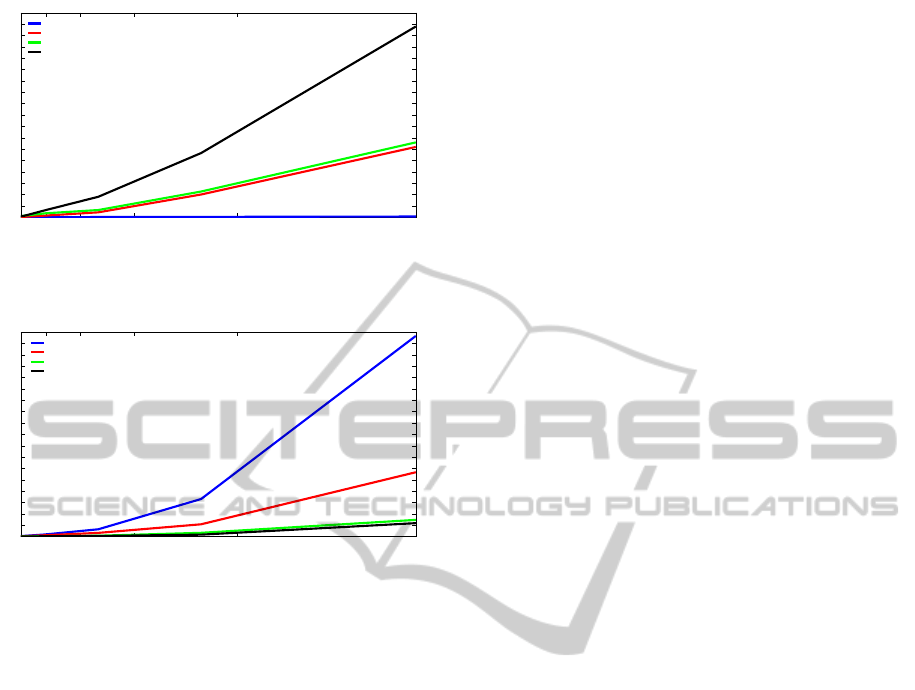
145200 187500 480000 1228800 2666000 5668704
0
5
10
15
20
25
30
35
40
45
50
55
60
65
70
75
80
85
90
Sobel Energy Map
Number of pixels
Computational time (min)
Non−Iterative
Iterative considering neighborhood and multiscale
Iterative considering neighborhood
Iterative
Figure 3: Execution time on a standard PC of the proposed
approaches vs. image size. Sobel has been chosen as energy
map.
145200 187500 480000 1228800 2666000 5668704
0
5
10
15
20
25
30
35
40
45
50
55
60
65
70
75
80
85
90
Non−Iterative Approach
Number of Pixels
Computational time (min)
Itti et al. Energy Map
Simplified Itti et al. Energy Map
Gaussian Energy Map
Sobel Energy Map
Figure 4: Execution time on a Nokia N900 of the non-
iterative approach vs. image size. Each line represents the
results obtained with different energy map: Itti et al. (blue),
simplified Itti et al. (red), Gaussian (green), Sobel (black).
ering both neighborhood and multiscale, and non-
iterative. These tests have been performed consider-
ing the Sobel energy map. All the approaches, ob-
viously, increase their computational time at increas-
ing of the image size. Although the non-iterative ap-
proach considerably outperforms the others, it shows
lower performance in terms of artifact generation as
pointed out in previous section. On the contrary,
the optimized iterative approaches (considering both
neighborhood and multiscale) sensitively reduce the
execution time with respect to the original iterative
approach without affecting the visual quality of the
resized image. From the analysis performed through
the quantitative tests on a standard PC, and consider-
ing the limitations in terms of CPU clock frequency
and memory storage of the mobile devices, only the
non-iterative approach has been considered for final
implementation on the Nokia N900 platform (Adams
et al., 2010; Battiato et al., 2012). Figure 4 shows the
execution time of the non-iterative algorithm running
on a Nokia N900 at increasing of the image size. Sev-
eral energy maps have been compared. Considering
all the optimizations both in terms of energy map (So-
bel) and algorithm (non-iterative), the approach takes
about 5 seconds on a Nokia N900 to resize an image
of about 5 megapixels.
4 CONCLUSIONS
In this paper we have proposed several strategies to
allow content-aware image resizing on mobile de-
vices. All the involved steps have been considered
and optimized in order to find a good trade-off be-
tween visual quality and computational complexity.
Specifically, several energy maps (Itti et al., simpli-
fied Itti et al., Gaussian, Sobel) and different versions
of the line removal algorithm (iterative, iterative with
neighborhood, iterative with neighborhood and mul-
tiscale, non-iterative) have been exploited. Moreover,
to properly compare the proposed solutions in terms
of visual quality and computational load, several tests
on a standard PC and on a Nokia N900 mobile phone
have been also performed. Future works will be de-
voted to find additional smart strategies useful to cut
down the computational time in order to employ the
iterative approaches on mobile devices.
REFERENCES
Adams, A., Talvala, E.-V., Park, S. H., Jacobs, D. E., Ajdin,
B., Gelfand, N., Dolson, J., Vaquero, D., Baek, J.,
Tico, M., Lensch, H. P. A., Matusik, W., Pulli, K.,
Horowitz, M., and Levoy, M. (2010). The franken-
camera: an experimental platform for computational
photography. ACM Trans. Graph., 29:29:1–29:12.
Avidan, S. and Shamir, A. (2007). Seam carving for
content-aware image resizing. ACM Transaction on
Graphics, 26(3).
Battiato, S., Farinella, G. M., Messina, E., Puglisi, G., Rav
`
ı,
D., Capra, A., and Tomaselli, V. (2012). On the per-
formances of computer vision algorithms on mobile
platforms. In SPIE Electronic Imaging - Digital Pho-
tography VIII.
Cho, T., Butman, M., Avidan, S., and Freeman, W. (2008).
The patch transform and its applications to image edit-
ing. In IEEE International Conference on Computer
Vision and Pattern Recognition (CVPR).
Gallea, R., Ardizzone, E., and Pirrone, R. (2010). Real-time
content-aware resizing using reduced linear model. In
IEEE International Conference on Image Processing.
Itti, L., Koch, C., and Niebur, E. (1998). A model of
saliency-based visual attention for rapid scene anal-
ysis. IEEE Transactions on Pattern Analysis and Ma-
chine Intelligence, 20(11).
Rubinstein, M., Shamir, A., and Avidan, S. (2009). Multi-
operator media retargeting. ACM Transaction on
Graphics, 28(3).
VISAPP 2012 - International Conference on Computer Vision Theory and Applications
90