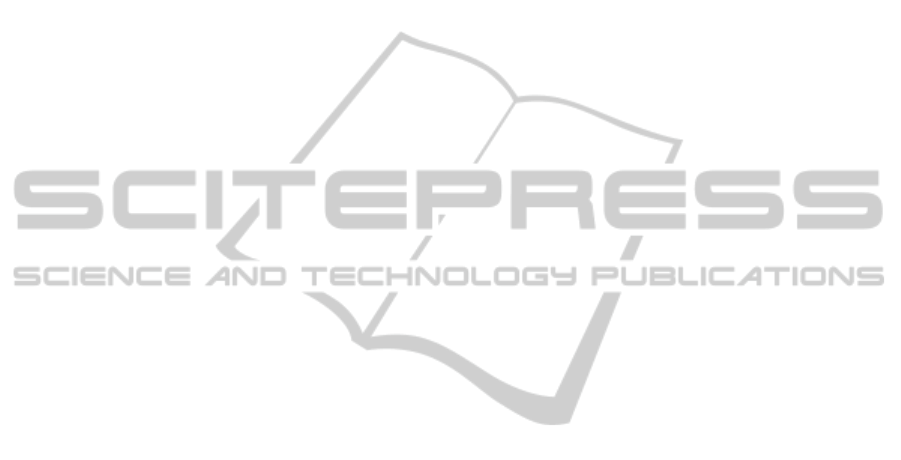
age based intuitive cognitive process or task-oriented
attention element.
C: A Center-Surround map was generated by drop-
ping an Gaussian kernel at the center of an im-
age. The half-height width for the Gaussian is
determined according to the area over which fix-
ation can be said to exist. The biological back-
ground for the algorithms is based on the central
fixation bias noted by previous studies (Buswell,
1935; Tatler, 2007).
D: An Attention Distribution Map combines the
saliency map from Itti’s model and a task rele-
vance map. For Itti’s model, the three conspicu-
ity maps are normalized and summed together at
an equal ratio, to become a saliency map (S).For
the task relevance map, under the context of the
images being graphs, the quantitative data it rep-
resents is used as task-relevance. For each section
labeled differently, the comparativeamount repre-
senting each different region is used in a manner
so that the larger the data, the higher the conspic-
uous value, the larger the cADR assigned to the
region.
3 EXPERIMENTAL METHOD
During the experiment, eye movements were mea-
sured using an eye tracker (nac: EMR-NL, 640x460
pixel resolution, 60Hz). Images of graphs were dis-
played on a computer screen for three seconds each,
followed by a one-second interval during which sub-
jects fixate on a central cross. The coordinated po-
sitions on the images where subjects were looking
was recorded for later analysis. The subjects were
seated in front of a screen with their head secured to a
chin-rest structure. The viewing distance was approx-
imately 65 cm; the stimulus size was about 20 cm x
25 cm. In this paper, fixation is defined as a stable eye
position with a velocity below the threshold of 20 de-
grees per second (Robert et al., 2003), and a scan path
as the spatial arrangement of a sequence of fixations.
45 different images of graphs were utilized. There
were five types of graphs with different design: two
types of bar graphs and three types of pie charts. For
each type of graph’s design, there were 9 graphs rep-
resenting 9 different sets of data. These 9 sets each
have three components. Six subjects (3 male, 3 fe-
male) were used. Each subject repeated this experi-
ments for 3 times.
4 RESULTS
4.1 Selection of IPAs for Each
Subsequent Numbered Fixation
The best IPAs for each subsequent numbered fixa-
tion in the experimental situation are determined from
the strength of the correlational relationship between
eADR (experimental Attention Distribution Ratio)
and cADR (computed Attention Distribution Ratio)
from the 1st fixation to the 7th fixation. The cADR in
the regions labeled from 1 to 3 by the method in sec-
tion 5.1.1 are calculated for all images (45 images x
3 IPAs). The experimental Attention Distribution Ra-
tio (eADR) of each subsequent numbered fixation on
each image in the three second experiment was calcu-
lated. Then, the correlation coefficients between the
computational data (cADR) and the experimental data
(eADR) are computed from the 1st fixation to the 7th
fixation.
Figure 3 plots the dynamic changes in correla-
tional value (prediction accuracy) of IPAs from 1st
fixation to the 7th fixation. The figure 3 confirms the
expectation that each IPA has its own peak at a differ-
ent subsequent numbered fixations. This data implies
that the distribution of the scan path can be modeled
by C from the 1st to the 2nd fixation, and by D from
the 3rd to the 5th. In this way, the dynamic changes
in the eADR over fixation number are suitably incor-
porated in the model by the shift of the static cADR
computed by each IPA. The effectiveness of this is
apparent when correlation values are compared with
existing prediction model (S), which defines an abso-
lute single path by a single cADR.
The cADR computed from the above-mentioned
model changes its value over the sequence of fixations
by shifting the IPA. Thus, the model for ADR predic-
tion can be defined as follows: IPA C calculate P(k, s)
for k = 1, 2; IPA D calculate P(k, s) for k = 3, 4. The
calculation of the scan path probability is by equation
1.
4.2 Accuracy of the Model
Table 1 compares the probability of the computation-
ally predicted scan paths (see equation 1) and the ex-
perimental probability when subjects are viewing a
particular image. There are 81 (3
4
) possible states
in total for the subsequent numbered fixations from 1
to 4, The table shows 4 types of scan paths with the
highest computational probabilities. This result is en-
couraging because it suggests the validity of our pro-
posed method of predicting possibility of scan paths.
The predicted probability of each scan path and
COMPUTATIONAL MODEL FOR PROBABILITY PREDICTION OF SCAN PATHS IN STATIC SCENES
429