow. International Journal
of Computer Vision, 92(1):1–31.
Barron, J., Fleet, D., Beauchemin, S., and Burkitt, T.
(1994). Performance Of Optical Flow Techniques. In-
ternational Journal of Computer Vision, 12(1):43–77.
Bennett, A. (1992). Inverse Methods in Physical Oceanog-
raphy. Cambridge University Press.
Brox, T., Bruhn, A., Papenberg, N., and Weickert, J. (2004).
High accuracy optical flow estimation based on a the-
ory for warping. pages 25–36. Springer.
Burt, P. (1988). Smart sensing within a pyramid vision ma-
chine. Proceedings of the IEEE, 76(8):1006 – 1015.
Corpetti, T., H´eas, P., M´emin, E., and Papadakis, N. (2009).
Pressure image assimilation for atmospheric motion
estimation. Tellus Series A: Dynamic Meteorology
and Oceanography, 61(1):160–178.
Corpetti, T. and M´emin, E. (2012). Stochastic uncer-
tainty models for the luminance consistency assump-
tion. IEEE Trans. on Image Processing, to appear.
Corpetti, T., M´emin, E., and P´erez, P. (2002). Dense Esti-
mation of Fluid Flows. IEEE Transactions on Pattern
Analysis and Machine Intelligence, 24(3):365–380.
Delanay, A. and Bresler, Y. (1998). Globally convergent
edge-preserving regularized reconstruction: an appli-
cation to limited-angle tomography. IEEE Trans. Im-
age Processing, 7(2):204–221.
Eck, M., DeRose, T., Duchamp, T., Hoppe, H., Lounsbery,
M., and Stuetzle, W. (1995). Multiresolution analy-
sis of arbitrary meshes. In Proceedings of the 22nd
VISAPP 2012 - International Conference on Computer Vision Theory and Applications
142