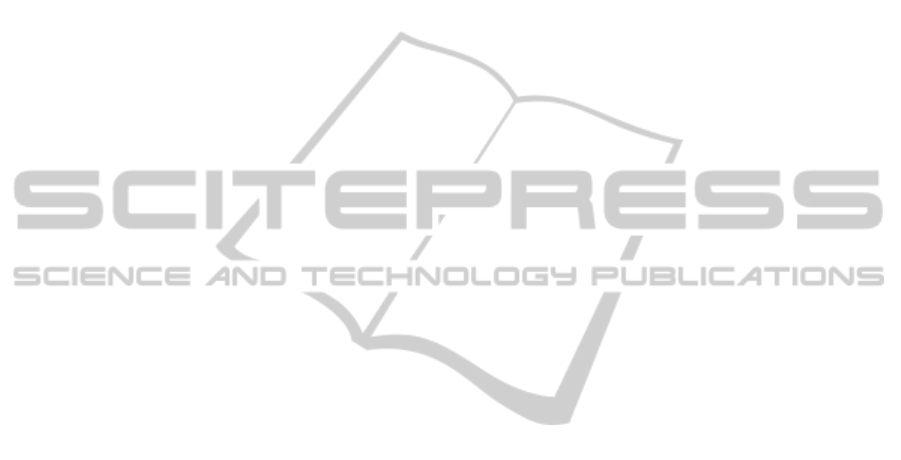
Environment, 22, 159–172.
Kolbe, T. H., Gerhard, G. and Plümer, L., 2005.
CityGML—Interoperable access to 3D city models.
In: International Symposium on Geoinformation for
Disaster Management GI4DM 2005, Delft,
Netherlands, Lecture Notes in Computer Science,
March, 2005.
Lee, D. D., and Seung. H. S., 2001. Algorithms for
nonnegative matrix factorization. In T. G. Dietterich
and V. Tresp, editors, Advances in Neural Information
Processing Systems, volume 13. Proceedings of the
2000 Conference: 556562, The MIT Press.
Lin, C. J., 2007. Projected gradient methods for non-
negative matrix factorization. Neural Computation,
19, 2756-2779.
Lindeberg, T., 2004. Feature detection with automatic
scale selection. International Journal of Computer
Vision, 30, 79-116.
Masaharu, H. and Ohtsubo, K., 2002. A filtering method
of airborne laser scanner data for complex terrain. The
International Archives of Photogrammetry, Remote
Sensing, and Spatial Information Sciences, 15 (3B),
165-169.
Nichol, J. E., 1994. A GIS-based approach to
microclimate monitoring in Singapore’s high-rise
housing estates. Photogrammetric Engineering and
Remote Sensing, 60, 1225–1232.
Nichol, J. E., 1996. High-resolution surface temperature
patterns related to urban morphology in a tropical city:
a satellite-based study. Journal of Applied
Meteorology, 35, 135–146.
Pudil P., Novovicova J., Kittler J., 1994. Floating search
methods in feature selection. Pattern Recognition
Letters, 15, 1119-1125.
Robila, S. A., and Maciak, L. G., 2006. A parallel
unmixing algorithm for hyperspectral images.
Technical report, Center for Imaging and Optics,
Montclair State University.
Roessner, S., Segl, K., Heiden, U. and Kaufmann, H.,
2001. Automated differentiation of urban surfaces
based on airborne hyperspectral imagery. IEEE
Transactions on Geoscience and Remote Sensing, 39
(7), 1525-1532.
Roth, M., Oke, T. R., and Emery, W. J., 1989. Satellite-
derived urban heat islands from three coastal cities and
the utilization of such data in urban climatology. Inter-
national Journal of Remote Sensing, 10, 1699–1720.
Rottensteiner, F., Trinder, J., Clode, S., Kubic, K., 2003.
Building detection using LIDAR data and
multispectral images. In: Proceedings of DICTA,
Sydney, Australia, 673-682.
Rubila S. A., and Maciak L. G., 2009. Considerations on
Parallelizing Nonnegative Matrix Factorization for
Hyperspectral Data Unmiging. Geoscience and
Remote Sensing Letters, IEEE, 6(1), 57-61.
Sacks, J., Welch, W. J., Mitchell, T. J., and Wynn, H. P.,
1989. Design and analysis of computer experiments.
Statistical Science, 4(4), 409–435.
Salisbury, J. W., Hapke B., and Eastes, J. W., 1987.
Usefulness of Weak Bands in Midinfrared Remote
Sensing of Particulate Planetary Surfaces. Journal of
Geophysical Research, 92, pp. 702-710.
Segl, K., Roessner, S., Heiden, U., Kaufman. H., 2003.
Fusion of spectral and shape features for identification
of urban surface cover types using reflective and
thermal hyperspectral data. ISPRS Journal of
Photogrammetry and Remote Sensing, 58, 99-112.
Scholkopf, B., Platt, J. C., Shawe-Taylor, J., Smola, A. J.,
and Williamson, R. C., 2001. Estimating the support
of a high-dimensional distribution. Technical report,
Microsoft Research, MSR-TR, 87-99.
Steele, J. M., 2002. Minimum spanning trees for graphs
with random edge lengths, Mathematics and Computer
Science, 2, 223-245.
Tao, V., 2001. Database-guided automatic inspection of
vertically structured transportation objects from mo-
bile mapping image sequences. In: ISPRS Press, 1401-
1409.UserGuide eCognition, 2003. Website: www.
Definiens_imaging.com.
Vapnik, V., 1998. Statistical learning theory. New York:
Wiley.
Velaga, N. R., Quddus, M. A. and Bristow, A. L., 2009.
Developing an enhanced weight-based topological
map-matching algorithm for intelligent transport sys-
tems. Transportation Research Part C: Emerging Tech-
nologies, 17, 672-683.
Villa, A., Chanussot, J., Benediktsson, J. A., and Jutten,
C., 2011. Spectral Unmixing for the Classification of
Hyperspectral Images at a Finer Spatial Resolution.
IEEE Selected Topics in Signal Processing, 5(3), 521
– 533.
Vukovich, F. M., 1983. An analysis of the ground tem-
perature and reflectivity pattern about St. Louis,
Missouri, using HCMM satellite data. Journal of
Climate and Applied Meteorology, 22, 560–571.
Wang, J., and Shan, J., 2009. Segmentation of LiDAR
point clouds for building extraction. In: ASPRS 2009
Annual Conference, Baltimore, MD.
Whitney A. W., 1971. A direct method of nonparametric
measurement selection. IEEE Transactions on
Computers, 20, 1100-1103.
Yang, F., and Jiang, T., 2003. Pixon-based image
segmentation with Markov random fields, IEEE Trans
Image Process. 12(12):1552-9.
Young, S. J., Johnson, R. B., and Hackwell, J. A., 2002.
An in-scene method for atmospheric compensation of
thermal hyperspectral data. Journal of Geophysical
Research, 107, 20-28.
Zhou, G., 2004. Urban 3D GIS from LiDAR and digital
aerial images. Computers and Geosciences, 30,
345-
353.
FUSION OF OPTICAL AND THERMAL IMAGERY AND LIDAR DATA FOR APPLICATION TO 3-D URBAN
ENVIRONMENT - Pattern Recognition Applications in Remotely Sensed Hyperspectral Image Analysis
405