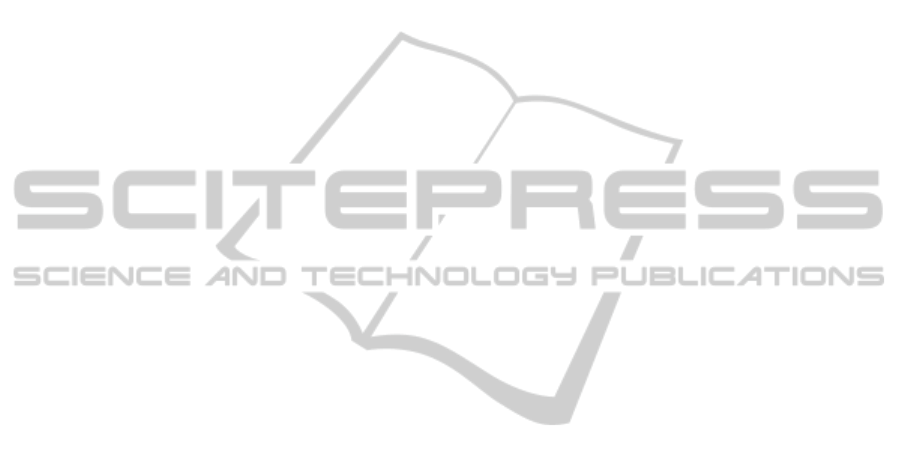
tively. We formed different age class schemes for
different datasets by using a heuristic approach. Our
future work will concentrate on generating age class
scheme automatically according to the characteristics
of the dataset that used in the age estimation experi-
ments.
ACKNOWLEDGEMENTS
The authors would like to thank Dr. K. Ricanek
of UNCW for providing the MORPH Face Aging
Database (Ricanek Jr. and Tesafaye, 2006) and they
would also like to thank the FG-NET consortium
for providing the FG-NET Aging Database (FGNET,
2010).
REFERENCES
Ahonen, T., Hadid, A., and Pietikainen, M. (2004). Face
Recognition with Local Binary Patterns. In Computer
Vision - ECCV 2004, pages 469–481.
Bhuiyan, A. and Liu, C. H. (2007). On face recognition
using gabor filters.
Burt, D. M. and Perrett, D. I. (1995). Perception of age in
adult caucasian male faces: Computer graphic manip-
ulation of shape and colour information. Proceedings
of the Royal Society of London. Series B: Biological
Sciences, 259(1355):137–143.
DeCarlo, D., Metaxas, D., and Stone, M. (1998). An an-
thropometric face model using variational techniques.
In Proceedings of the 25th annual conference on
Computer graphics and interactive techniques, SIG-
GRAPH ’98, pages 67–74, New York, NY, USA.
ACM.
Ekenel, H., Fischer, M., Tekeli, E., Stiefelhagen, R., and
Ercil, A. (2008). Local binary pattern domain local
appearance face recognition. In Signal Processing,
Communication and Applications Conference, 2008.
SIU 2008. IEEE 16th, pages 1–4.
FGNET (2010). The FG-NET Aging Database [On-
line]. Available: http://www.fgnet.rsunit.com/, 2010.
http://www.fgnet.rsunit.com.
Freund, Y. and Schapire, R. E. (1996). Experiments with
a New Boosting Algorithm. In In Proceedings of
the Thirteenth International Conference on Machine
Learning, pages 148–156.
Fu, Y., Guo, G., and Huang, T. (2010). Age synthesis and
estimation via faces: A survey. IEEE Transactions on
Pattern Analysis and Machine Intelligence, 32.
Gao, F. and Ai, H. (2009). Face age classification on
consumer images with gabor feature and fuzzy lda
method. In Proceedings of the Third International
Conference on Advances in Biometrics, ICB ’09,
pages 132–141, Berlin, Heidelberg. Springer-Verlag.
Geng, X., hua Zhou, Z., and Smith-Miles, K. (2007). Au-
tomatic age estimation based on facial aging patterns.
IEEE Transactions on Pattern Analysis and Machine
Intelligence, 29:2234–2240.
Guo, G., Fu, Y., Dyer, C., and Huang, T. (2008). Image-
based human age estimation by manifold learning and
locally adjusted robust regression. Image Processing,
IEEE Transactions on, 17(7):1178–1188.
Guo, G., Mu, G., Fu, Y., and Huang, T. (2009). Human age
estimation using bio-inspired features. In Computer
Vision and Pattern Recognition, 2009. CVPR 2009.
IEEE Conference on, pages 112–119.
Kwon, Y. H. and Lobo, N. D. V. (1999). Age classification
from facial images. In In Proc. IEEE Conf. Computer
Vision and Pattern Recognition, pages 762–767.
Lanitis, A., Draganova, C., and Christodoulou, C. (2004).
Comparing different classifiers for automatic age es-
timation. IEEE Trans Syst Man Cybern B Cybern,
34(1):621–8.
Marcel, S., Rodriguez, Y., and Heusch, G. (2007). On the
recent use of local binary patterns for face authen-
tication. International Journal on Image and Video
Processing Special Issue on Facial Image Processing.
IDIAP-RR 06-34, accepted for publication but with-
drawn because of author charges.
Ramanathan, N. and Chellappa, R. (2006). Modeling age
progression in young faces. In Computer Vision and
Pattern Recognition, 2006 IEEE Computer Society
Conference on, volume 1, pages 387–394.
Ramanathan, N. and Chellappa, R. (2008). Modeling shape
and textural variations in aging faces. In Automatic
Face Gesture Recognition, 2008. FG ’08. 8th IEEE
International Conference on, pages 1–8.
Ricanek Jr., K. and Tesafaye, T. (2006). Morph: A longitu-
dinal image database of normal adult age-progression.
In Proceedings of the 7th International Conference on
Automatic Face and Gesture Recognition, FGR ’06,
pages 341–345, Washington, DC, USA. IEEE Com-
puter Society.
Shan, S., Gao, W., Chang, Y., Cao, B., and Yang, P.
(2004). Review the Strength of Gabor Features for
Face Recognition from the Angle of Its Robustness
to Mis-Alignment. Pattern Recognition, International
Conference on, 1:338–341.
Yan, S., Zhou, X., Liu, M., Hasegawa-Johnson, M., and
Huang, T. (2008). Regression from patch-kernel.
In Computer Vision and Pattern Recognition, 2008.
CVPR 2008. IEEE Conference on, pages 1–8.
Yang, Z. G. and Ai, H. Z. (2007). Demographic Classifica-
tion with Local Binary Patterns. Biometric Authenti-
cation, pages 464–473.
Zhang, W., Shan, S., Gao, W., Chen, X., and Zhang, H.
(2005). Local gabor binary pattern histogram se-
quence (lgbphs): a novel non-statistical model for face
representation and recognition. In Computer Vision,
2005. ICCV 2005. Tenth IEEE International Confer-
ence on, volume 1, pages 786–791 Vol. 1.
VISAPP 2012 - International Conference on Computer Vision Theory and Applications
538